Geometric Synthesis: A Free lunch for Large-scale Palmprint Recognition Model Pretraining
arxiv(2022)
摘要
Palmprints are private and stable information for biometric recognition. In the deep learning era, the development of palmprint recognition is limited by the lack of sufficient training data. In this paper, by observing that palmar creases are the key information to deep-learning-based palmprint recognition, we propose to synthesize training data by manipulating palmar creases. Concretely, we introduce an intuitive geometric model which represents palmar creases with parameterized B\'ezier curves. By randomly sampling B\'ezier parameters, we can synthesize massive training samples of diverse identities, which enables us to pretrain large-scale palmprint recognition models. Experimental results demonstrate that such synthetically pretrained models have a very strong generalization ability: they can be efficiently transferred to real datasets, leading to significant performance improvements on palmprint recognition. For example, under the open-set protocol, our method improves the strong ArcFace baseline by more than 10\% in terms of TAR@1e-6. And under the closed-set protocol, our method reduces the equal error rate (EER) by an order of magnitude.
更多查看译文
关键词
model pretraining,recognition
AI 理解论文
溯源树
样例
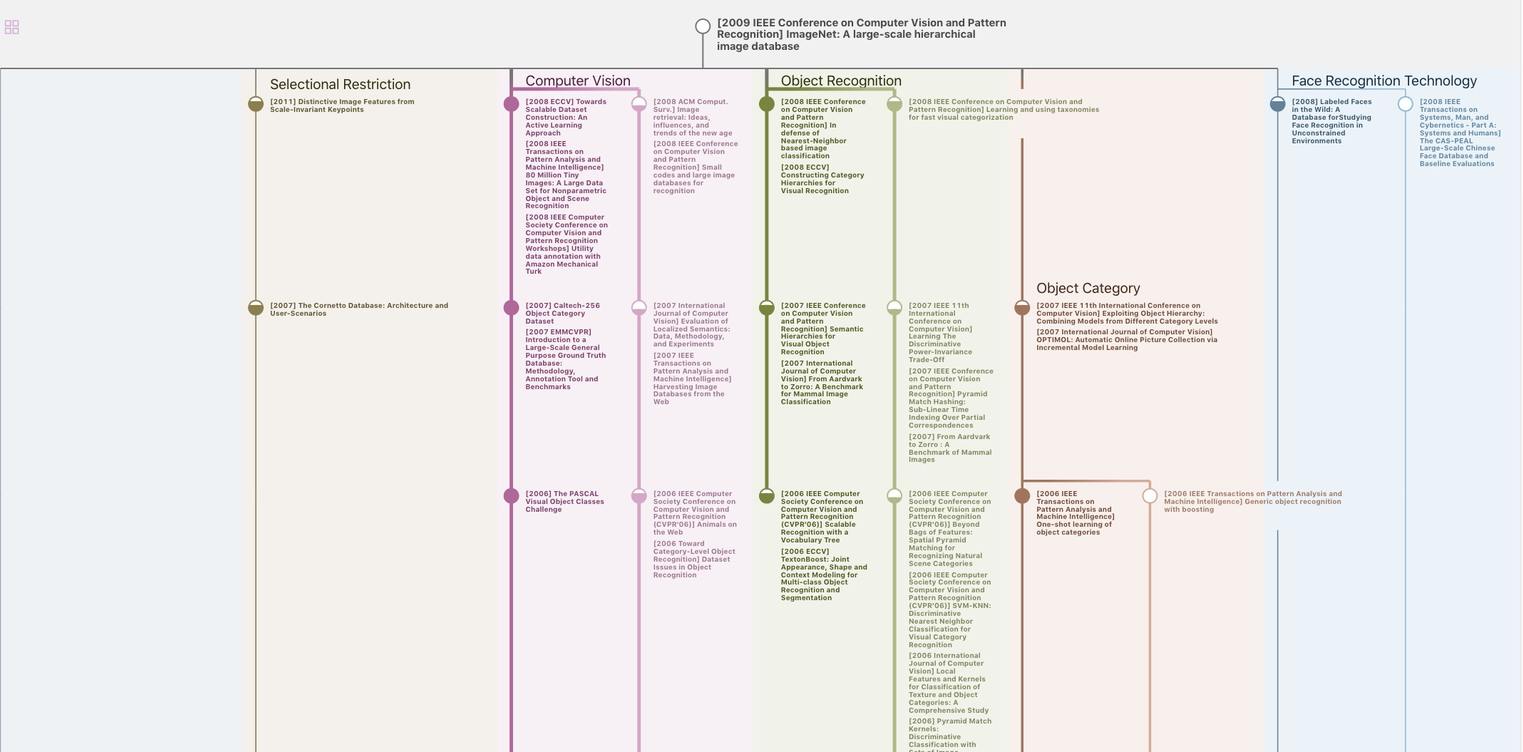
生成溯源树,研究论文发展脉络
Chat Paper
正在生成论文摘要