PRNet++: Learning towards generalized occluded pedestrian detection via progressive refinement network
Neurocomputing(2022)
摘要
Pedestrian detection has achieved significant progress in recent years. Though promising results have been obtained on standard pedestrians, it remains challenging to detect pedestrians in various occlusion situations. In this paper, we propose Progressive Refinement Network (PRNet), a novel single-stage detector for occluded pedestrian detection. Inspired by human’s progressive process on annotating occluded pedestrians, PRNet perform sequential refinement in a single-stage detection framework by three phases: finding anchors of visible parts with high confidence, calibrating these anchors with a full body template derived from occlusion statistics, and adjusting the calibrated anchors to target full-body regions. Unlike conventional methods that utilize predefined anchors directly for full-body estimation, the proposed confidence-aware anchor calibration offers an adaptive anchor initialization for detection with occlusions, while helps reduce the gap between visible-part and full-body detection. We also propose an occlusion loss, which automatically up-weights heavily occluded pedestrian samples. In addition, a Receptive Field Backfeed (RFB) module is introduced to diversify receptive fields in early layers that commonly fire only on visible parts or small-size full-body regions. To further learn generalized representations of pedestrians in different occlusion states, we propose to establish a new single-stage detector with dual-stream architecture namely PRNet++. An Easy-branch and a Hard-branch are designed to learn complementary representation that are more robust to various occlusions. Moreover, the generalization ability of models for other domains are critical in real-world applications, but it has not attracted too much attention. To address this issue, we introduce the unsupervised domain adaptation setting. To handle occlusion situations in unknown domain better, we especially design a Dynamic Iterative adaptation strategy and a Multi-experts adaptation strategy for our occlusion-aware detectors PRNet and PRNet++. Extensive supervised within-dataset experiments and unsupervised domain adaptation experiments were performed on CityPersons, Caltech, ECP-night, KITTI and INRIA datasets, which can validate the effectiveness of our proposed methods in occluded pedestrian detection.
更多查看译文
关键词
Generalized Occluded Pedestrian Detection,Progressive Refinement Network,Dual-stream architecture,Unsupervised domain adaptation,Dynamic Iterative adaptation strategy,Multi-experts adaptation strategy
AI 理解论文
溯源树
样例
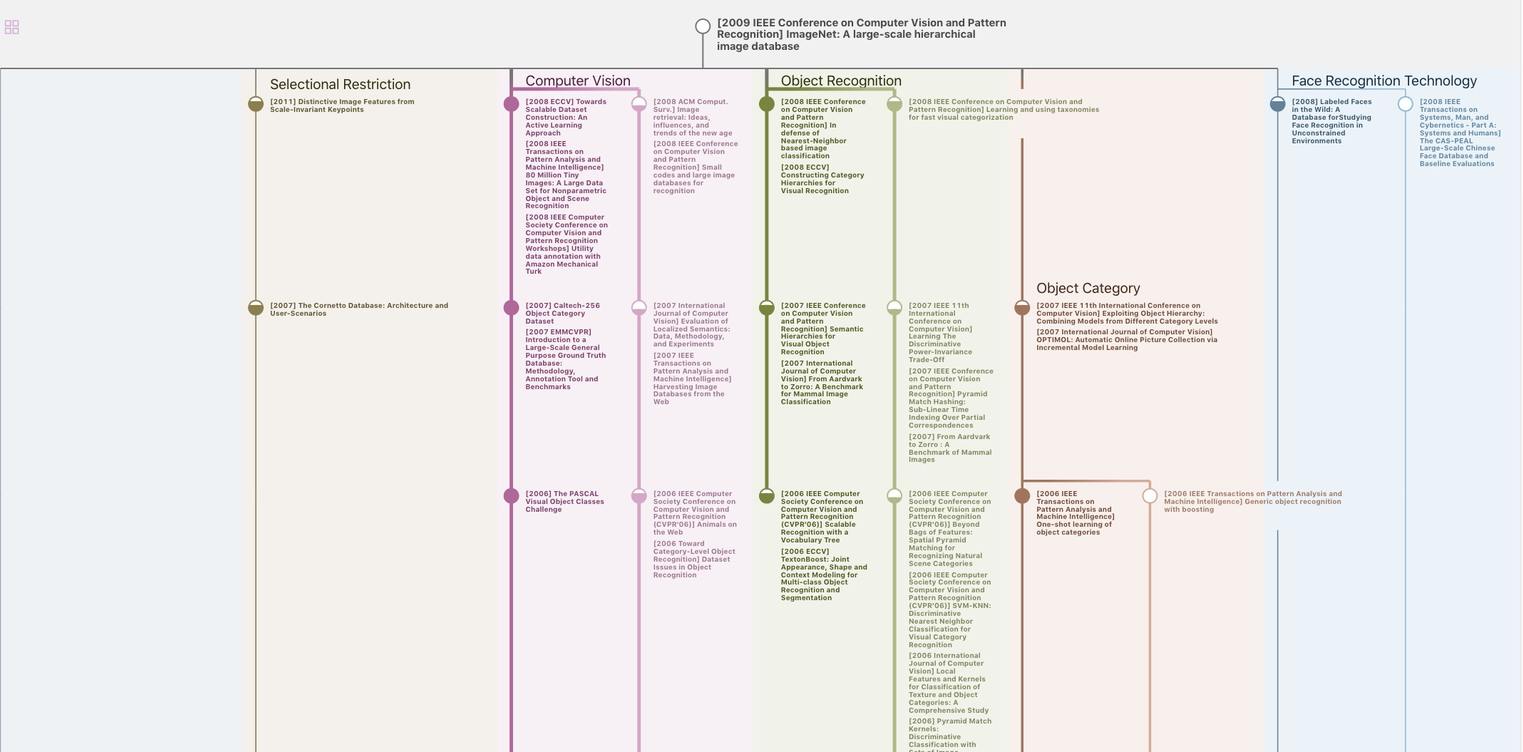
生成溯源树,研究论文发展脉络
Chat Paper
正在生成论文摘要