Microelectronics Failure Prediction and Localization from Optical and Thermal Imagery
2021 IEEE Physical Assurance and Inspection of Electronics (PAINE)(2021)
摘要
Understanding and predicting microelectronic failures is important for ensuring the reliability of modern electronic devices. In this paper, we develop a set of computer vision algorithms for modeling device failure due to electromigration, in which metal atoms are displaced due to current flow. The experimental setup contains a series of optical and thermal images of aluminum interconnects. We propose deep neural networks for two specific problems: predicting where in the device a failure will occur, and estimating the remaining lifespan before failure. We pose the former as a segmentation problem and solve it with a convolutional neural network (CNN) trained on multi-scale optical images. We pose the latter as a regression problem for which we designed a convolutional neural network augmented with a recurrent module (RNN) to model the temporal dimension. We compare against two baseline networks, finding that our model can predict the age of the aluminum more accurately from the optical images instead of the thermal ones. This work is the first that pursues a deep neural network-based modeling to predict electromigration failures from experimental optical and thermal images.
更多查看译文
AI 理解论文
溯源树
样例
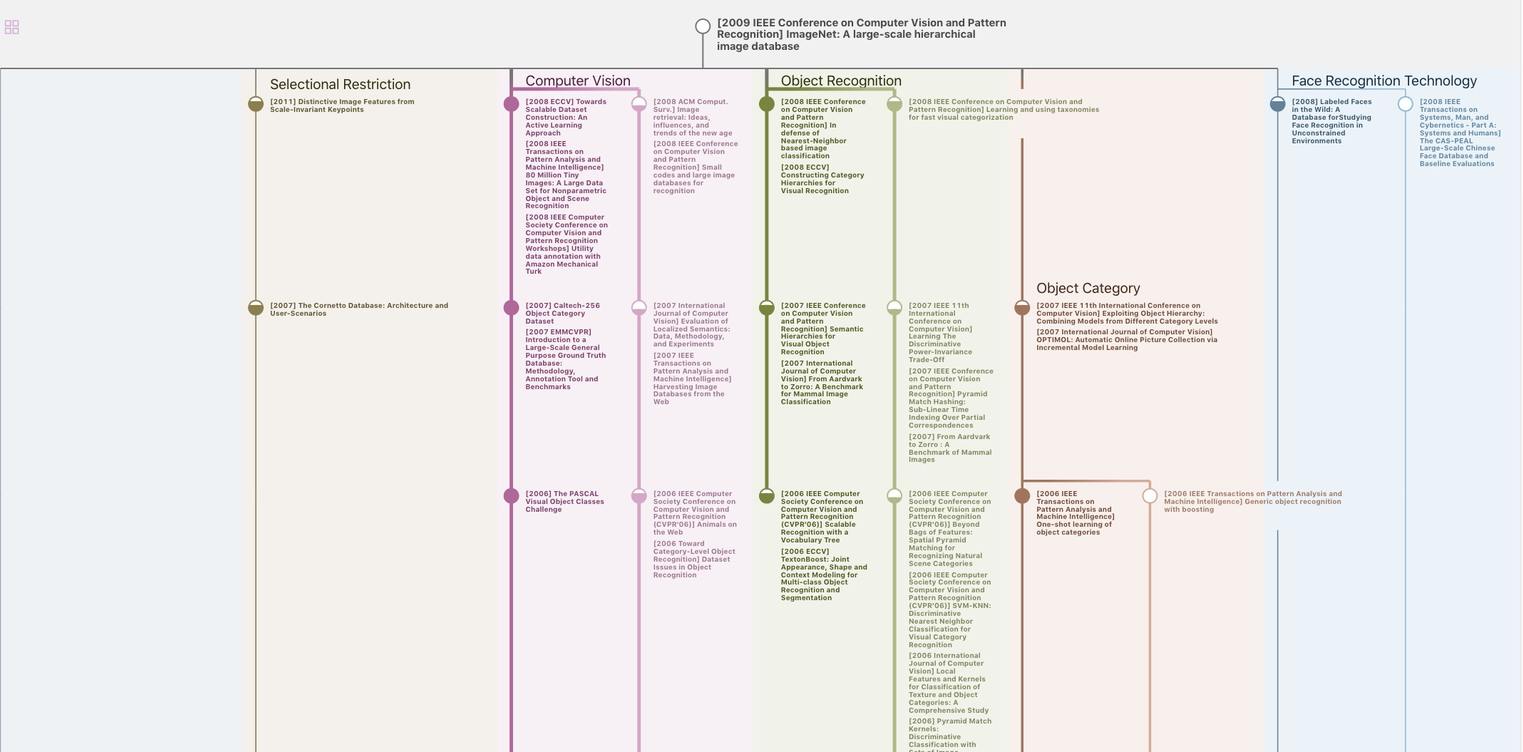
生成溯源树,研究论文发展脉络
Chat Paper
正在生成论文摘要