Federated Meta-Learning Enhanced Acoustic Radio Cooperative Framework for Ocean of Things
IEEE Journal of Selected Topics in Signal Processing(2022)
摘要
Ocean of Things, consisting of multiple buoys distributed on the sea, is an acoustic radio cooperative wireless network that aims to acquire underwater information. In this paper, a deep neural network (DNN)-based receiver with data augmentation, termed chirp (C)-DNN, is developed for a buoy that uses chirp modulation-based underwater acoustic communications. To further solve the problem that the training data at a single buoy may not be sufficient, a federated meta-learning (FML) scheme is proposed to train the DNN-based receiver by exploiting the model parameters from multiple buoys. We analyze the convergence performance of FML and derive a closed-form expression for the convergence rate, accounting for the impacts of scheduling ratios, local epochs, and data volumes on a single node. Simulation results show that the proposed C-DNN receiver that is trained with sufficient data achieves better bit error rate performance and lower complexity than classical matched filter (MF)-based detectors. The proposed FML also outperforms the MF-based method after several communication rounds and achieves better generalization performance than federated learning based systems.
更多查看译文
关键词
Federated meta-learning,underwater acoustic communications,chirp communications,distributed systems,convergence
AI 理解论文
溯源树
样例
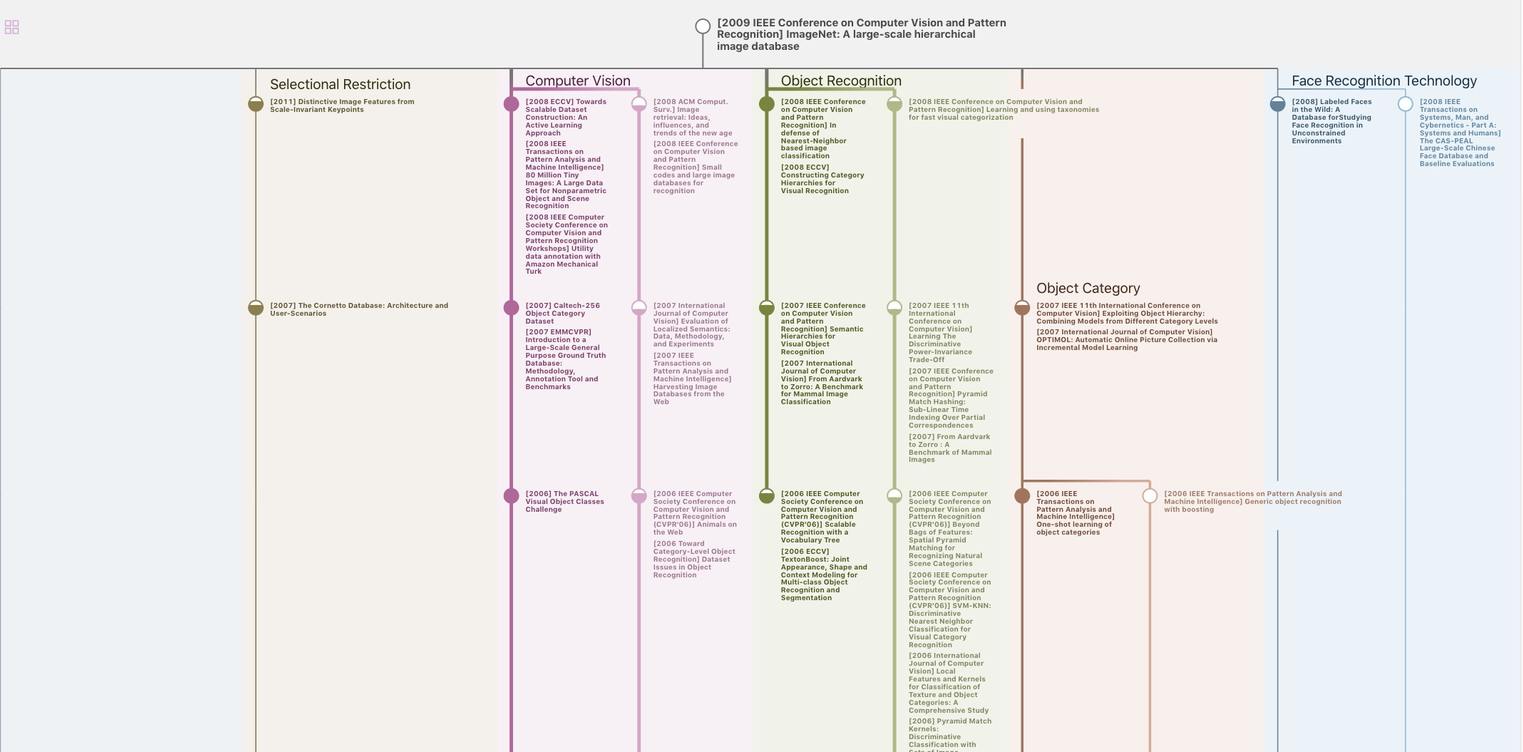
生成溯源树,研究论文发展脉络
Chat Paper
正在生成论文摘要