Deep Learning for Wireless Dynamics
2022 IEEE INTERNATIONAL CONFERENCE ON COMMUNICATIONS WORKSHOPS (ICC WORKSHOPS)(2022)
摘要
This paper aims to predict radio channel variations over time by deep learning from channel observations without knowledge of the underlying channel dynamics. In next generation wideband cellular systems, multicarrier transmission for higher data rate leads to high-resolution predicting problem. By leveraging recent advances of deep learning in high-resolution image processing, we propose a purely data-driven deep learning (DL) approach to predicting high-resolution temporal evolution of wideband radio channels. In order to investigate the effect of architectural design choices, we develop and study three deep learning prediction models, namely, baseline, image completion and next-frame prediction models using UNet. Numerical results show that the proposed DL approach achieves a 52% lower prediction error than the traditional approach based on Kalman filter (KF) in mean absolute errors. To quantify impact of channel aging and prediction on precoding performance, we also evaluate the performance degradation due to outdated and predicted channel state information (CSI) compared to perfect CSI. Our simulations show that the proposed DL approach can reduce the performance loss due to channel aging by 71 % through adapting precoding vector to changes in radio channel while the traditional KF approach only shows a 27% reduction.
更多查看译文
关键词
Radio channel, channel prediction, deep learning, UNet
AI 理解论文
溯源树
样例
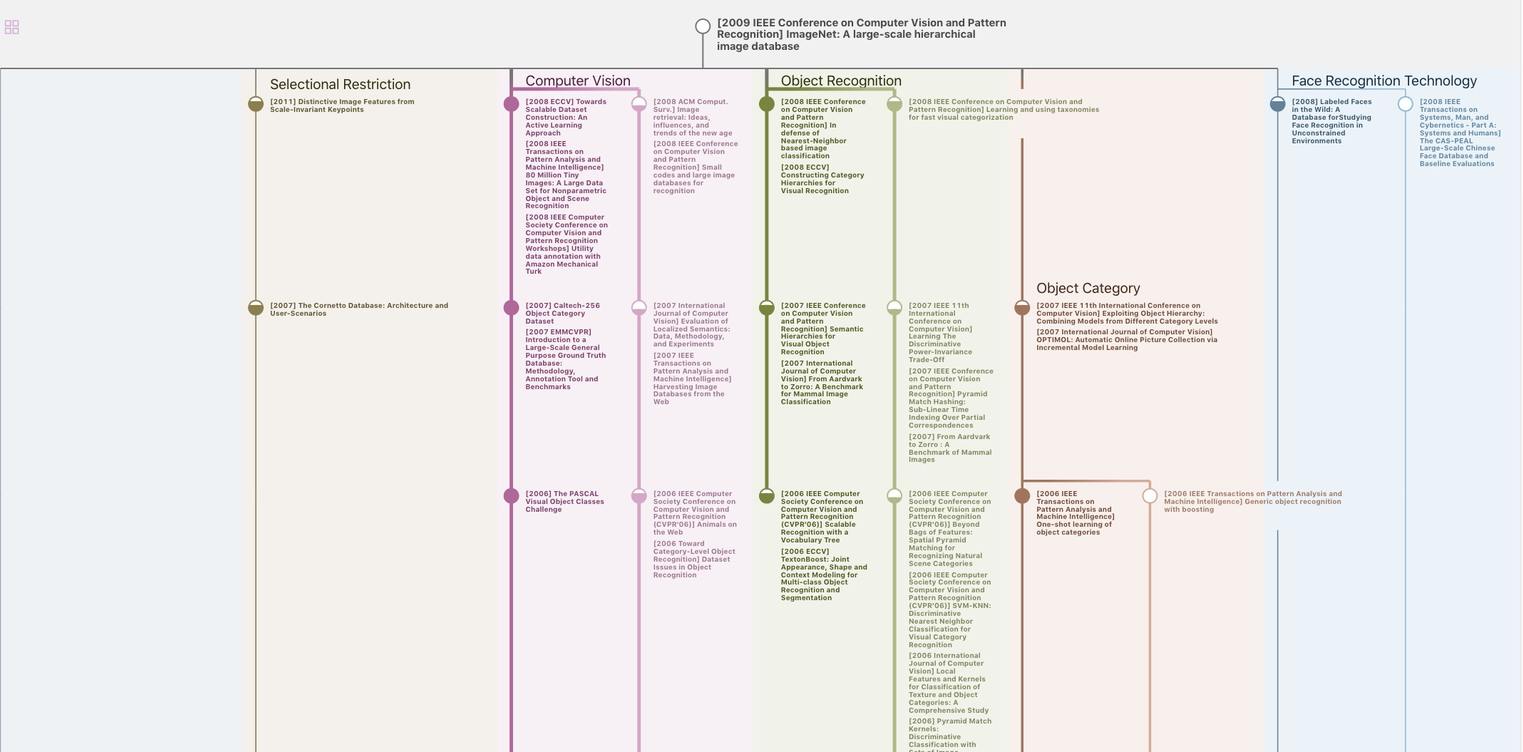
生成溯源树,研究论文发展脉络
Chat Paper
正在生成论文摘要