Asymptotic Bounds for Smoothness Parameter Estimates in Gaussian Process Interpolation
SIAM-ASA JOURNAL ON UNCERTAINTY QUANTIFICATION(2023)
摘要
It is common to model a deterministic response function, such as the output of a computer experiment, as a Gaussian process with a Mat & eacute;rn covariance kernel. The smoothness parameter of a Mat & eacute;rn kernel determines many important properties of the model in the large data limit, including the rate of convergence of the conditional mean to the response function. We prove that the maximum likelihood estimate of the smoothness parameter cannot asymptotically undersmooth the truth when the data are obtained on a fixed bounded subset of R-d. That is, if the data-generating response function has Sobolev smoothness nu(0)>d/2, then the smoothness parameter estimate cannot be asymptotically less than nu(0). The lower bound is sharp. Additionally, we show that maximum likelihood estimation recovers the true smoothness for a class of compactly supported self-similar functions. For cross-validation we prove an asymptotic lower bound nu(0)-d/2, which however is unlikely to be sharp. The results are based on approximation theory in Sobolev spaces and some general theorems that restrict the set of values that the parameter estimators can take.
更多查看译文
关键词
Gaussian processes,parameter estimation,Mate',rn covariance,reproducing kernel Hilbert spaces,fixed-domain asymptotics
AI 理解论文
溯源树
样例
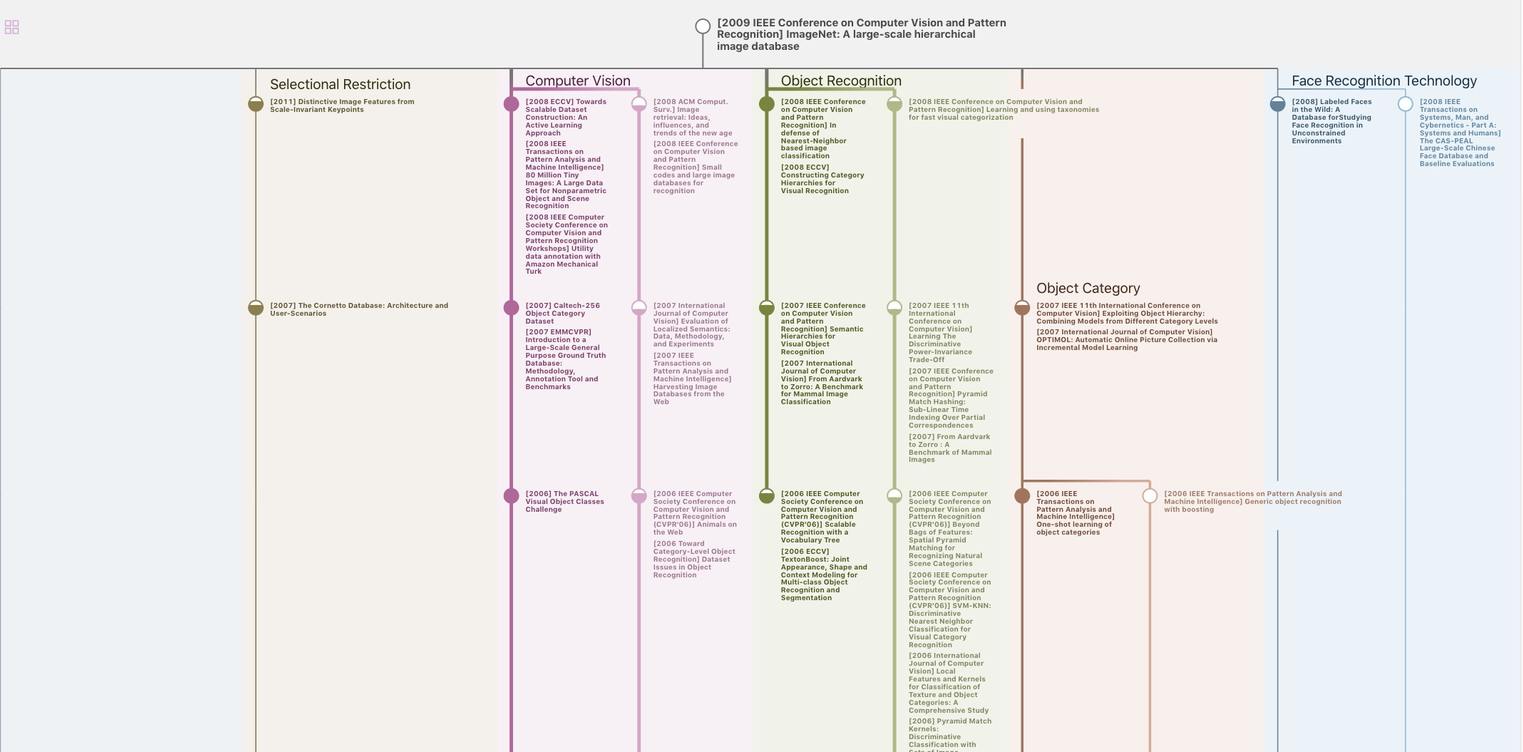
生成溯源树,研究论文发展脉络
Chat Paper
正在生成论文摘要