Spatial Consistency Loss for Training Multi-Label Classifiers from Single-Label Annotations
WACV(2023)
摘要
Multi-label image classification is more applicable "in the wild" than single-label classification, as natural images usually contain multiple objects. However, exhaustively annotating images with every object of interest is costly and time-consuming. We train multi-label classifiers from datasets where each image is annotated with a single positive label only. As the presence of all other classes is unknown, we propose an Expected Negative loss that builds a set of expected negative labels in addition to the annotated positives. This set is determined based on prediction consistency, by averaging predictions over consecutive training epochs to build robust targets. Moreover, the 'crop' data augmentation leads to additional label noise by cropping out the single annotated object. Our novel spatial consistency loss improves supervision and ensures consistency of the spatial feature maps by maintaining per-class running-average heatmaps for each training image. We use MS-COCO, Pascal VOC, NUS-WIDE and CUB-Birds datasets to demonstrate the gains of the Expected Negative loss in combination with consistency and spatial consistency losses. We also demonstrate improved multi-label classification mAP on ImageNet-1K using the ReaL multi-label validation set.
更多查看译文
关键词
Algorithms: Machine learning architectures,formulations,and algorithms (including transfer)
AI 理解论文
溯源树
样例
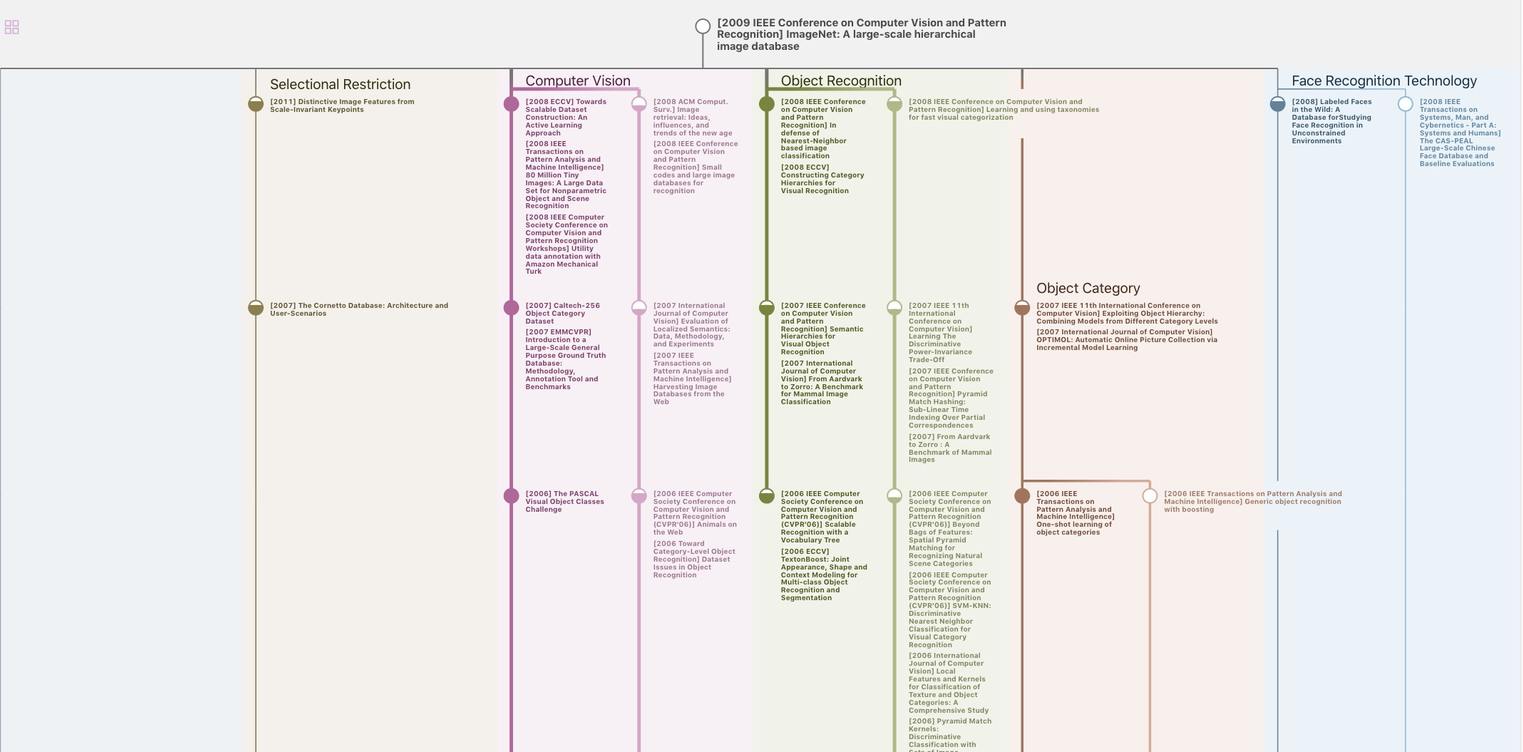
生成溯源树,研究论文发展脉络
Chat Paper
正在生成论文摘要