Deep representation learning for face hallucination
Multimedia Tools and Applications(2022)
摘要
Recently, deep learning, as a novel emerging algorithm, offers an end-to-end effective paradigm for super-resolution. Various successful practices with the deep learning model have confirmed the truth that deeper features always bring better performance. In this paper, we present a novel deep representation learning framework for face hallucination to verify the “coarse-to-fine” nature of deep features. The proposed framework includes the optimization of deep representation coefficients and the updating of deep dictionary learning. First, local and nonlocal patches are used to enrich the self-similarity prior to local to global optimization. Then a unified regularization term is added into the representation objective function to fully exploit accurate prior. Deeply coupled multi-layer dictionaries are developed to support the deep representation scheme as refining the high-resolution image from coarse to fine layer-by-layer. Finally, residual recursive learning is combined into a deep representation framework for boosting the reconstruction performances. Different from neural network’s deep feature learning manner, the proposed method provides a novel explanation of how deep representation works. Extensive experiments are conducted on FEI, CAS-PEAL-R1, and LFW databases to testify its subjective and objective performance. Experimental results demonstrate that the proposed approach outperforms some state-of-the-art face hallucination methods, including the method based on convolution neural network and the method based on vanilla representation.
更多查看译文
关键词
Deep representation,Deep dictionary updating,Residual recursive learning,Local and nonlocal patches
AI 理解论文
溯源树
样例
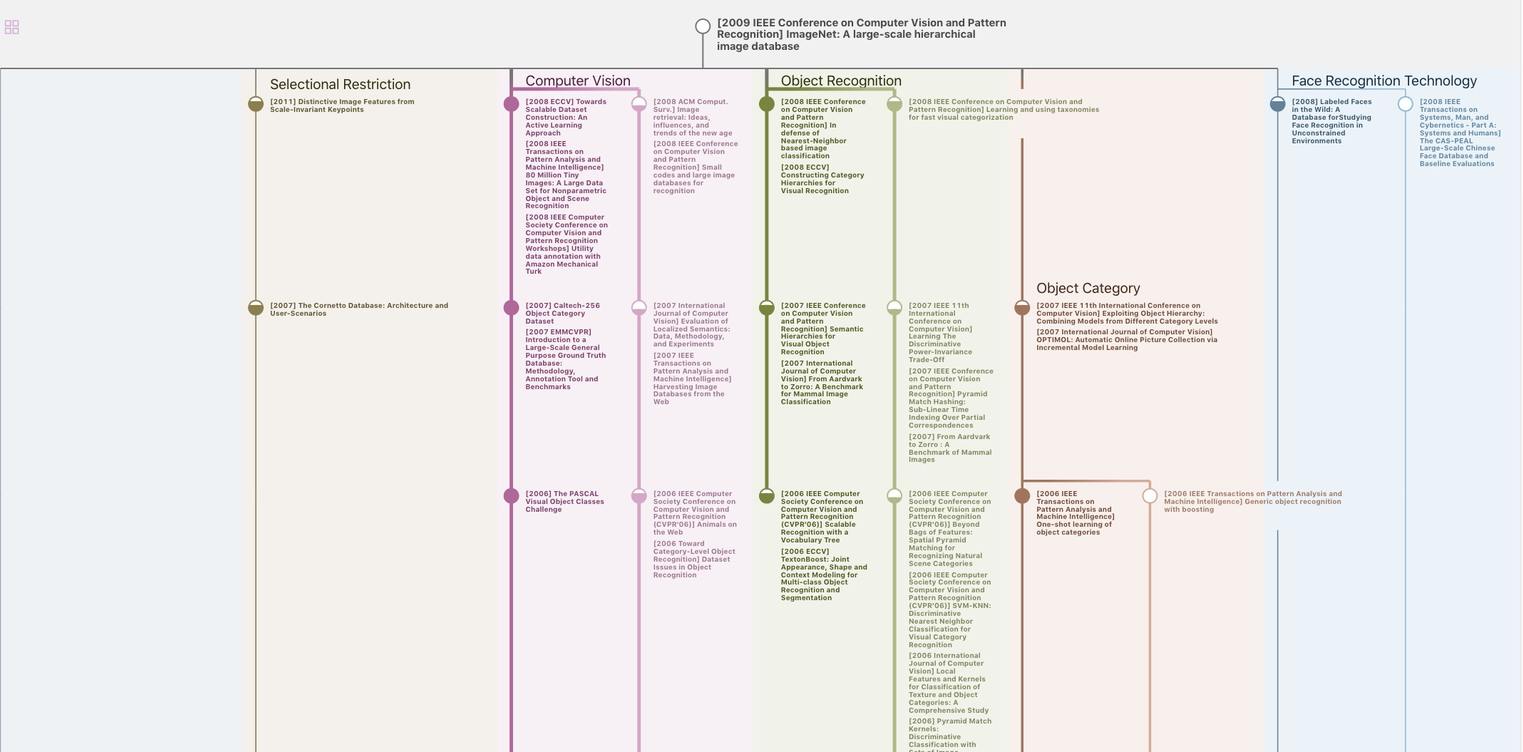
生成溯源树,研究论文发展脉络
Chat Paper
正在生成论文摘要