Influence maximization based on community structure and second-hop neighborhoods
Applied Intelligence(2022)
摘要
With the spread of Internet and big data research and applications, influence propagation in networks becomes one of the hot topics in the field of social network analysis in recent years. Influence Maximization (IM), which selects a set of k seeds from a network to maximize the expected number of influenced nodes, has been extensively studied due to its immense application potential and enormous technical challenges. In this paper, we present a new heuristic method named IMCAN ( I nfluence M aximization based on C ommunity A nd Second-hop N eighbors), which makes utilization of community structure to select the seed nodes. We employ two efficient community detection algorithms, Fast Q and LPA, to extract multiple community structures first, then calculate an influence score for each node by considering the average number of its adjacent communities and its influences among its first- and second-order neighbors. After that, we select the node with the largest influence score as a seed, remove its direct neighbors from the candidate set, attenuate its second-order neighbors’ influence scores, and then choose the node with the largest influence score as the next seed. This procedure is repeated until all the k seeds are selected. The experiments on some real-world networks show that IMCAN can extract the seeds with the larger propagation abilities from networks, it outperforms the comparison algorithms significantly.
更多查看译文
关键词
Influence maximization,Networks,Community structure
AI 理解论文
溯源树
样例
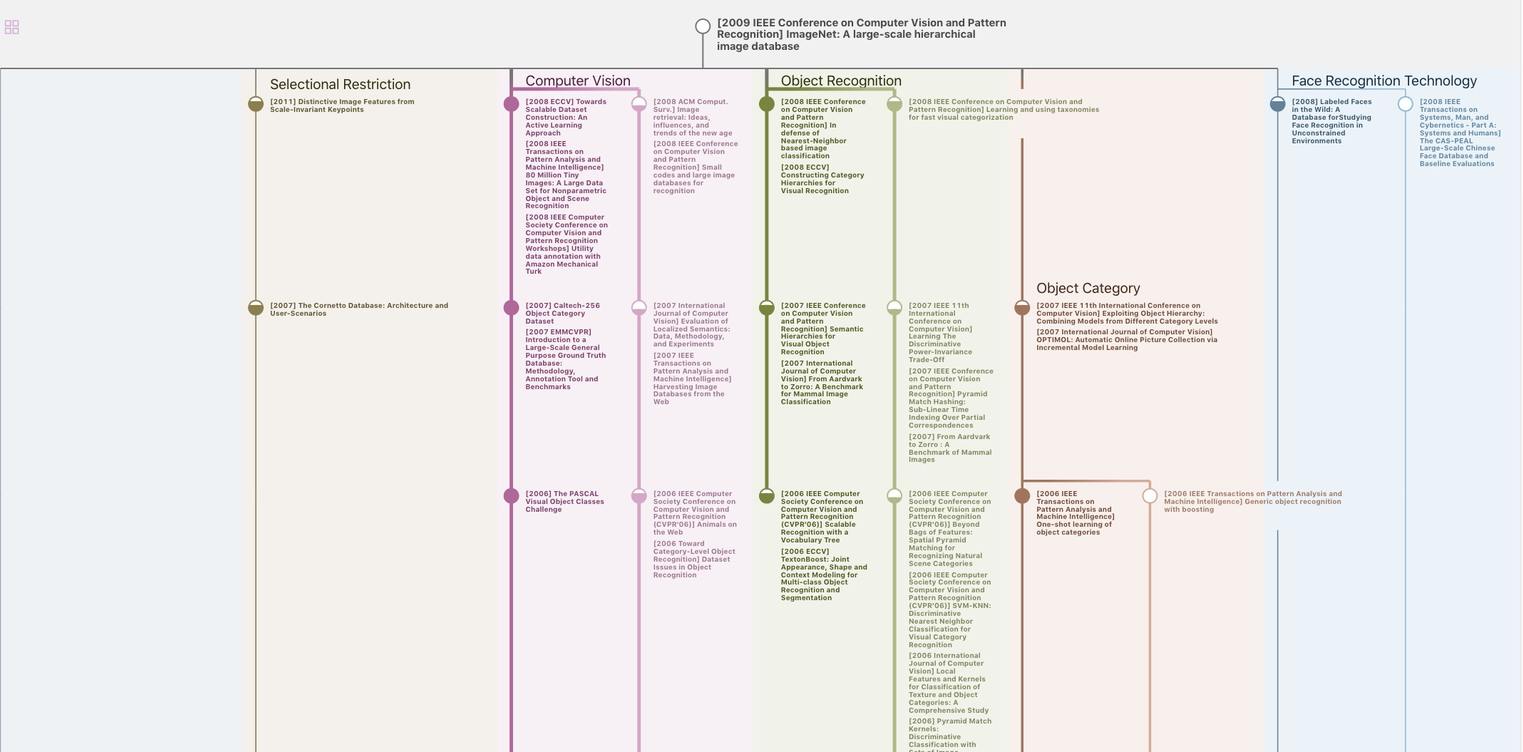
生成溯源树,研究论文发展脉络
Chat Paper
正在生成论文摘要