Modeling context appearance changes for person re-identification via IPES-GCN
Neurocomputing(2022)
摘要
Most of the previous person re-identification works focus on learning discriminative features for individuals, and retrieve a query person only based on pair-wise individual feature similarities, ignoring context relationships among gallery images. Consequently, it is hard to re-identify a query person when large appearance changes occur. To address this problem, we propose the IPES-GCN model to exploit context relationships across gallery images. In the IPES-GCN, we first construct an Individual Pivot Expansion Subgraph (IPES) to enrich the context representation of each individual. By taking high-order nearest neighbors of each individual into account, we can model more positive appearance changes into the representation. Then GCN is employed to explicitly model various appearance changes contained in the IPES into a graph embedding for each enriched individual. Finally, the graph embeddings are utilized to re-rank the gallery images. Experiments on the Market1501, DukeMTMC and MSMT17 datasets show that the proposed method has strong generalization and can yield favorable results to the state-of-the-art methods.
更多查看译文
关键词
High-order nearest neighbors,GCN,Re-ranking,Person Re-identification
AI 理解论文
溯源树
样例
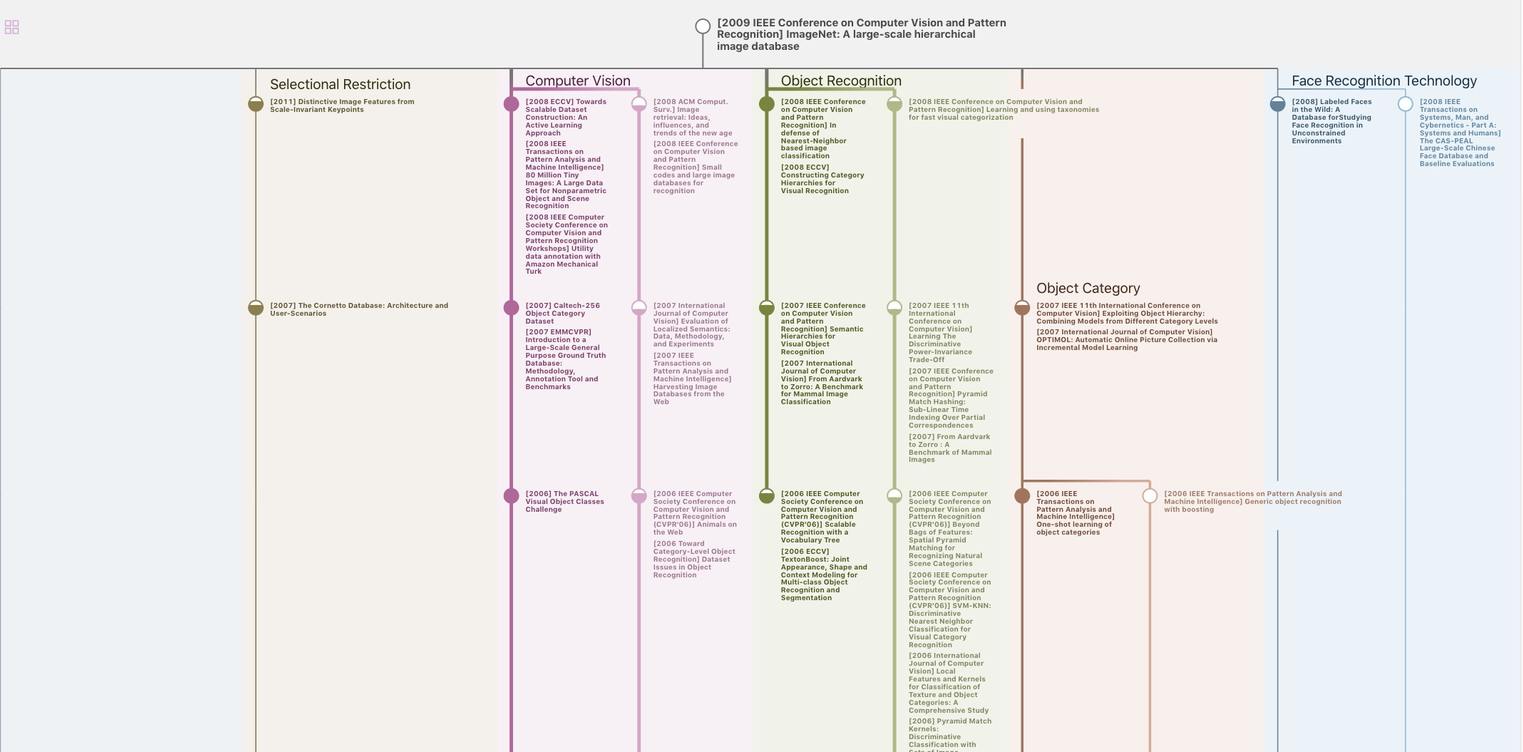
生成溯源树,研究论文发展脉络
Chat Paper
正在生成论文摘要