Prediction of Compressive Strength of Partially Saturated Concrete Using Machine Learning Methods
MATERIALS(2022)
摘要
The aim of this research is to recommend a set of criteria for estimating the compressive strength of concrete under marine environment with various saturation and salinity conditions. Cylindrical specimens from three different design mixtures are used as concrete samples. The specimens are subjected to different saturation levels (oven-dry, saturated-surface dry and three partially dry conditions: 25%, 50% and 75%) on water and water-NaCl solutions. Three parameters (P- and S-wave velocities and electrical resistivity) of concrete are measured using two NDT equipment in the laboratory while two parameters (density and water-to-binder ratio) are obtained from the design documents of the concrete cylinders. Three different machine learning methods, which include, artificial neural network (ANN), support vector machine (SVM) and Gaussian process regression (GPR), are used to obtain multivariate prediction models for compressive strength from multiple parameters. Based on the R-squared value, ANN results in the highest accuracy of estimation while GPR gives the lowest root-mean-squared error (RMSE). Considering both the data analysis and practicality of the method, the prediction model based on two NDE parameters (P-wave velocity measurement and electrical resistivity) and one design parameter (water-to-binder ratio) is recommended for assessing compressive strength under marine environment.
更多查看译文
关键词
data fusion, ultrasonic pulse velocity, electrical resistivity, marine environment, compressive strength
AI 理解论文
溯源树
样例
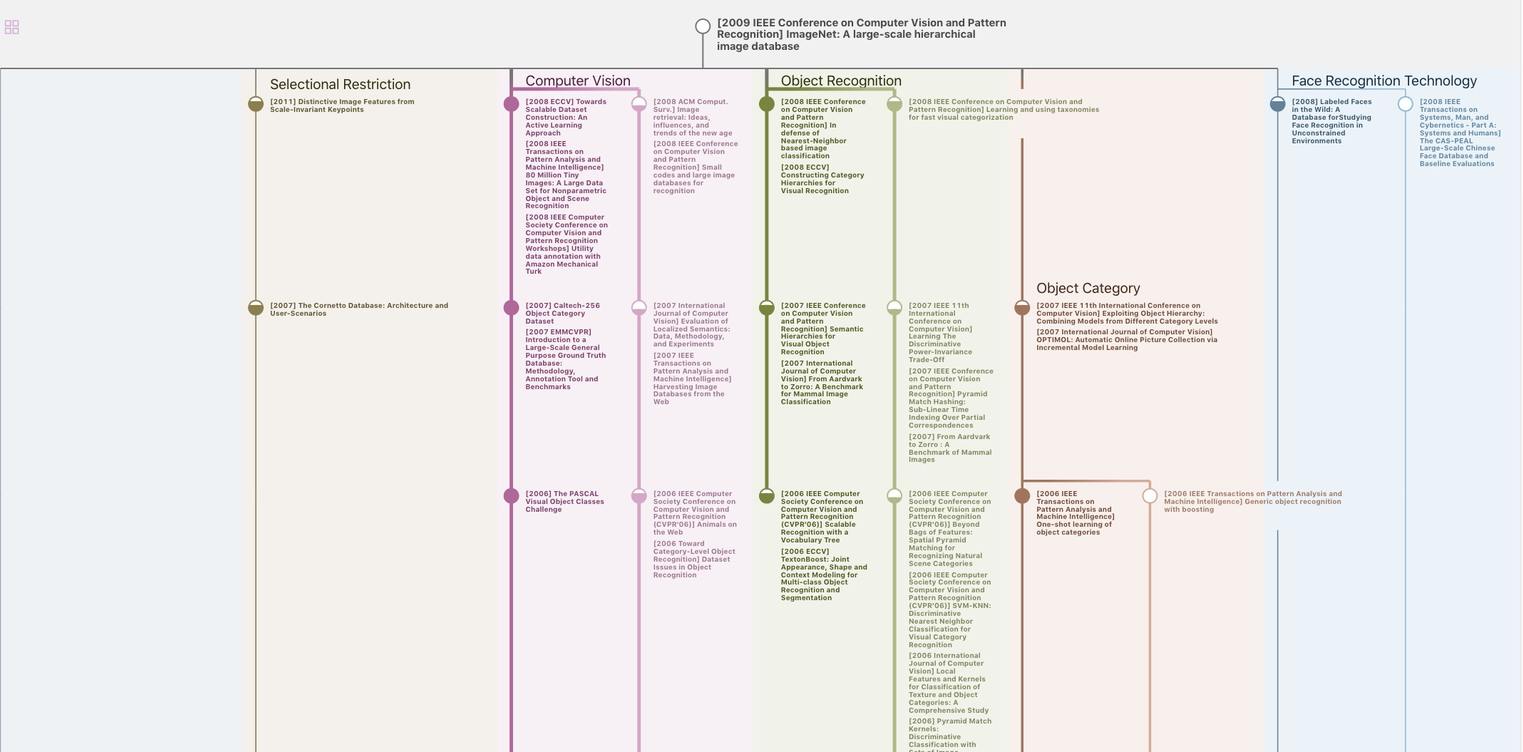
生成溯源树,研究论文发展脉络
Chat Paper
正在生成论文摘要