Deep Class-Incremental Learning From Decentralized Data.
IEEE transactions on neural networks and learning systems(2024)
摘要
In this article, we focus on a new and challenging decentralized machine learning paradigm in which there are continuous inflows of data to be addressed and the data are stored in multiple repositories. We initiate the study of data-decentralized class-incremental learning (DCIL) by making the following contributions. First, we formulate the DCIL problem and develop the experimental protocol. Second, we introduce a paradigm to create a basic decentralized counterpart of typical (centralized) CIL approaches, and as a result, establish a benchmark for the DCIL study. Third, we further propose a decentralized composite knowledge incremental distillation (DCID) framework to transfer knowledge from historical models and multiple local sites to the general model continually. DCID consists of three main components, namely, local CIL, collaborated knowledge distillation (KD) among local models, and aggregated KD from local models to the general one. We comprehensively investigate our DCID framework by using a different implementation of the three components. Extensive experimental results demonstrate the effectiveness of our DCID framework. The source code of the baseline methods and the proposed DCIL is available at https://github.com/Vision-Intelligence-and-Robots-Group/DCIL.
更多查看译文
关键词
Catastrophic forgetting,continuous learning,incremental learning (IL),knowledge distillation (KD)
AI 理解论文
溯源树
样例
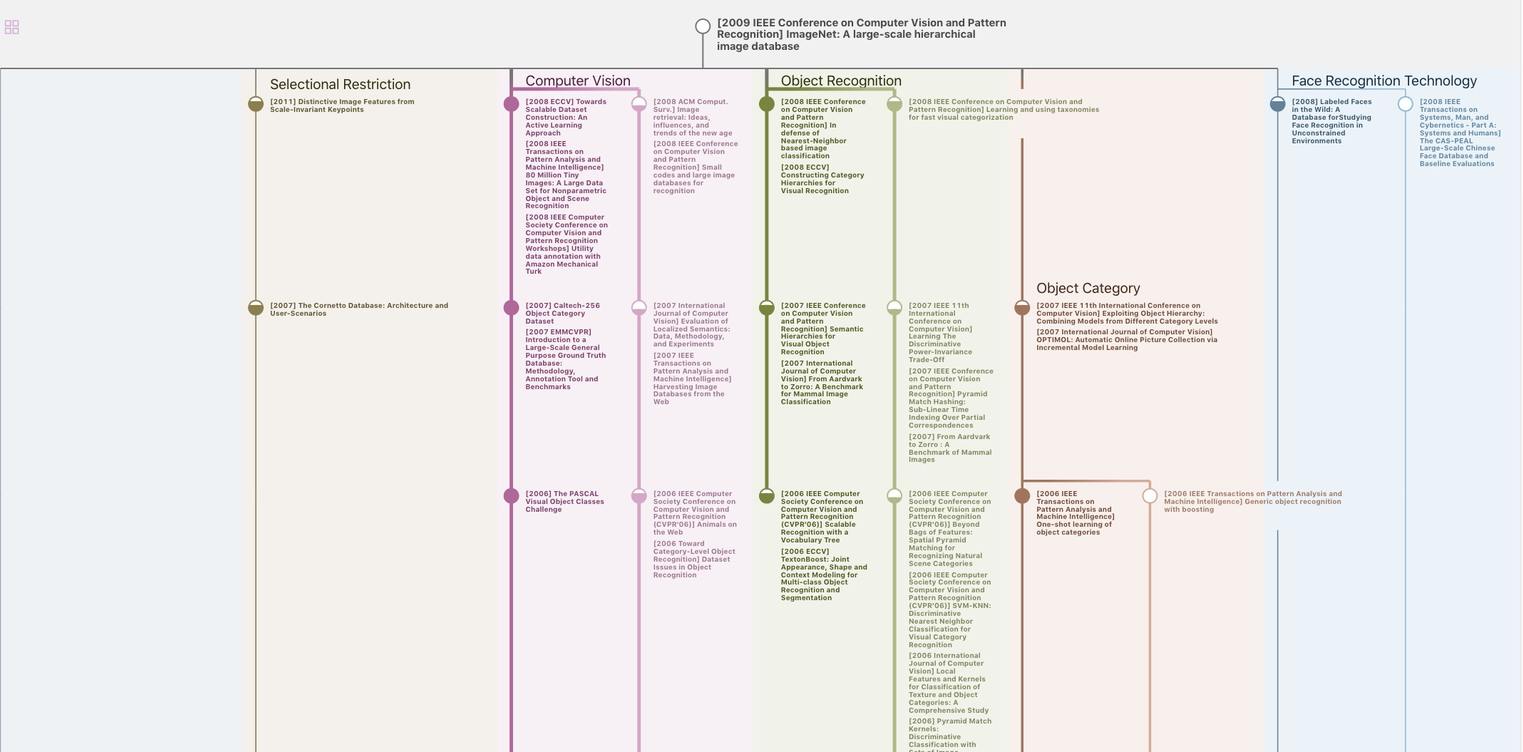
生成溯源树,研究论文发展脉络
Chat Paper
正在生成论文摘要