Lightweight adaptive enhanced attention network for image super-resolution
Multimedia Tools and Applications(2022)
摘要
In recent years, convolutional neural networks have obtained significant success in super-resolution (SR) with remarkable performance. However, as the depth of the network increases, the model parameters and computational overhead become complex. In this paper, we propose a lightweight adaptive enhanced attention network named LAEAN by cascading a series of shared adaptive enhanced attention modules (AEAM). Specifically, the AEAM is implemented by adding a novel up-down sampling module (UDSM) in the position and channel attention modules (PCAM) to adaptively capture wider and richer contextual information. The UDSM is designed to aggregate representative features while enabling the PCAM to learn more discriminative features. Furthermore, we propose a weighted fusion module (WFM) to flexibly combine informative features from all AEAMs for further boosting reconstruction performance. The experimental results demonstrate that our LAEAN is efficient and lightweight with only ∼ 1.5M training parameters, and outperforms most state-of-the-art methods.
更多查看译文
关键词
Super-resolution,Lightweight network,Up-down sampling,Attention mechanism,Weighted fusion
AI 理解论文
溯源树
样例
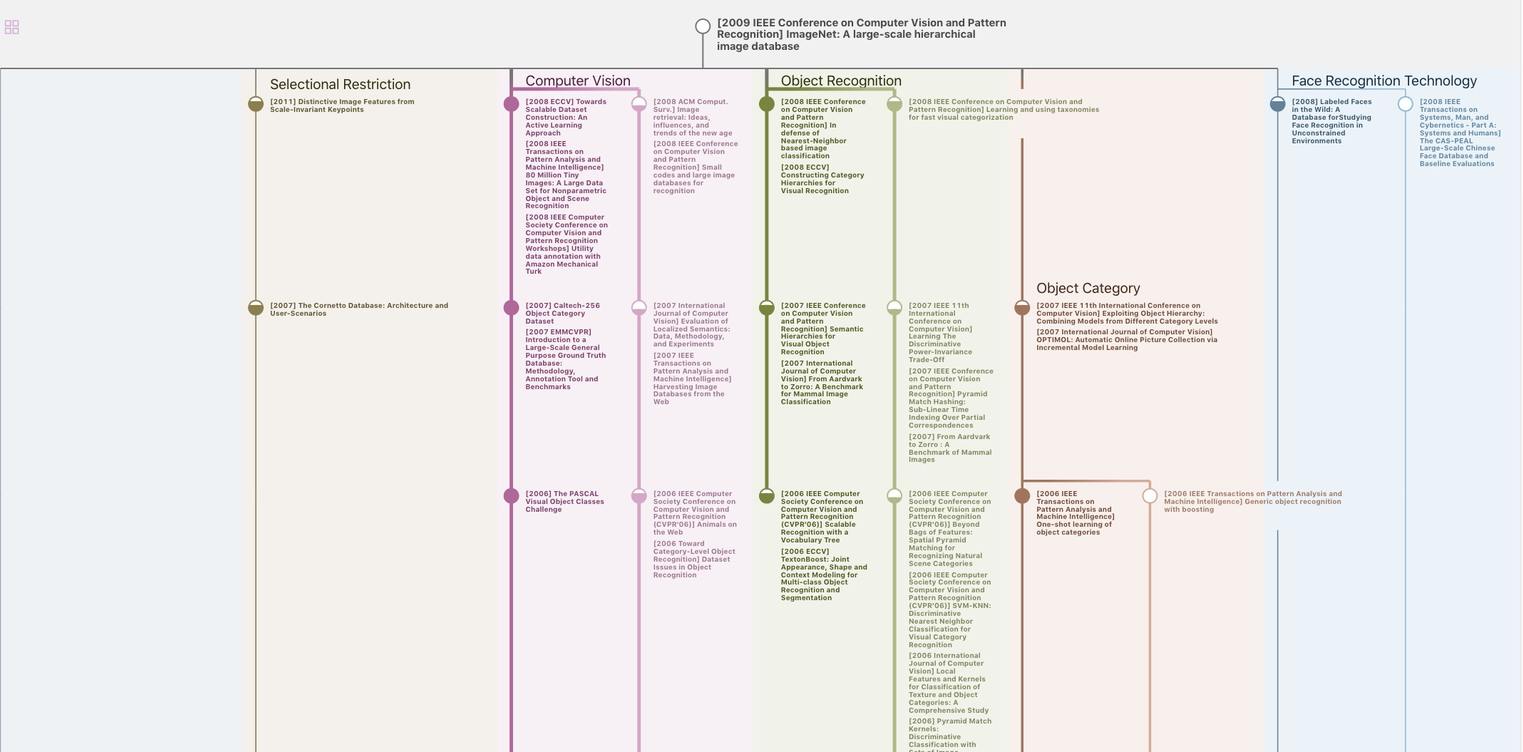
生成溯源树,研究论文发展脉络
Chat Paper
正在生成论文摘要