EMRA-Net: A Pixel-Wise Network Fusing Local and Global Features for Tiny and Low-Contrast Surface Defect Detection
IEEE TRANSACTIONS ON INSTRUMENTATION AND MEASUREMENT(2022)
摘要
The problem of tiny and low-contrast surface defect detection is a nontrivial one. To solve the problems, this article proposes an edge and multi-scale reverse attention network (EMRA-Net), of which includes feature extraction and feature fusion. In the process feature extraction, the global dynamic convolution features, global dynamic multi-scale fusion (MSF) feature, and local pyramid edge feature are obtained through the pre-training backbone Resnet 34, the fresh MSF module, and the innovative pyramid edge module, respectively. In the process of feature fusion, these features are blended by a new self-learning scale module and a novel spatial channel domain reverse attention (SCRA) module step by step. The experimental results of five widely used datasets show that the EMRA-Net outperforms the existing methods. In addition, the mean intersection of union (mIoU)on the printed circuit board (PCB) industry dataset reaches 95.31 & x0025;. Moreover, the results of EMRA-Net indicated that the local edge feature can improve the performance of the defect detection network. The EMRA-Net has great potential in the application in the detection of tiny and low-contrast defects.
更多查看译文
关键词
Feature extraction,Image edge detection,Convolution,Image segmentation,Deep learning,Object segmentation,Data mining,Global dynamic features,local pyramid edge module,multi-scale fusion (MSF),reverse attention,tiny and low-contrast defects detection
AI 理解论文
溯源树
样例
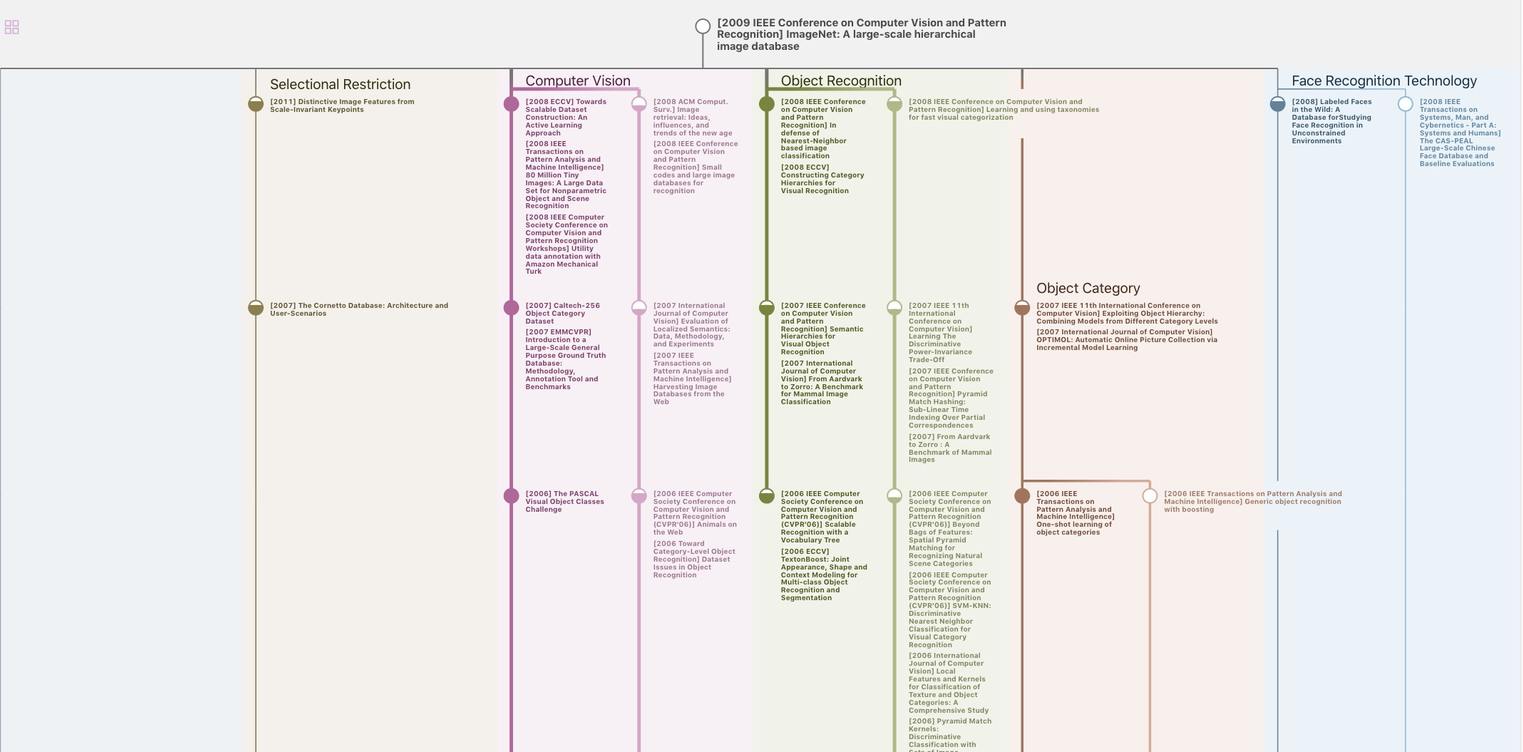
生成溯源树,研究论文发展脉络
Chat Paper
正在生成论文摘要