Automatic recognition and classification of microseismic waveforms based on computer vision
TUNNELLING AND UNDERGROUND SPACE TECHNOLOGY(2022)
摘要
The recognition and classification of microseismic (MS) waveforms detected using MS monitoring are of great importance for predicting instability in rock engineering. The MS waveform can be displayed as an original image of time-amplitude, or it can be converted into a spectrogram, and such images can be classified accurately with the deep learning method in computer vision. Deep learning models, including VGG16, ResNet18, AlexNet, and their ensemble model, are employed to identify and classify MS waveform images and spectrograms. The results show that these models perform well in learning each model of the nondenoised waveform image set with accuracies of AlexNet, VGG16, ResNet18 and the ensemble model on the original waveform data of 0.96, 0.98, 0.96 and 0.98, respectively. However, different models differ in recognising noise, electricity and MS events, which making it necessary to select the model according to the real scenes. The model feature maps can accu-rately explain not only the learning process of the convolution network but also the reason why the test results for the original waveform dataset and spectrogram dataset are similar, thereby realizing end-to-end recognition and classification of the original waveform images. Finally, the mixed signals of MS, noise and electricity are discussed to provide a reference for automatic classification of waveforms in MS monitoring engineering.
更多查看译文
关键词
Microseismic (MS) events,Waveform classification,Convolutional neural networks (CNN),Ensemble learning,Visualizing feature map
AI 理解论文
溯源树
样例
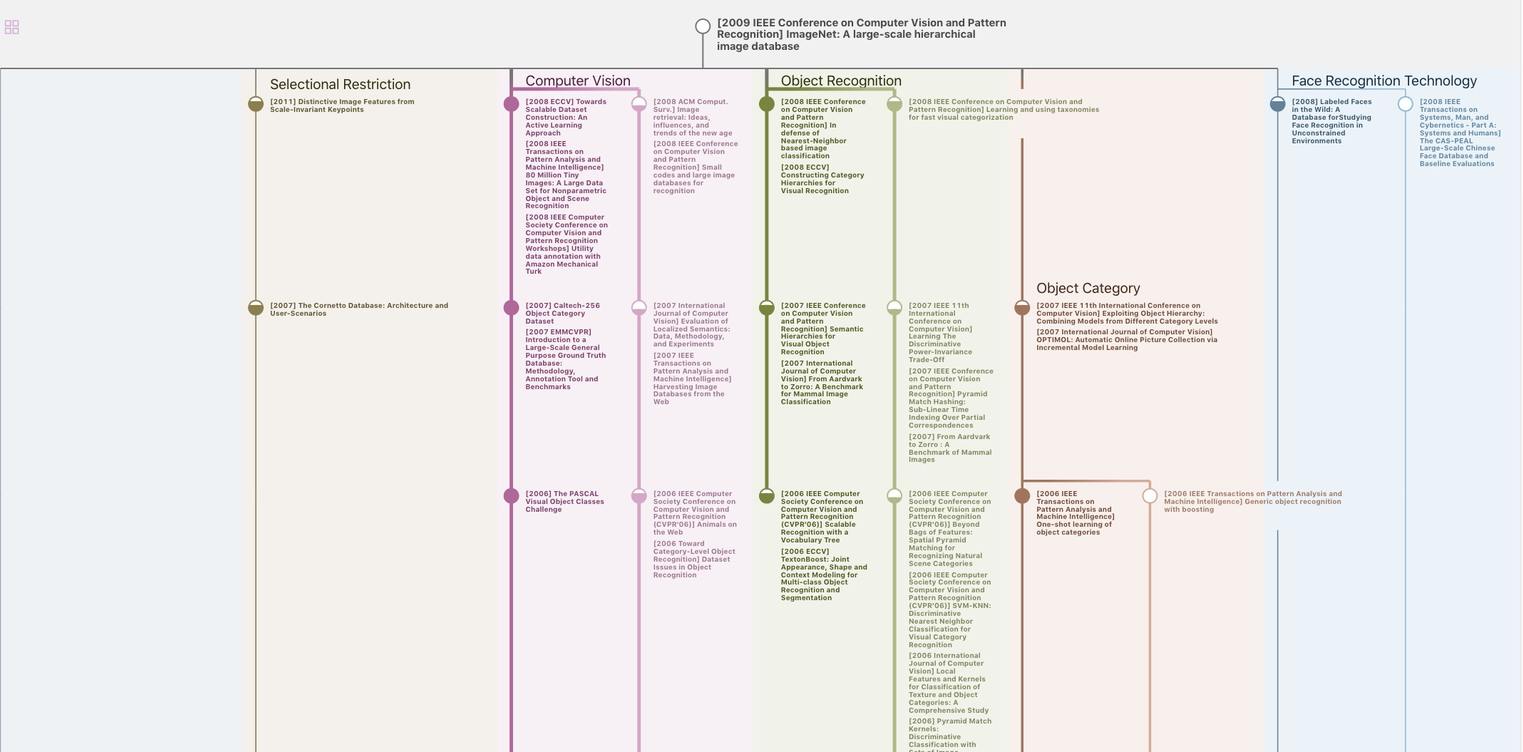
生成溯源树,研究论文发展脉络
Chat Paper
正在生成论文摘要