Neural Data-Dependent Transform for Learned Image Compression
IEEE Conference on Computer Vision and Pattern Recognition(2022)
摘要
Learned image compression has achieved great success due to its excellent modeling capacity, but seldom further considers the Rate-Distortion Optimization (RDO) of each input image. To explore this potential in the learned codec, we make the first attempt to build a neural data-dependent transform and introduce a continuous online mode decision mechanism to jointly optimize the coding efficiency for each individual image. Specifically, apart from the image content stream, we employ an additional model stream to generate the transform parameters at the decoder side. The pres-ence of a model stream enables our model to learn more abstract neural-syntax, which helps cluster the latent repre-sentations of images more compactly. Beyond the transform stage, we also adopt neural-syntax based post-processing for the scenarios that require higher quality reconstructions regardless of extra decoding overhead. Moreover, the in-volvement of the model stream further makes it possible to optimize both the representation and the decoder in an on-line way, i. e. RDO at the testing time. It is equivalent to a continuous online mode decision, like coding modes in the traditional codecs, to improve the coding efficiency based on the individual input image. The experimental results show the effectiveness of the proposed neural-syntax de-sign and the continuous online mode decision mechanism, demonstrating the superiority of our method in coding effi-ciency. Our project is available at: https://dezhao-wang.github.io/Neural-Syntax-Website/.
更多查看译文
关键词
Low-level vision, Computational photography
AI 理解论文
溯源树
样例
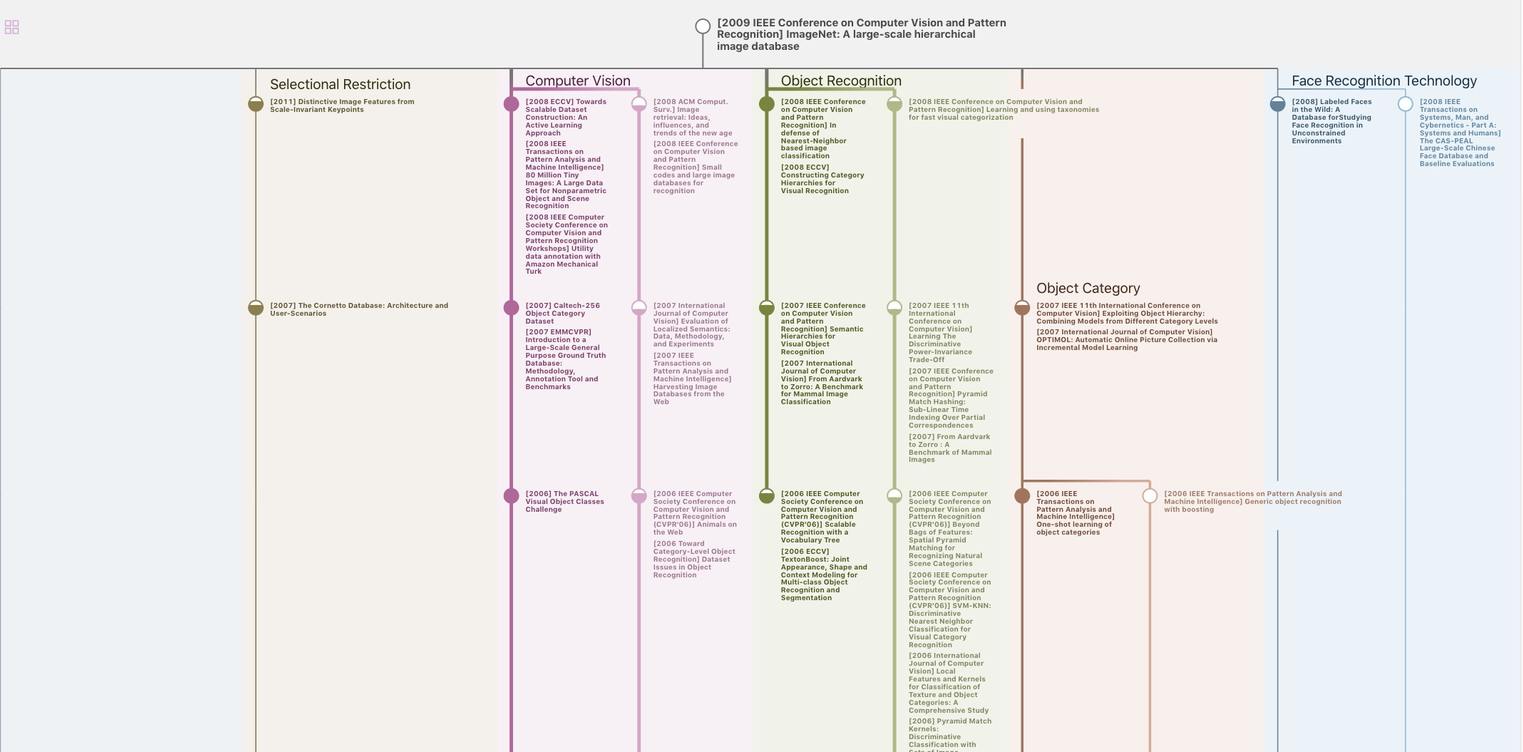
生成溯源树,研究论文发展脉络
Chat Paper
正在生成论文摘要