Extracting built-up areas from spectro-textural information using machine learning
Soft Computing(2022)
摘要
Extraction of built-up area (BUA) is essential for proper city planning and management. It enables the concerned authorities to formulate better city development policies and manage emergent disasters. However, the traditionally used optical data present spectral confusion where BUAs are mixed with other features adding to management complexities. Therefore, an advanced automated method is required to extract the spectral and textural features from satellite data for the pattern recognition of BUA. Landsat-8 Operational Land Imager (OLI) has been used in the current study to identify the pattern and extract BUA of Gujranwala, Pakistan. First, eight textural features based on the gray-level co-occurrence matrix (GLCM) are selected and combined with multispectral data. Then, feature selection methods are applied to select optimal features used to train the proposed support vector machine (SVM) classifier. Finally, the results from SVM classifiers are compared with k-nearest neighbor (k-NN) and backpropagation neural network (BP-NN) to highlight any improvements in results. The comparisons show that the proposed approach increases the overall accuracy of linear-SVM by 8.41%, radial basis function SVM by 8.3%, BP-NN by 7.63%, and k-NN by 6.6%. This can help city managers and planners to extract critical BUA information in otherwise unplanned and rapidly expanding cities to move toward smart and sustainable cities.
更多查看译文
关键词
Built-up areas, Support vector machine, Spectro-textural information, Pattern recognition, City planning
AI 理解论文
溯源树
样例
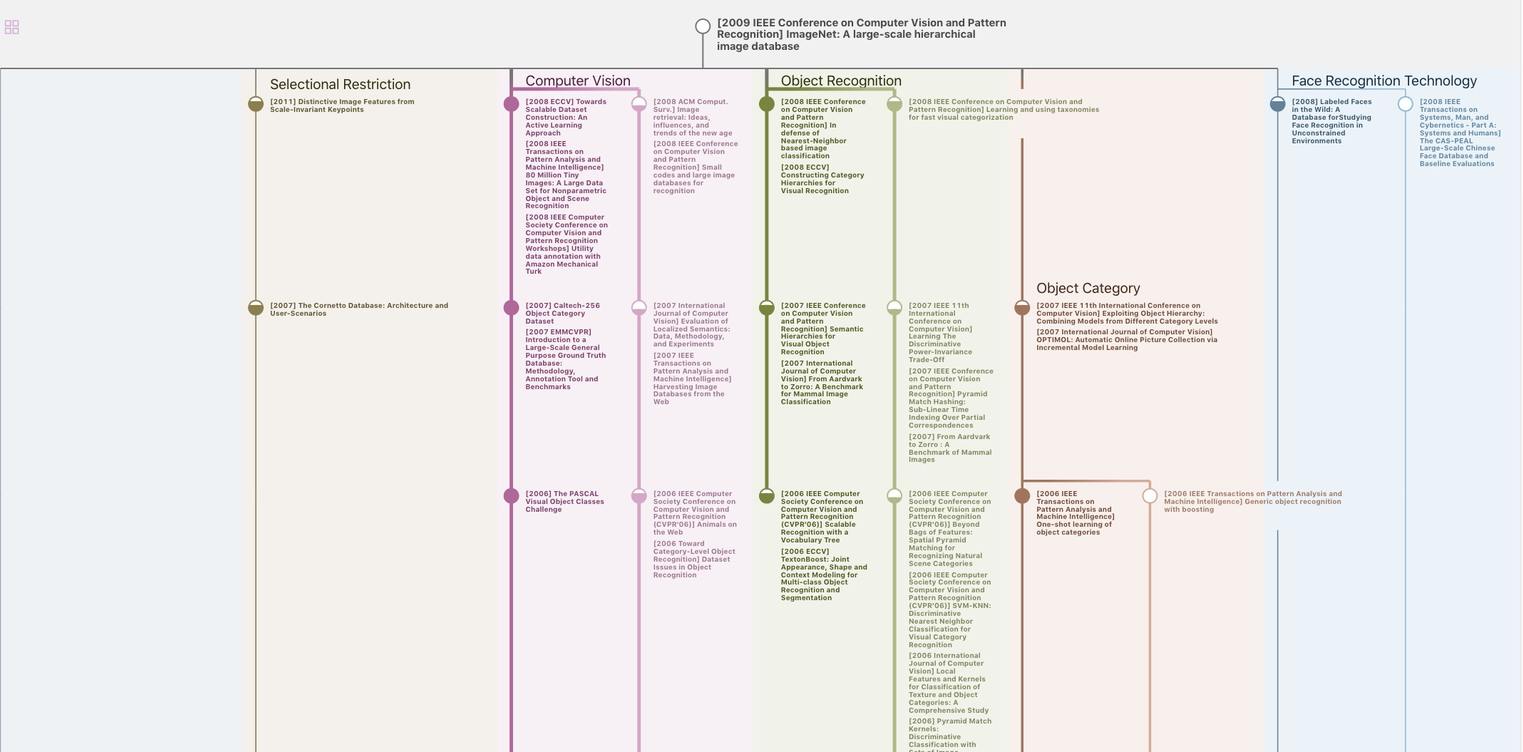
生成溯源树,研究论文发展脉络
Chat Paper
正在生成论文摘要