FeatInter: Exploring fine-grained object features for video-text retrieval
Neurocomputing(2022)
摘要
In this paper, we target the challenging task of video-text retrieval. The common way for this task is to learn a text-video joint embedding space by cross-modal representation learning, and compute the cross-modality similarity in the joint space. As videos typically contain rich information, how to represent videos in a joint embedding space is crucial for video-text retrieval. The majority of works typically depend on pre-extracted frame-level features or clip-level features for video representation, which may cause fine-grained object information in videos to be ignored. To alleviate it, we explicitly introduce more fine-grained object-level features to enrich video representation. In order to exploit the potential of the object-level features, we propose a new model named FeatInter, which jointly considers the visual and semantic features of objects. Besides, a visual-semantic interaction and a cross-feature interaction are proposed to mutually enhance object features and frame features. Extensive experiments on two challenging video datasets, i.e., MSR-VTT and TGIF, demonstrate the effectiveness of our proposed model. Moreover, our model achieves a new state-of-the-art on TGIF. While the state-of-the-art methods use seven video features on MSR-VTT, our model with just three features obtains comparable performance.
更多查看译文
关键词
Cross-modal retrieval,Video-text retrieval,Feature interaction,Visual semantic interaction,Fine-grained object feature
AI 理解论文
溯源树
样例
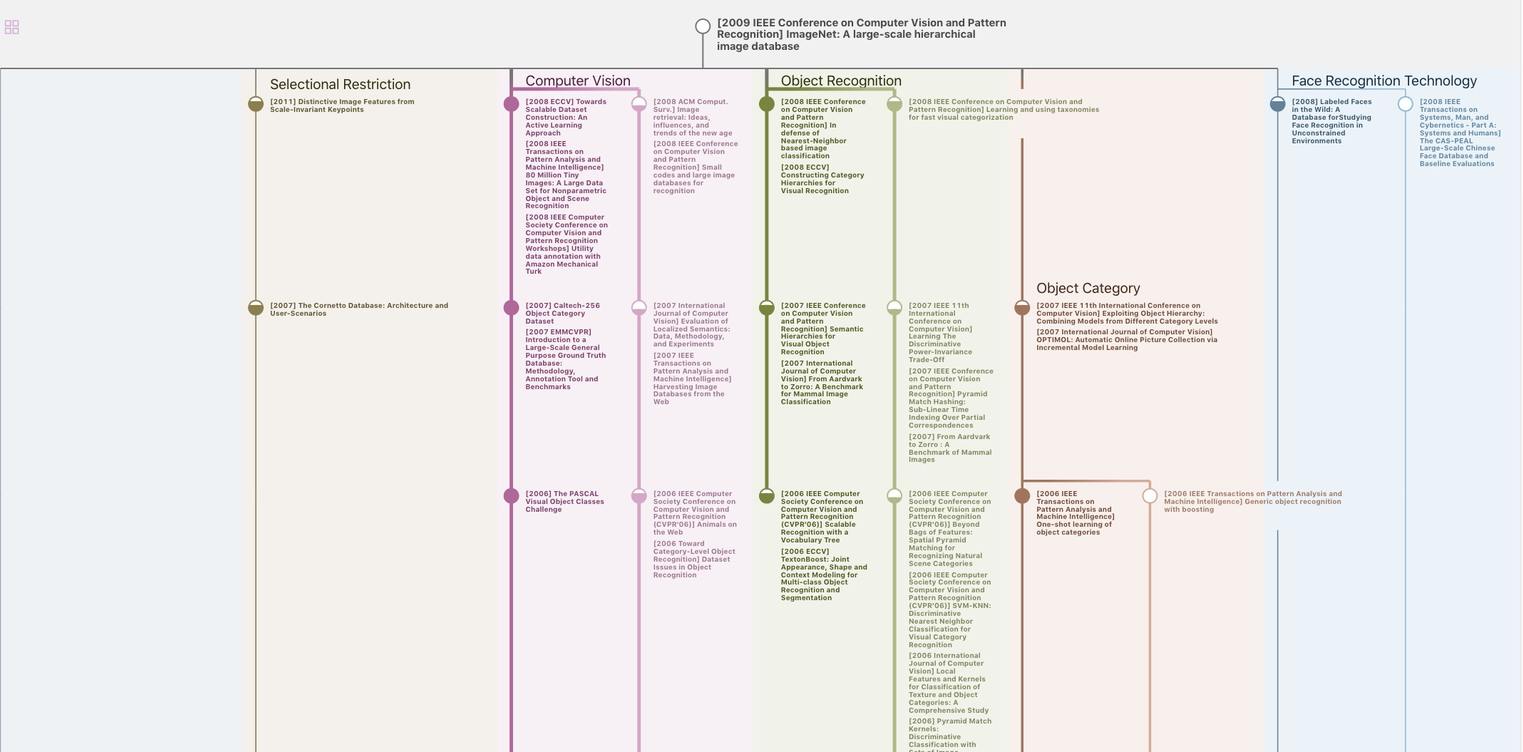
生成溯源树,研究论文发展脉络
Chat Paper
正在生成论文摘要