Multi-task Facial Activity Patterns Learning for micro-expression recognition using Joint Temporal Local Cube Binary Pattern
Signal Processing: Image Communication(2022)
摘要
As a spontaneous expression that cannot be suppressed, micro-expressions are a valuable clue to reveal the psychological reactions of humans. However, interpreting micro-expressions is a challenging task due to the interaction of these facial activities. The Facial Action Coding System explains the relationship between complex muscle movements and emotional states by defining action units that are both independent and interactive. Inspired by this study, the induction of relationships between action units and emotion categories can provide promising solutions for micro-expression recognition tasks. In this paper, a facial activity patterns learning-based micro-expression recognition method is proposed to explore the relationship between action units and emotional states. Specifically, the proposed method consists of two components, i.e., Joint Temporal Local Cube Binary Pattern (Joint Temporal LCBP) and Multi-task Facial Activity Patterns Learning framework. In the first step, Joint Temporal LCBP is proposed for encoding temporal structure and subtle changes. By applying data segmentation in the temporal dimension, the binary feature encodes variation among continuous facial activities, which describes the directional and intensity difference within the cubic data block. On this basis, a Multi-task Facial Activity Patterns Learning framework is designed to learn a set of the projection matrix to bridge the proposed descriptor and facial actions. In addition, the proposal framework further embeds class-level information into the mapping data space and selects a meaningful subset of features based on regularization techniques. Experimental results on three existing micro-expression datasets, i.e., CASME, CASME II, and SAMM, show that our model performs better than state-of-the-art methods.
更多查看译文
关键词
Micro-expression recognition,Joint temporal local cube binary pattern,Facial action unit,Facial activity patterns learning,Feature selection
AI 理解论文
溯源树
样例
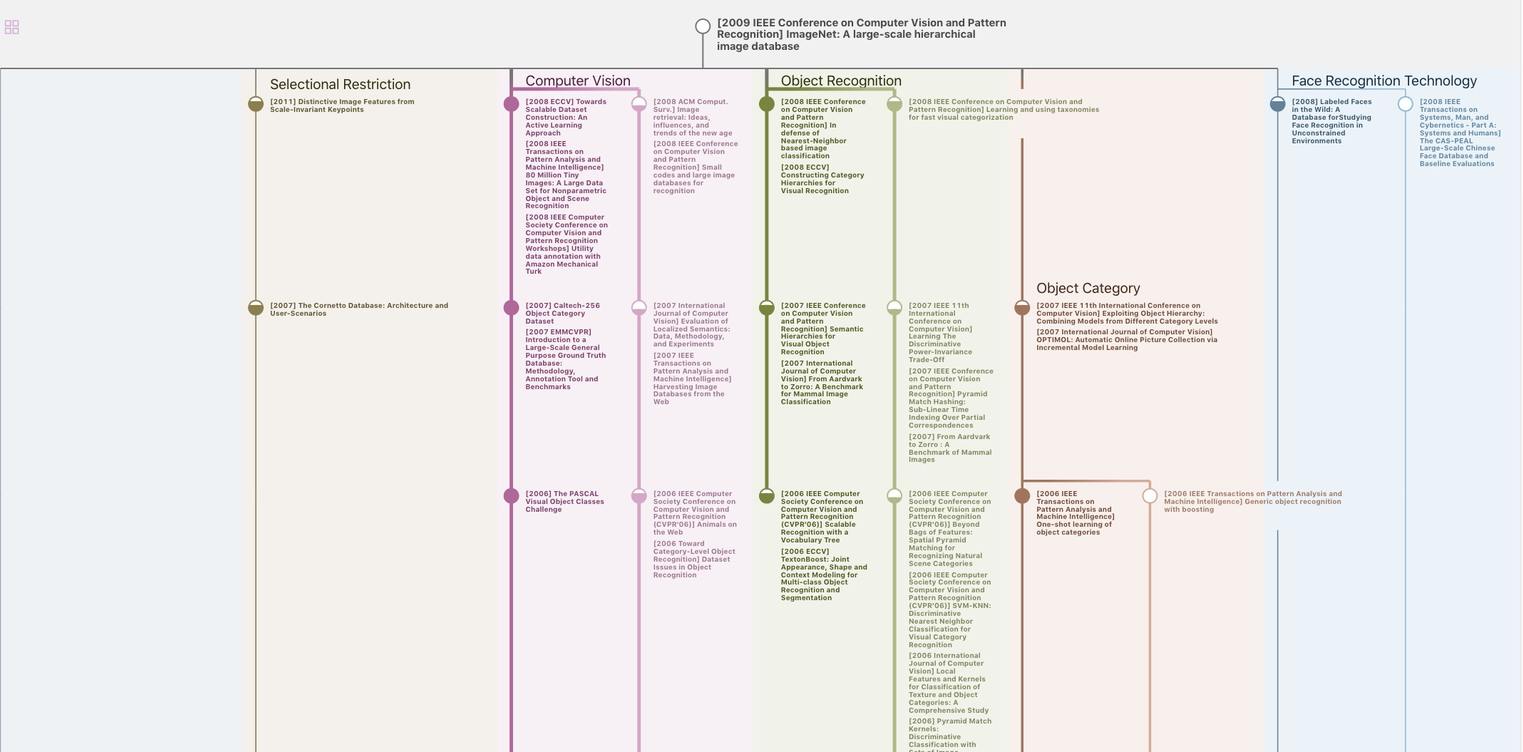
生成溯源树,研究论文发展脉络
Chat Paper
正在生成论文摘要