Novel Insights on Establishing Machine Learning Based Stroke Prediction Models Among Hypertensive Adults
Social Science Research Network(2022)
摘要
BackgroundStroke is a major global health burden, and risk prediction is essential for the primary prevention of stroke. However, uncertainty remains about the optimal prediction model for analyzing stroke risk. In this study, we aim to determine the most effective stroke prediction method in a Chinese hypertensive population using machine learning and establish a general methodological pipeline for future analysis. MethodsThe training set included 70% of data (n = 14,491) from the China Stroke Primary Prevention Trial (CSPPT). Internal validation was processed with the rest 30% of CSPPT data (n = 6,211), and external validation was conducted using a nested case-control (NCC) dataset (n = 2,568). The primary outcome was the first stroke. Four received analysis methods were processed and compared: logistic regression (LR), stepwise logistic regression (SLR), extreme gradient boosting (XGBoost), and random forest (RF). Population characteristic data with inclusion and exclusion of laboratory variables were separately analyzed. Accuracy, sensitivity, specificity, kappa, and area under receiver operating characteristic curves (AUCs) were used to make model assessments with AUCs the top concern. Data balancing techniques, including random under-sampling (RUS) and synthetic minority over-sampling technique (SMOTE), were applied to process this unbalanced training set. ResultsThe best model performance was observed in RUS-applied RF model with laboratory variables. Compared with null models (sensitivity = 0, specificity = 100, and mean AUCs = 0.643), data balancing techniques improved overall performance with RUS, demonstrating a more satisfactory effect in the current study (RUS: sensitivity = 63.9; specificity = 53.7; and mean AUCs = 0.624. Adding laboratory variables improved the performance of analysis methods. All results were reconfirmed in validation sets. The top 10 important variables were determined by the analysis method with the best performance. ConclusionAmong the tested methods, the most effective stroke prediction model in targeted population is RUS-applied RF. From the insights, the current study revealed, we provided general frameworks for building machine learning-based prediction models.
更多查看译文
关键词
machine learning, risk assessment, stroke, primary prevention, XGBoost
AI 理解论文
溯源树
样例
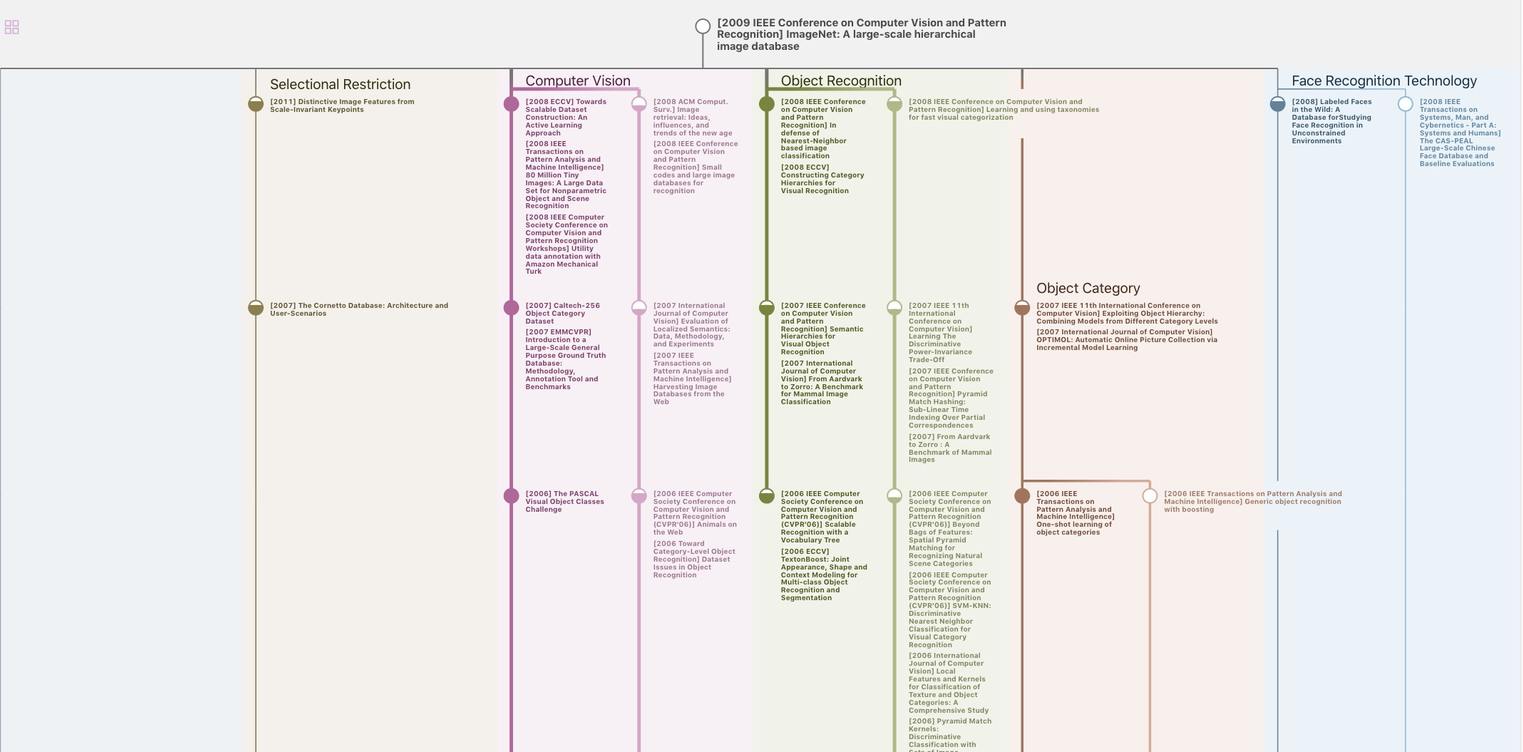
生成溯源树,研究论文发展脉络
Chat Paper
正在生成论文摘要