Deep Reinforcement Learning-Based Joint Optimization of Delay and Privacy in Multiple-User MEC Systems
IEEE Transactions on Cloud Computing(2023)
摘要
Multi-access Edge Computing (MEC) enables mobile users to run various delay-sensitive applications via offloading computation tasks to MEC servers. However, the location privacy and the usage pattern privacy are disclosed to the untrusted MEC servers. The most related work concerning privacy-preserving offloading schemes in MEC either consider an impractical MEC scenario consisting of a single user or take a large amount of computation and communication cost. In this article, we propose a deep reinforcement learning based joint optimization of delay and privacy preservation during offloading for multiple-user wireless powered MEC systems, preserving users' both location privacy and usage pattern privacy. The main idea is that, to protect both the two kinds of privacy, we propose to disguise users' offloading decisions and deliberately offloading redundant tasks along with the actual tasks to the MEC servers. On this basis, we further formalize the task offloading as an optimization problem of computation rate and privacy preservation. Then, we design a deep reinforcement learning based offloading algorithm to solve such an non-convex problem, aiming to obtain the better tradeoff between the computation rate and the privacy preservation. Finally, extensive simulation results demonstrate that our algorithm can maintain a high level of computation rate while protecting users' usage pattern privacy and location privacy, compared with two learning-based methods and two Baselines.
更多查看译文
关键词
Mobile edge computing,task offloading,privacy preservation,deep reinforcement learning,computation rate
AI 理解论文
溯源树
样例
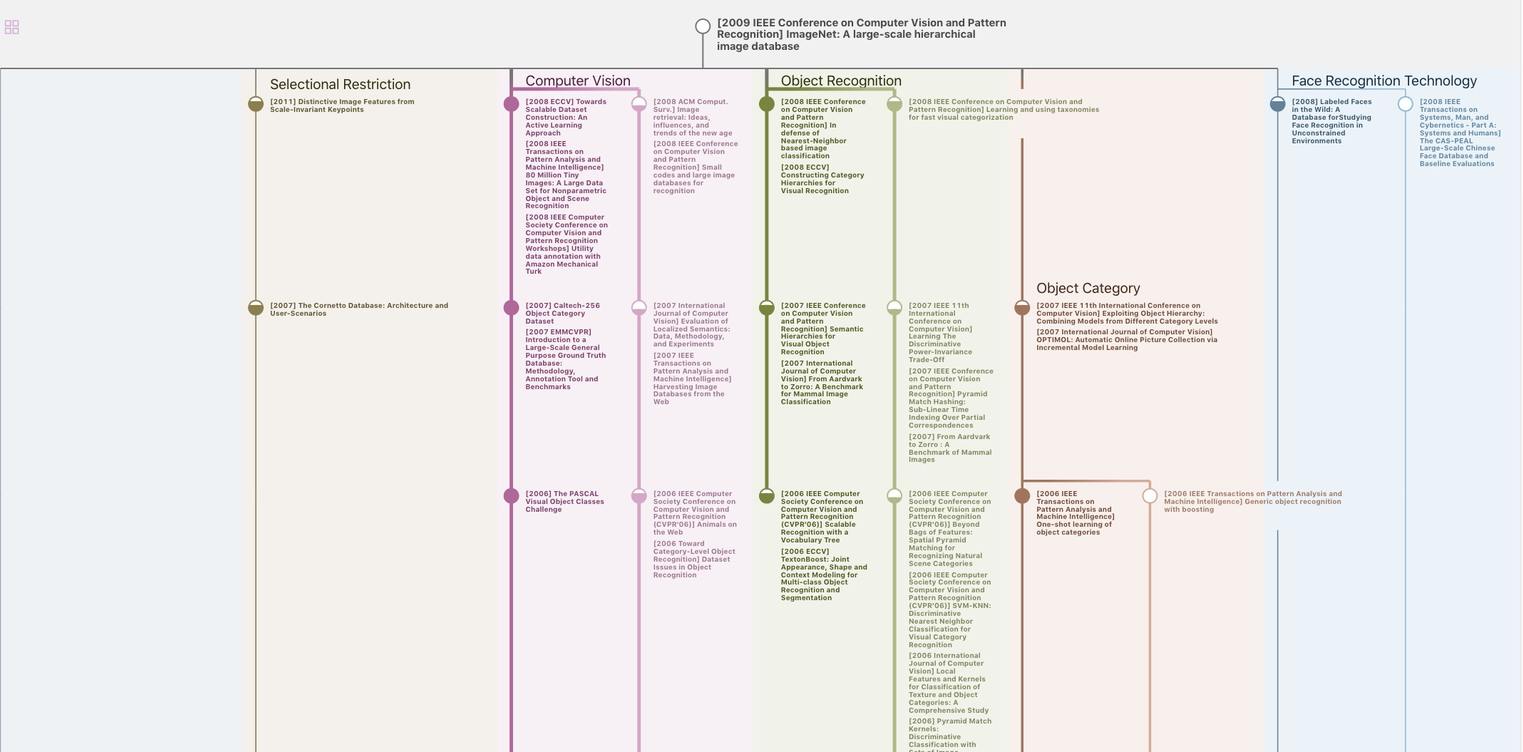
生成溯源树,研究论文发展脉络
Chat Paper
正在生成论文摘要