A Novel COVID-19-Related Drug Discovery Approach Based on Non-Equidimensional Data Clustering
FRONTIERS IN PHARMACOLOGY(2022)
摘要
The novel coronavirus disease (COVID-19) caused by severe acute respiratory syndrome coronavirus-2 (SARS-CoV-2) has spread all over the world. Since currently no effective antiviral treatment is available and those original inhibitors have no significant effect, the demand for the discovery of potential novel SARS-CoV-2 inhibitors has become more and more urgent. In view of the availability of the inhibitor-bound SARS-CoV-2 Mpro and PLpro crystal structure and a large amount of proteomics knowledge, we attempted using the existing coronavirus inhibitors to synthesize new ones, which combined the advantages of similar effective substructures for COVID-19 treatment. To achieve this, we first formulated this issue as a non-equidimensional inhibitor clustering and a following cluster center generating problem, where three essential challenges were carefully addressed, which are 1) how to define the distance between pairwise inhibitors with non-equidimensional molecular structure; 2) how to group inhibitors into clusters when the dimension is different; 3) how to generate the cluster center under this non-equidimensional condition. To be more specific, a novel matrix Kronecker product (p, m)-norm . p m & OTIMES; was first defined to induce the distance D-p(A, B) between two inhibitors. Then, the hierarchical clustering approach was conducted to find similar inhibitors, and a novel iterative algorithm-based Kronecker product (p, m)-norm was designed to generate individual cluster centers as the drug candidates. Numerical experiments showed that the proposed methods can find novel drug candidates efficiently for COVID-19, which has provided valuable predictions for further biological evaluations.
更多查看译文
关键词
COVID-19, matrix norm, Kronecker product, non-equidimensional data clustering, cluster center generating
AI 理解论文
溯源树
样例
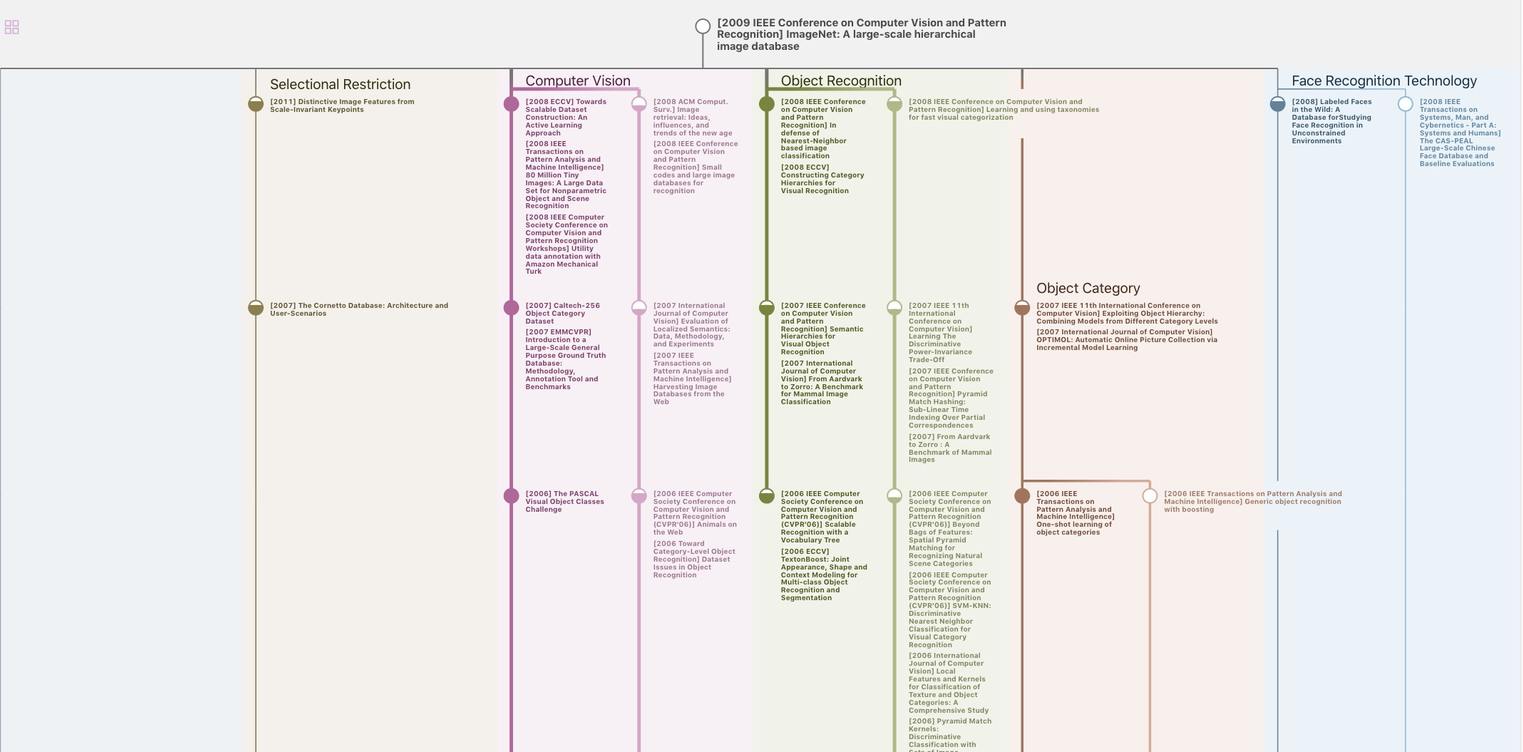
生成溯源树,研究论文发展脉络
Chat Paper
正在生成论文摘要