Rethinking maximum-margin softmax for adversarial robustness
COMPUTERS & SECURITY(2022)
摘要
Learning discriminative features with adversarial behaviors can be extremely challenging to build a robust learning model. This is partly evidenced by the difficulties in training robust maximum-margin models (e.g., ArcFace and CosFace) that cannot discriminate decision boundaries between perturbed samples perfectly. One potential approach is to design a loss function that achieves robust generalization by learning high-density features in the latent space to discriminate between adversarial and legitimate samples effectively. Therefore, we propose an Ensemble Maximum-Margin Softmax (EMMS) method to construct a robust generalization that yields reliable models. Specifically, EMMS is designed to address the limitation in maximum-margin methods and induce high-density discriminative features for clean and adversarial settings. The empirical experiments using the CIFAR and SVHN datasets show that EMMS is more robust in terms of accuracy and error rates than other peer techniques. The outcomes suggest that EMMS could improve the robustness of the model compared with ArcFace and CosFace under various types of attacks. (c) 2022 Elsevier Ltd. All rights reserved.
更多查看译文
关键词
Adversarial attacks,Adversarial defenses,Max -Margin learning,Image classification,Deep learning
AI 理解论文
溯源树
样例
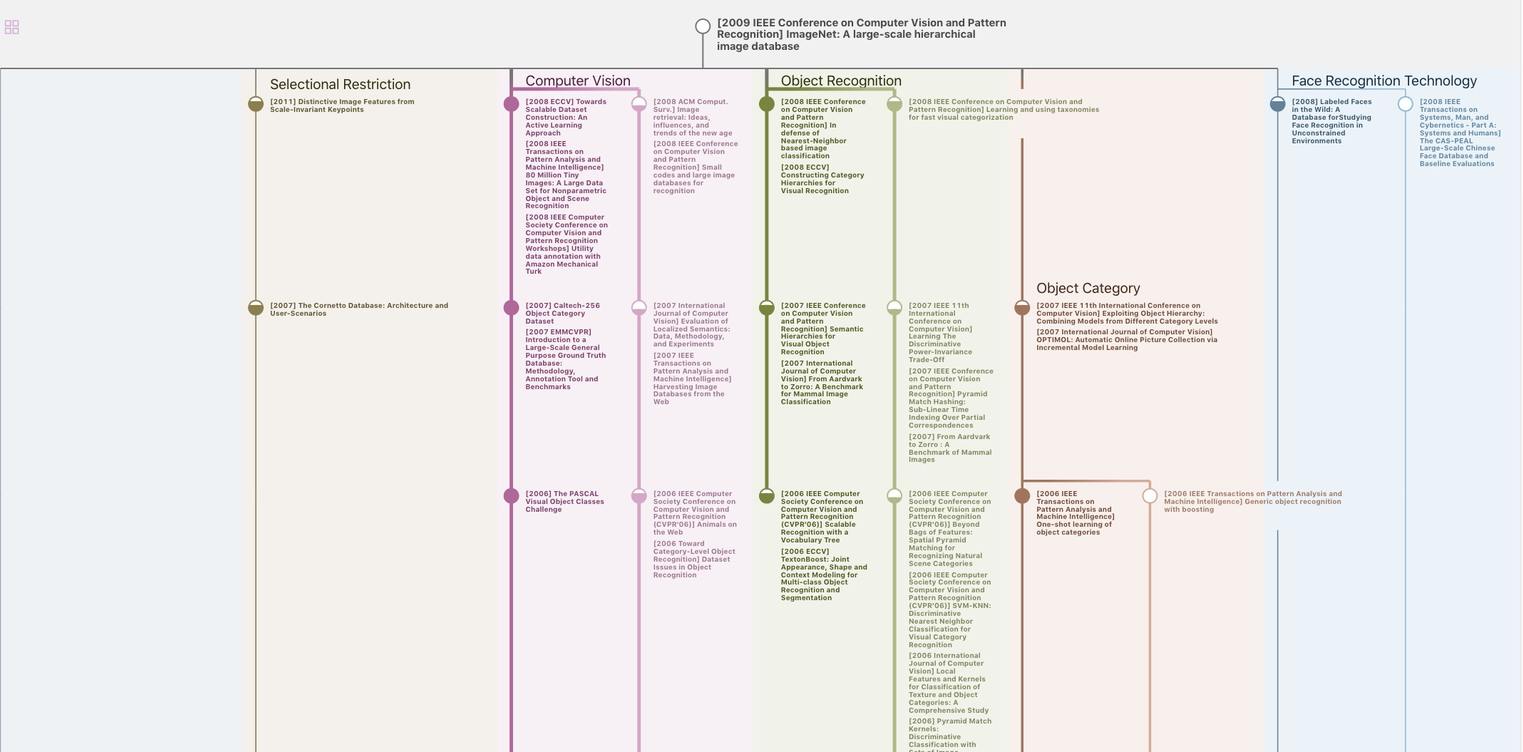
生成溯源树,研究论文发展脉络
Chat Paper
正在生成论文摘要