IAE-Net: Integral Autoencoders for Discretization-Invariant Learning
arxiv(2022)
摘要
Discretization invariant learning aims at learning in the infinite-dimensional function spaces with the capacity to process heterogeneous discrete representations of functions as inputs and/or outputs of a learning model. This paper proposes a novel deep learning framework based on integral autoencoders (IAE-Net) for discretization invariant learning. The basic building block of IAE-Net consists of an encoder and a decoder as integral transforms with data-driven kernels, and a fully connected neural network between the encoder and decoder. This basic building block is applied in parallel in a wide multi-channel structure, which are repeatedly composed to form a deep and densely connected neural network with skip connections as IAE-Net. IAE-Net is trained with randomized data augmentation that generates training data with heterogeneous structures to facilitate the performance of discretization invariant learning. The proposed IAE-Net is tested with various applications in predictive data science, solving forward and inverse problems in scientific computing, and signal/image processing. Compared with alternatives in the literature, IAE-Net achieves state-of-the-art performance in existing applications and creates a wide range of new applications.
更多查看译文
关键词
integral autoencoders,learning,iae-net,discretization-invariant
AI 理解论文
溯源树
样例
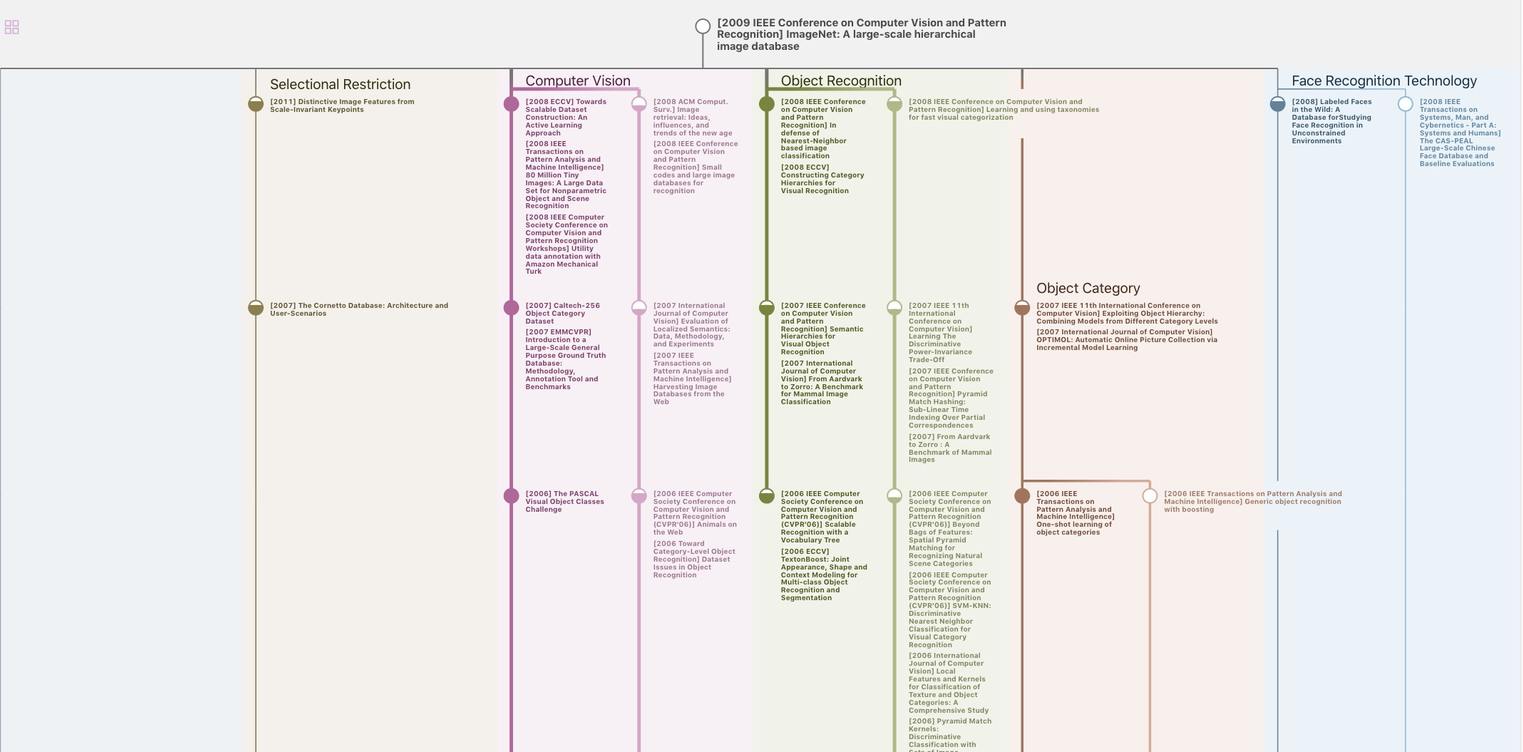
生成溯源树,研究论文发展脉络
Chat Paper
正在生成论文摘要