Unsupervised Learning in Drug Design from Self-Organization to Deep Chemistry
INTERNATIONAL JOURNAL OF MOLECULAR SCIENCES(2022)
摘要
The availability of computers has brought novel prospects in drug design. Neural networks (NN) were an early tool that cheminformatics tested for converting data into drugs. However, the initial interest faded for almost two decades. The recent success of Deep Learning (DL) has inspired a renaissance of neural networks for their potential application in deep chemistry. DL targets direct data analysis without any human intervention. Although back-propagation NN is the main algorithm in the DL that is currently being used, unsupervised learning can be even more efficient. We review self-organizing maps (SOM) in mapping molecular representations from the 1990s to the current deep chemistry. We discovered the enormous efficiency of SOM not only for features that could be expected by humans, but also for those that are not trivial to human chemists. We reviewed the DL projects in the current literature, especially unsupervised architectures. DL appears to be efficient in pattern recognition (Deep Face) or chess (Deep Blue). However, an efficient deep chemistry is still a matter for the future. This is because the availability of measured property data in chemistry is still limited.
更多查看译文
关键词
drug design, deep learning, deep chemistry, self-organizing maps, unsupervised learning, supervised learning, feature engineering, feature learning, molecular representation
AI 理解论文
溯源树
样例
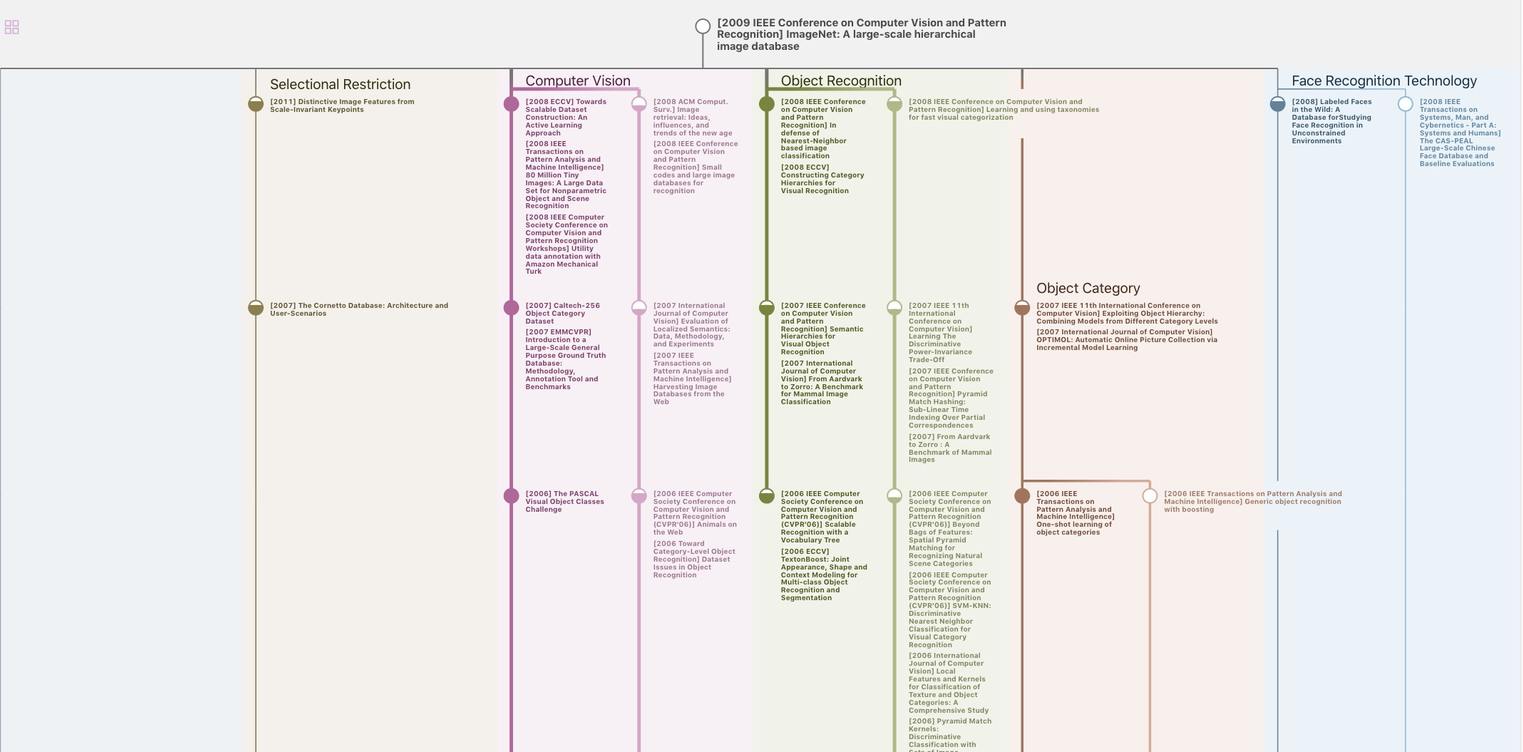
生成溯源树,研究论文发展脉络
Chat Paper
正在生成论文摘要