Accelerating On-Chip Training with Ferroelectric-Based Hybrid Precision Synapse
ACM JOURNAL ON EMERGING TECHNOLOGIES IN COMPUTING SYSTEMS(2022)
摘要
In this article, we propose a hardware accelerator design using ferroelectric transistor (FeFET)-based hybrid precision synapse (HPS) for deep neural network (DNN) on-chip training. The drain erase scheme for FeFET programming is incorporated for both FeFET HPS design and FeFET buffer design. By using drain erase, high-density FeFET buffers can be integrated onchip to store the intermediate input-output activations and gradients, which reduces the energy consuming off-chip DRAM access. Architectural evaluation results show that the energy efficiency could be improved by 1.2x similar to 2.1x, 3.9x similar to 6.0x compared to the other HPS-based designs and emerging non-volatile memory baselines, respectively. The chip area is reduced by 19% similar to 36% compared with designs using SRAM on-chip buffer even though the capacity of FeFET buffer is increased. Besides, by utilizing drain erase scheme for FeFET programming, the chip area is reduced by 11% similar to 28.5% compared with the designs using body erase scheme.
更多查看译文
关键词
Deep neural network,emerging non-volatile memory,ferroelectric field effect transistor (FeFET),DNN hardware acceleration,in-memory computing,on-chip training
AI 理解论文
溯源树
样例
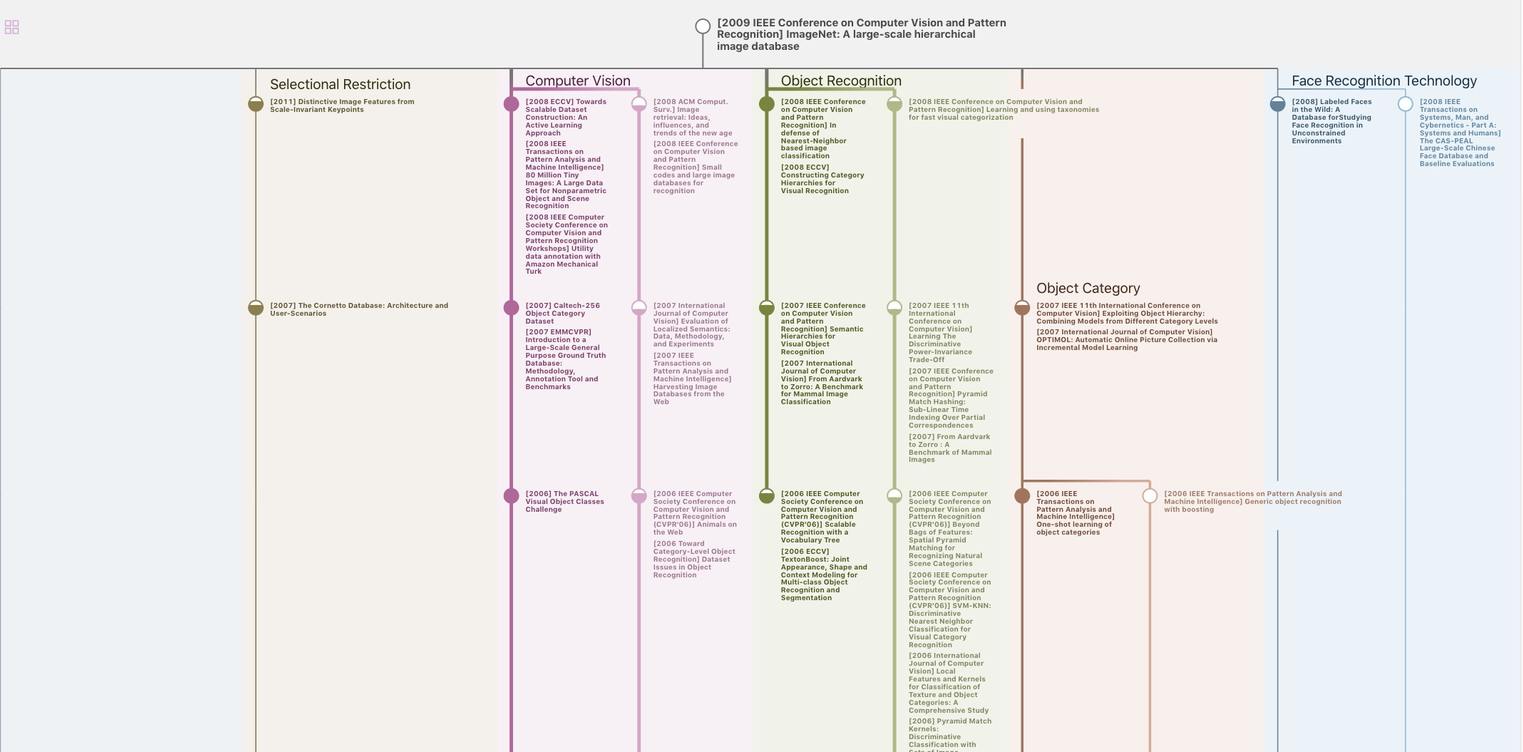
生成溯源树,研究论文发展脉络
Chat Paper
正在生成论文摘要