Detecting the linkage between arable land use and poverty using machine learning methods at global perspective
GEOGRAPHY AND SUSTAINABILITY(2022)
摘要
Eradicating extreme poverty is one of the UN's primary sustainable development goals (SDG). Arable land is related to eradicating poverty (SDG1) and hunger (SDG2). However, the linkage between arable land use and poverty reduction is ambiguous and has seldom been investigated globally. Six indicators of agricultural inputs, crop intensification and extensification were used to explore the relationship between arable land use and poverty. Non-parametric machine learning methods were used to analyze the linkage between agriculture and poverty at the global scale, including the classification and regression tree (CART) and random forest models. We found that the yield gap, fertilizer consumption and potential cropland ratio in protected areas correlated with poverty. Developing countries usually had a ratio of actual to potential yield less than 0.33 and fertilizer consumption less than 7.31 kg/ha. Overall, crop extensification, intensification and agricultural inputs were related to poverty at the global level.
更多查看译文
关键词
Arable land use,Poverty,Machine leaming,Yield gap,Random forest
AI 理解论文
溯源树
样例
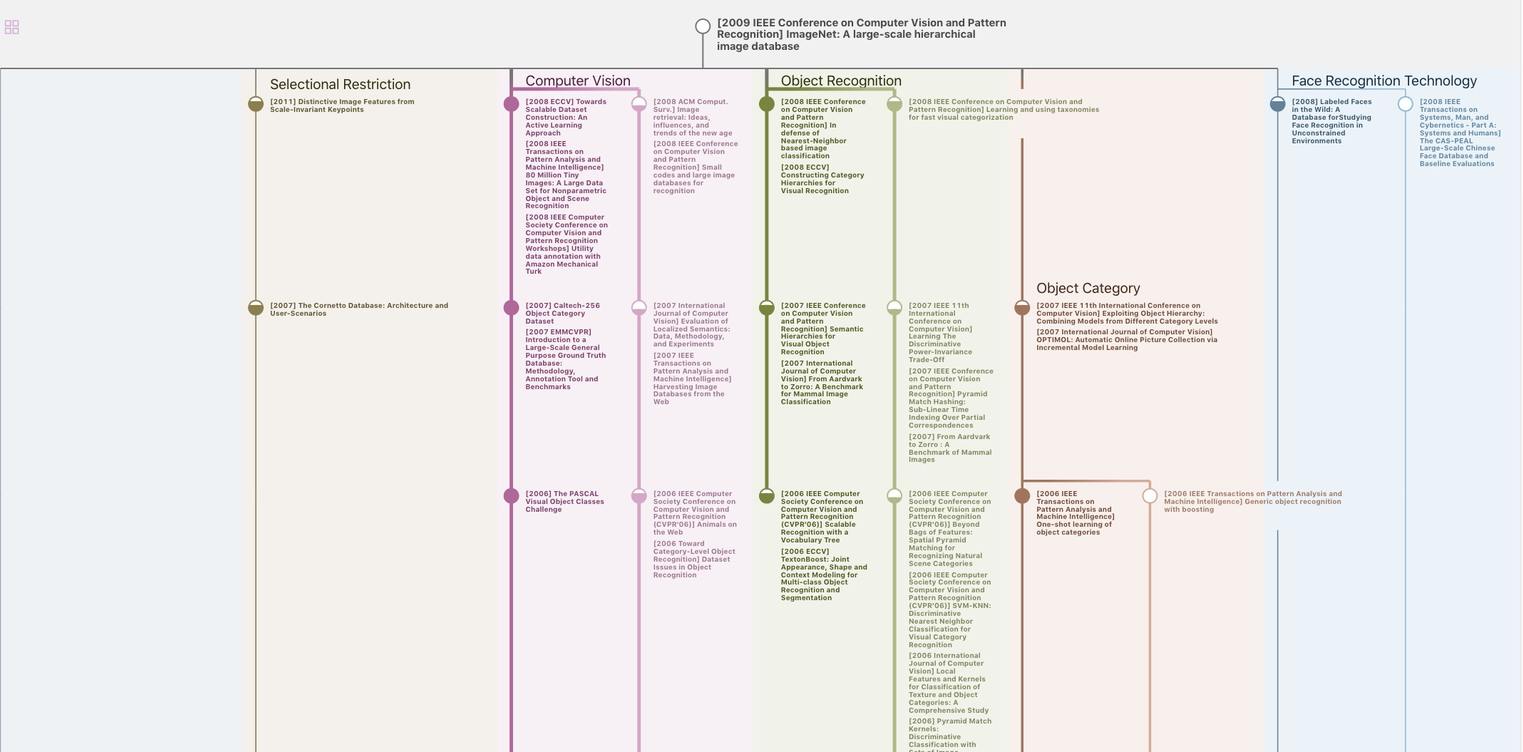
生成溯源树,研究论文发展脉络
Chat Paper
正在生成论文摘要