Semi-Supervised Few-shot Image Classification Based on Subspace Learning
Journal of physics(2022)
摘要
Abstract Few-shot image classification is an image classification technology that uses minimal sample data to train the classifier or classification network and achieves a sure classification accuracy. Compared with the image classification technology based on big data, it has the advantages of an unlimited number of samples and fast processing speed. The purpose of subspace learning is to learn good mapping. This mapping maps high-dimensional image data from visual to low-dimensional semantic information space, which is convenient for information recognition, processing, and classification. Therefore, this paper proposes to use the subspace learning method to extract image features. On this basis, studies semi-supervised few-shot image classification, the self-training semi-supervised algorithm is improved to overcome the performance degradation caused by adding wrong pseudo label samples to the self-training algorithm. It is trained and tested on four mainstream few-shot learning datasets. It has a specific significance for the research and development of few-shot image classification.
更多查看译文
关键词
classification,learning,semi-supervised,few-shot
AI 理解论文
溯源树
样例
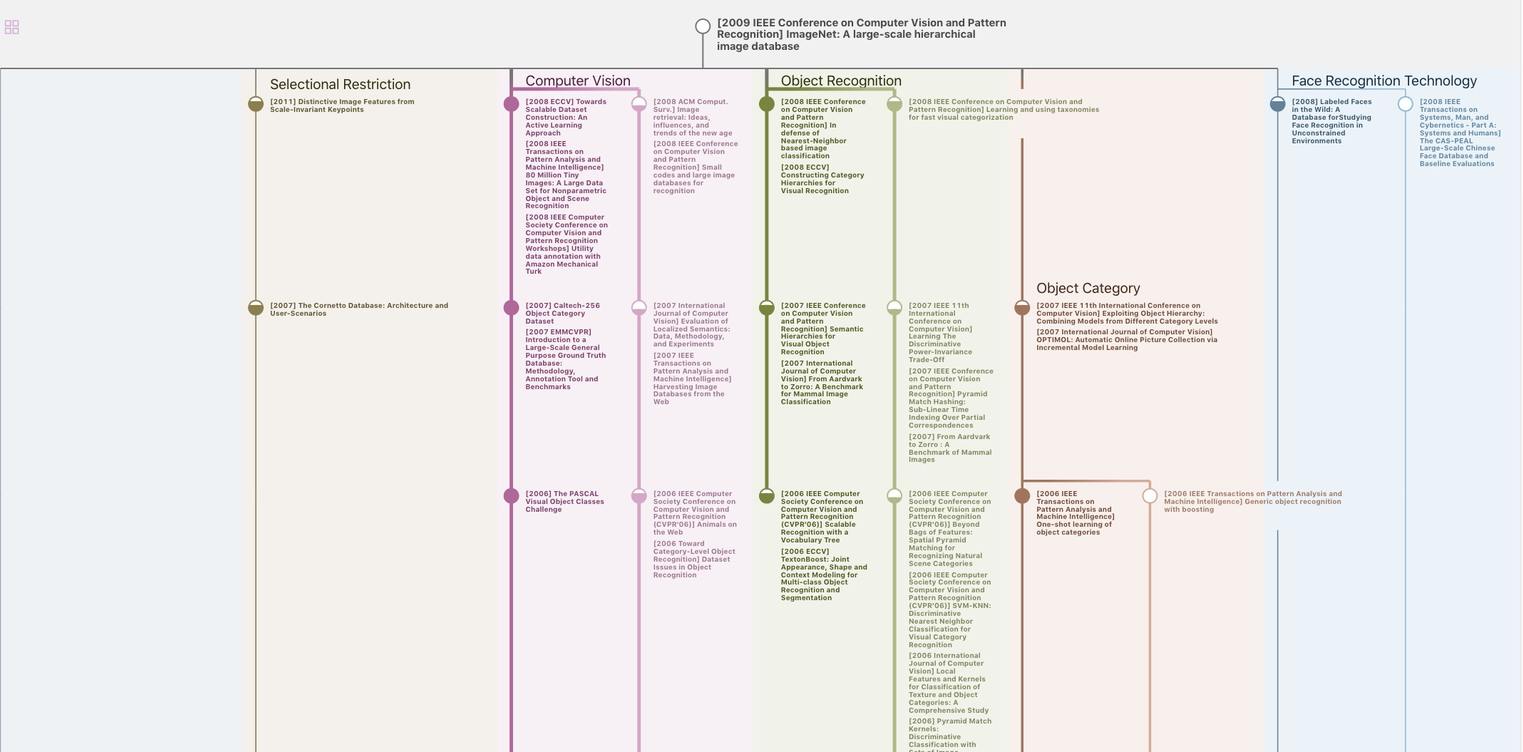
生成溯源树,研究论文发展脉络
Chat Paper
正在生成论文摘要