A Geometry Information Enhanced Unet for Tumor Segmentation
2021 IEEE International Conference on Medical Imaging Physics and Engineering (ICMIPE)(2021)
摘要
Currently in clinical practice, tumor segmentation contributes to diagnosis and determination of radiotherapy area, leading to higher efficiency. In order to offer doctors help in lesion analysis and measurement, segmenting tumors from medical images is investigated in this paper. We propose a geometry information enhanced Unet to solve the problem of edge perception in medical images. In this network, we devise a method of extracting differential geometry information at the input of Unet, which makes full use of the tissue edge information of images to improve the segmentation accuracy of the network. This network makes the edge of tumors clearer by using Jacobian determinant and Laplace operator. Experiments on BraTS2018 dataset are performed to demonstrate that our network has superior performance to the baseline. And we apply our method to the clinical liver tumor CT data to explore practicality of our model in other tumor types or other medical image modality.
更多查看译文
关键词
medical image,differential geometry,Unet,tumor segmentation
AI 理解论文
溯源树
样例
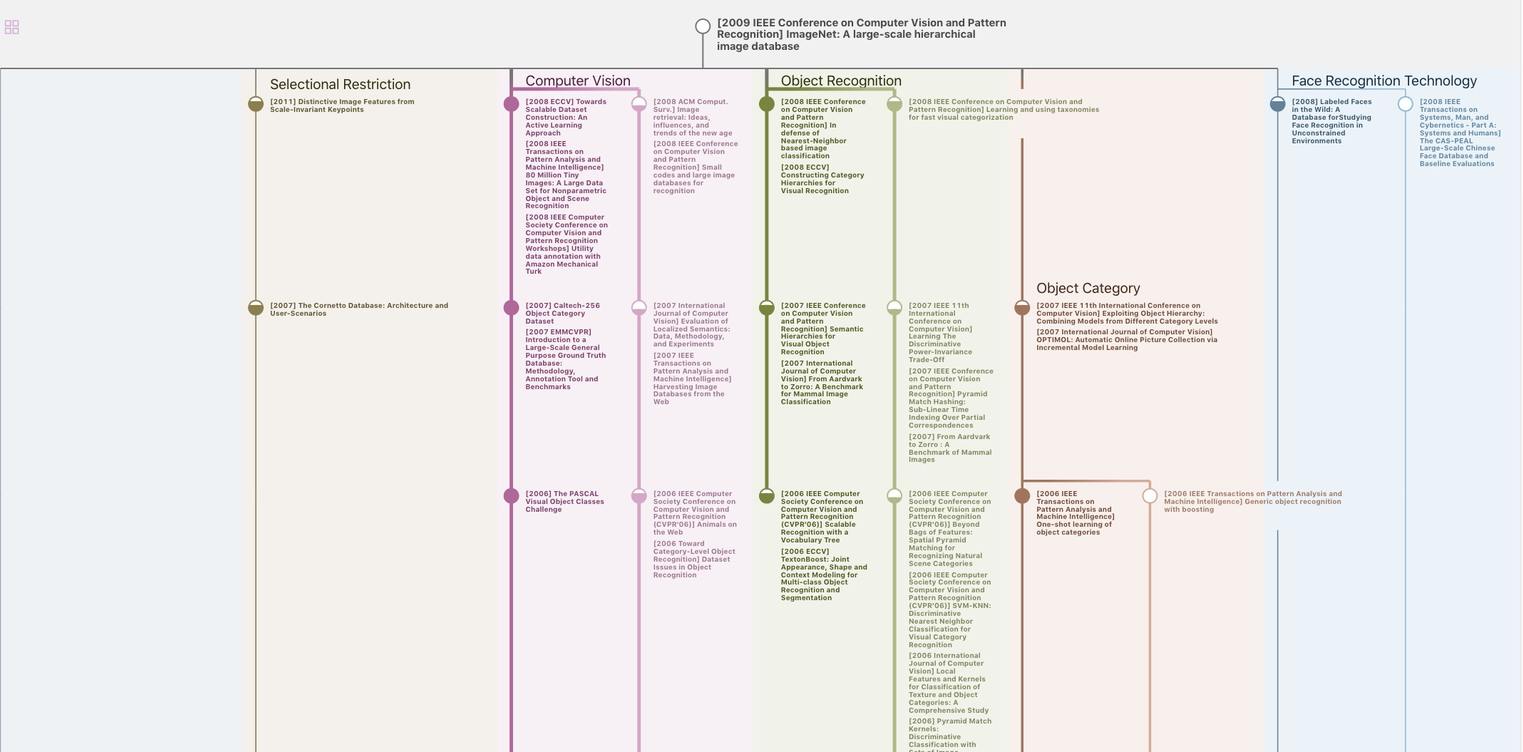
生成溯源树,研究论文发展脉络
Chat Paper
正在生成论文摘要