A heuristic approach for multiple instance learning by linear separation
Soft Computing(2022)
摘要
We present a fast heuristic approach for solving a binary multiple instance learning (MIL) problem, which consists in discriminating between two kinds of item sets: the sets are called bags and the items inside them are called instances. Assuming that only two classes of instances are allowed, a common standard hypothesis states that a bag is positive if it contains at least a positive instance and it is negative when all its instances are negative. Our approach constructs a MIL separating hyperplane by preliminary fixing the normal and reducing the learning phase to a univariate nonsmooth optimization problem, which can be quickly solved by simply exploring the kink points. Numerical results are presented on a set of test problems drawn from the literature.
更多查看译文
关键词
Multiple instance learning (MIL),Linear separation,Nonsmooth optimization
AI 理解论文
溯源树
样例
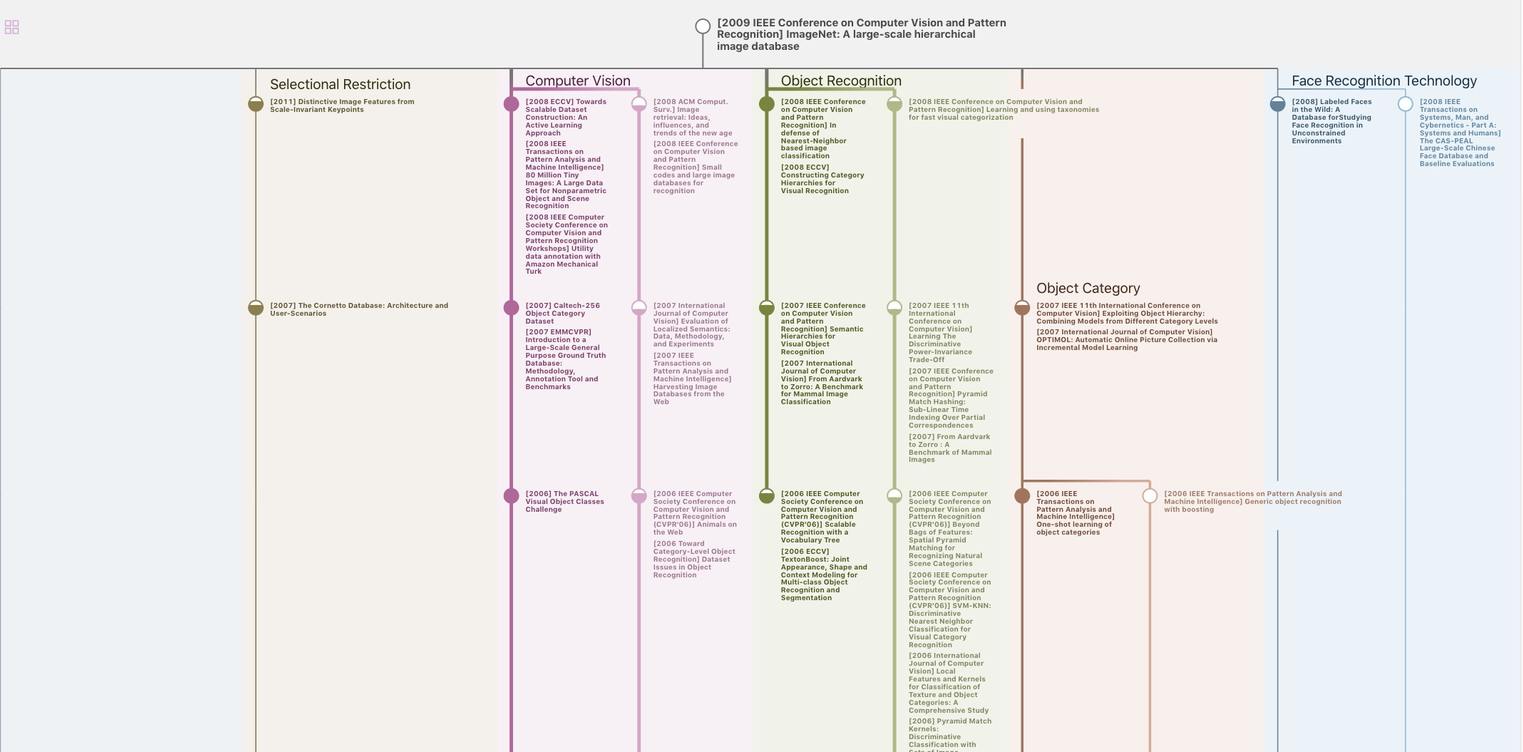
生成溯源树,研究论文发展脉络
Chat Paper
正在生成论文摘要