LTST: Long-term segmentation tracker with memory attention network
Image and Vision Computing(2022)
摘要
Recent interest in the combination of visual object tracking (VOT) and video object segmentation (VOS) has yielded rapid progress. However, existing segmentation methods are still restricted to the target model created in the first frame, which leads to the lack of long-term adaptability. To overcome this limitation, we propose a novel long-term segmentation tracker LTST leveraging memory attention network to achieve the effect of online learning without additional training. Specifically, we first combine a discriminative correlation filter with a matching-based paradigm for the segmentation task, then we develop a memory attention network based on partial cost volume to extract relevant historical information and dynamically reform the segmentation template. Moreover, we extend our LTST for long-term tracking by introducing a multi-scale verification network to identify tracking failures, and a global detector to re-locate the missing target. Experimental results on VOT-LT2018, VOT-LT2019, LaSOT and TLP benchmarks show that our proposed tracker achieves comparable performance to the state-of-the-art long-term tracking algorithms.(c) 2022 Elsevier B.V. All rights reserved.
更多查看译文
关键词
Long-term tracking,Object segmentation,Memory network,Attention mechanism
AI 理解论文
溯源树
样例
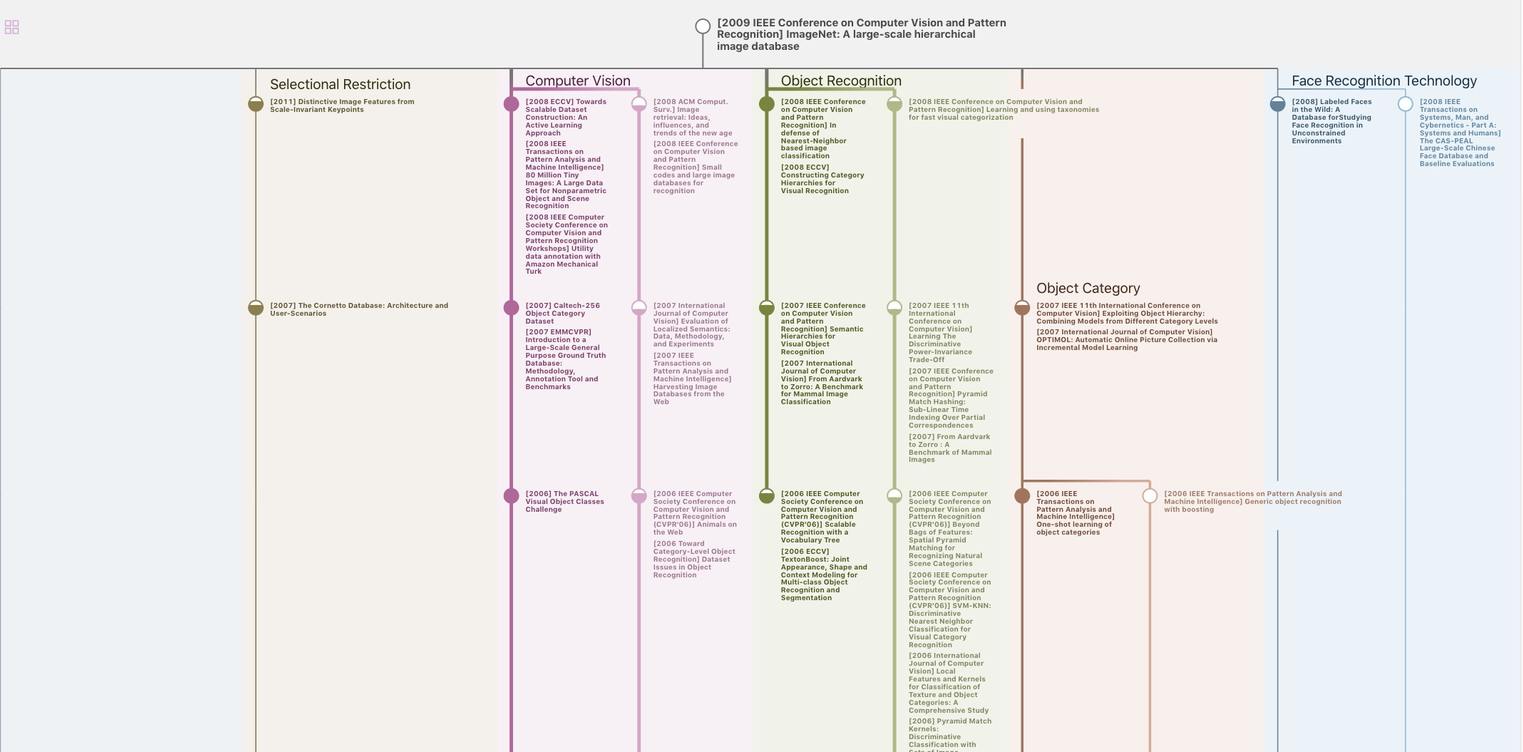
生成溯源树,研究论文发展脉络
Chat Paper
正在生成论文摘要