Confidence-and-Refinement Adaptation Model for Cross-Domain Semantic Segmentation
IEEE TRANSACTIONS ON INTELLIGENT TRANSPORTATION SYSTEMS(2022)
摘要
With the rapid development of convolutional neural networks (CNNs), significant progress has been achieved in semantic segmentation. Despite the great success, such deep learning approaches require large scale real-world datasets with pixel-level annotations. However, considering that pixel-level labeling of semantics is extremely laborious, many researchers turn to utilize synthetic data with free annotations. But due to the clear domain gap, the segmentation model trained with the synthetic images tends to perform poorly on the real-world datasets. Unsupervised domain adaptation (UDA) for semantic segmentation recently gains an increasing research attention, which aims at alleviating the domain discrepancy. Existing methods in this scope either simply align features or the outputs across the source and target domains or have to deal with the complex image processing and post-processing problems. In this work, we propose a novel multi-level UDA model named Confidenceand-Refinement Adaptation Model (CRAM), which contains a confidence-aware entropy alignment (CEA) module and a style feature alignment (SFA) module. Through CEA, the adaptation is done locally via adversarial learning in the output space, making the segmentation model pay attention to the high-confident predictions. Furthermore, to enhance the model transfer in the shallow feature space, the SFA module is applied to minimize the appearance gap across domains. Experiments on two challenging UDA benchmarks "GTAS-to-Cityscapes" and "SYNTHIA-to-Cityscapes" demonstrate the effectiveness of CRAM. We achieve comparable performance with the existing state-of-the-art works with advantages in simplicity and convergence speed.
更多查看译文
关键词
Semantic segmentation,unsupervised domain adaptation,style feature alignment,confidence-aware entropy alignment
AI 理解论文
溯源树
样例
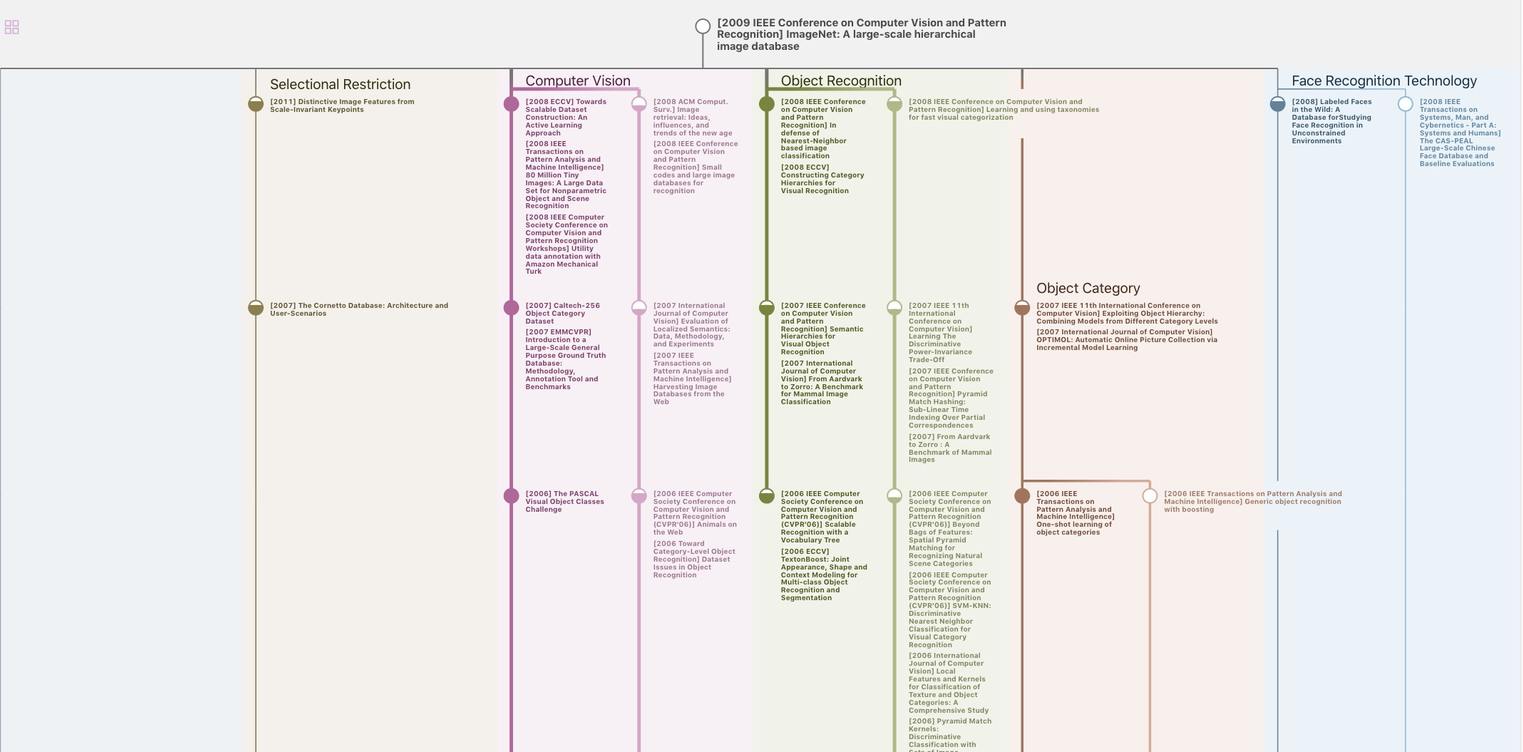
生成溯源树,研究论文发展脉络
Chat Paper
正在生成论文摘要