SIFTER: A Unified Framework for Robust Rumor Detection
IEEE/ACM transactions on audio, speech, and language processing(2022)
摘要
With the development of online social media, the fabrication and dissemination of rumors are much easier than before. As a result, automatic rumor detection becomes more urgent for internet governors, and has received great interest from the AI community. Even though many initial successes have been achieved in terms of detection accuracy and timeliness, existing rumor detection methods are still not robust due to the frequent domain shift problem and the inconsistent propagation problem. Frequent domain shift refers that rumors’ topic changes frequently on online social media, so the trained model may expire quickly in real applications. Inconsistent propagation is another practical challenge, referring to the fact that the spread of rumors is often accompanied by misleading comments. Therefore, a vulnerable model may output inconsistent predictions for the same instance. In this paper, we propose a new framework to improve the robustness of existing rumor detection methods, named SIFTER ( S ubjective I n F orma T ion E nhanced R einforcement learning). To address the frequent domain shift , SIFTER employs multi-task learning to introduce external knowledge that can explicitly describe rumors, and thus makes models more adaptive across domains. In particular, SIFTER involves a multi-task learning module to capture subjective information, which is found to be discriminative in rumors’ propagations. To address the inconsistent propagation , SIFTER employs reinforcement learning to realize a new training schema tailed for rumor detection, namely sequential training, which can reduce the attendance of noisy comments in the feature extraction process. Experimental results on two benchmark datasets, Twitter and Weibo, validate the effectiveness of SIFTER. After migrating existing methods to SIFTER, their accuracy score is improved up to 9.4% in cross-domain inference, and their reverse ratio decreases by nearly 4% in continuous prediction.
更多查看译文
AI 理解论文
溯源树
样例
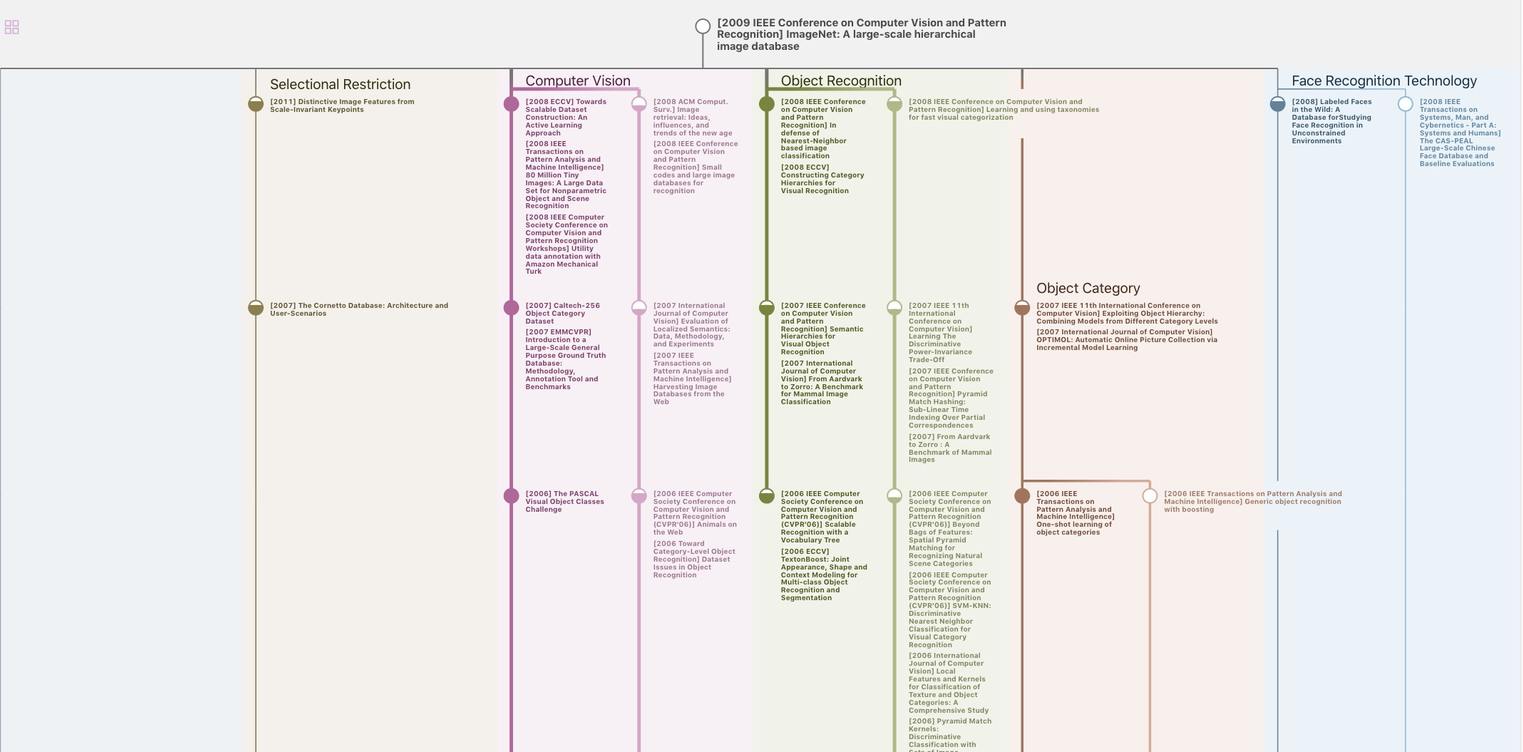
生成溯源树,研究论文发展脉络
Chat Paper
正在生成论文摘要