Leveraging uncertainty estimation and spatial pyramid pooling for extracting hydrological features from scanned historical topographic maps
GISCIENCE & REMOTE SENSING(2022)
摘要
Historical maps are almost the exclusive source to trace back the characteristics of earth before modern earth observation techniques came into being. Processing historical maps is challenging due to the factors such as diverse designs and scales, or inherent noise from painting, aging, and scanning. Our paper is the first to leverage uncertainty estimation under the framework of Bayesian deep learning to model noise inherent in maps for semantic segmentation of hydrological features from scanned topographic historical maps. To distinguish different features with similar symbolization, we integrate atrous spatial pyramid pooling (ASPP) to incorporate multi-scale contextual information. In total, our algorithm yields predictions with an average dice coefficient of 0.827, improving the performance of a simple U-Net by 26%. Our algorithm outputs intuitively interpretable pixel-wise uncertainty maps that capture uncertainty in object boundaries, noise from drawing, aging, and scanning, as well as out-of-distribution designs. We can use the predicted uncertainty potentially to refine segmentation results, locate rare designs, and select reliable features for future GIS analyses.
更多查看译文
关键词
Historical map processing,uncertainty estimation,semantic segmentation,hydrology,deep learning,gis
AI 理解论文
溯源树
样例
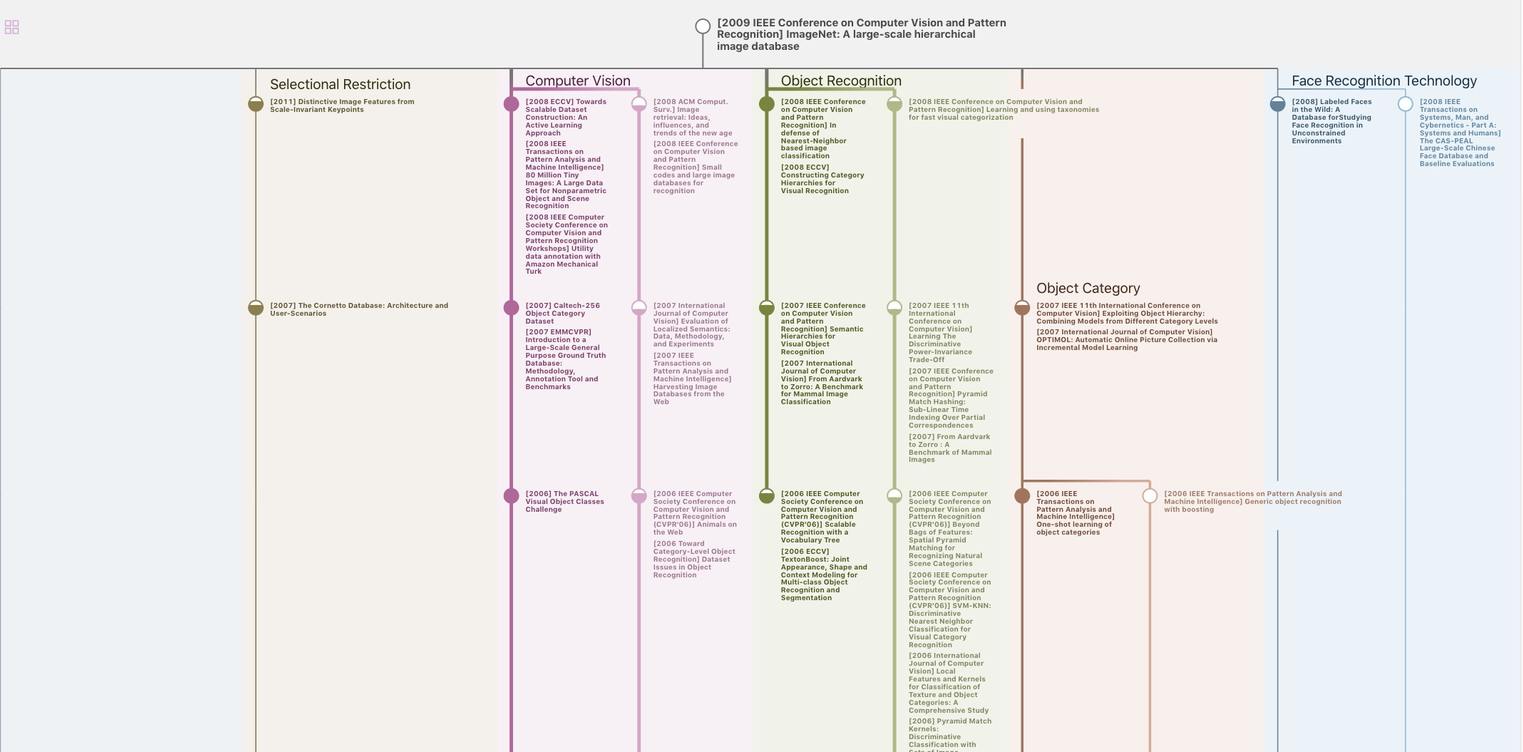
生成溯源树,研究论文发展脉络
Chat Paper
正在生成论文摘要