Offline reinforcement learning with anderson acceleration for robotic tasks
Applied Intelligence(2022)
摘要
Offline reinforcement learning (RL) can learn effective policy from a fixed batch of data without interaction. However, the real-world requirements, such as better performance and high sample efficiency, put substantial challenges on current offline RL algorithms. In this paper, we propose a novel offline RL method, Constrained and Conservative Reinforcement Learning with Anderson Acceleration (CCRL-AA), which aims to enable the agent to effectively and efficiently learn from offline demonstration data. In our method, Constrained and Conservative Reinforcement Learning (CCRL) restricts the policy’s actions with respect to a batch of training data and learns a conservative Q-function to make the agent effectively learn from the previously collected demonstrations. The mechanism of Anderson acceleration (AA) is integrated to speed up the learning process and improve sample efficiency. Experiments were conducted on robotic simulation tasks, and the results demonstrate that our method can efficiently learn from given demonstrations and give better performance than several other state-of-the-art methods.
更多查看译文
关键词
Offline reinforcement learning,Robot learning,Demonstrations,Constrained and conservative reinforcement learning,Anderson acceleration
AI 理解论文
溯源树
样例
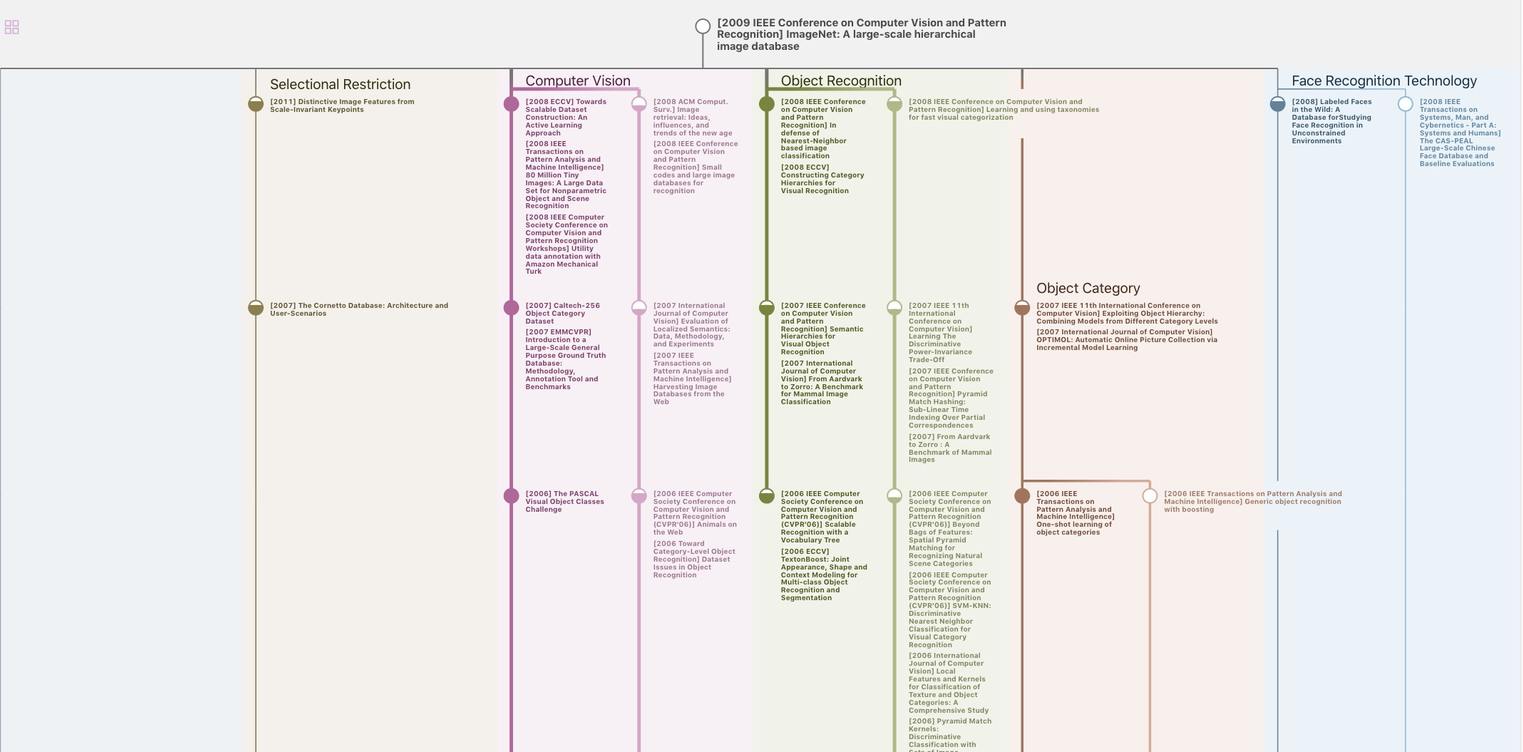
生成溯源树,研究论文发展脉络
Chat Paper
正在生成论文摘要