Using Segmentation With Multi-Scale Selective Kernel for Visual Object Tracking
IEEE SIGNAL PROCESSING LETTERS(2022)
摘要
Generic visual object tracking is challenging due to various difficulties, e.g. scale variations and deformations. To solve those problems, we propose a novel multi-scale selective kernel module for tracking, which contains small-scale and large-scale branches to model the target at different scales and attention mechanism to capture the more effective appearance information of the target. In our module, we cascade multiple small-scale convolutional blocks as an equivalent large-scale branch to extract large-scale features of the target effectively. Besides, we present a hybrid strategy for feature selection to extract significant information from features of different scales. Based on the current excellent segmentation tracking framework, we propose a novel tracking network that leverages our module at multiple places in the up-sample phase to construct a more accurate and robust appearance model. Extensive experimental results show that our tracker outperforms other state-of-the-art trackers on multiple challenging benchmarks including VOT2018, TrackingNet, DAVIS-2017, and YouTube-VOS-2018 while achieves real-time tracking.
更多查看译文
关键词
Target tracking,Feature extraction,Kernel,Object tracking,Benchmark testing,Annotations,Convolution,Feature selection,object tracking,selective ker-nel,video segmentation
AI 理解论文
溯源树
样例
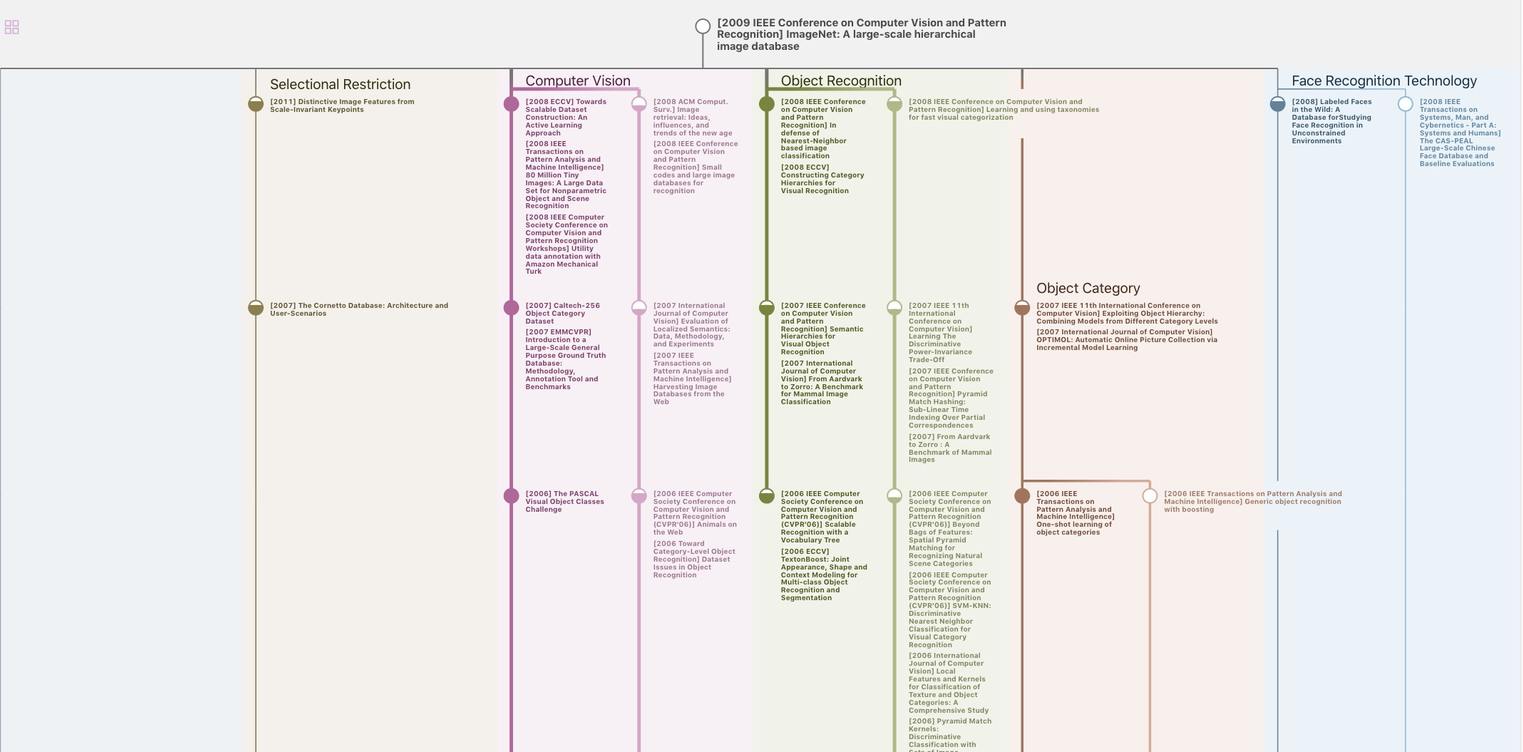
生成溯源树,研究论文发展脉络
Chat Paper
正在生成论文摘要