Machine Comprehension Comparison using Latest Advancements in Deep Learning
2021 IEEE 18th India Council International Conference (INDICON)(2021)
摘要
Machine Comprehension or Question Answering (QA) is one of the most challenging natural language processing tasks due to the language’s dynamic nature and understanding the context of the question. In this paper, we propose a similarity attention layer with an aim to reduce human labor by automating tedious QA tasks using the attention mechanism in deep learning model; it uses attention scores and obtains good results even without pre-training. The QA using attention has immense scope in search engine optimization, page ranking, and chatbots. The traditional rule-based models and statistical methods underperform due to variations in the language. This dynamic nature of the language is well captured by the nonlinear learning of the neural networks. The conventional encoder-decoder architecture of neural networks for QA works well in the case of short sentences. However, the performance comes down for paragraphs and very long sentences as it is difficult for the network to memorize the super-long sentences. In contrast, the attention model helps the network focus on smaller attention areas in the complex input paragraph, part by part, until the entire text is processed. The results are very promising; our (single) model outperforms the existing ensemble method too.
更多查看译文
关键词
Machine Comprehension,Question Answering,Deep Learning,Attention models
AI 理解论文
溯源树
样例
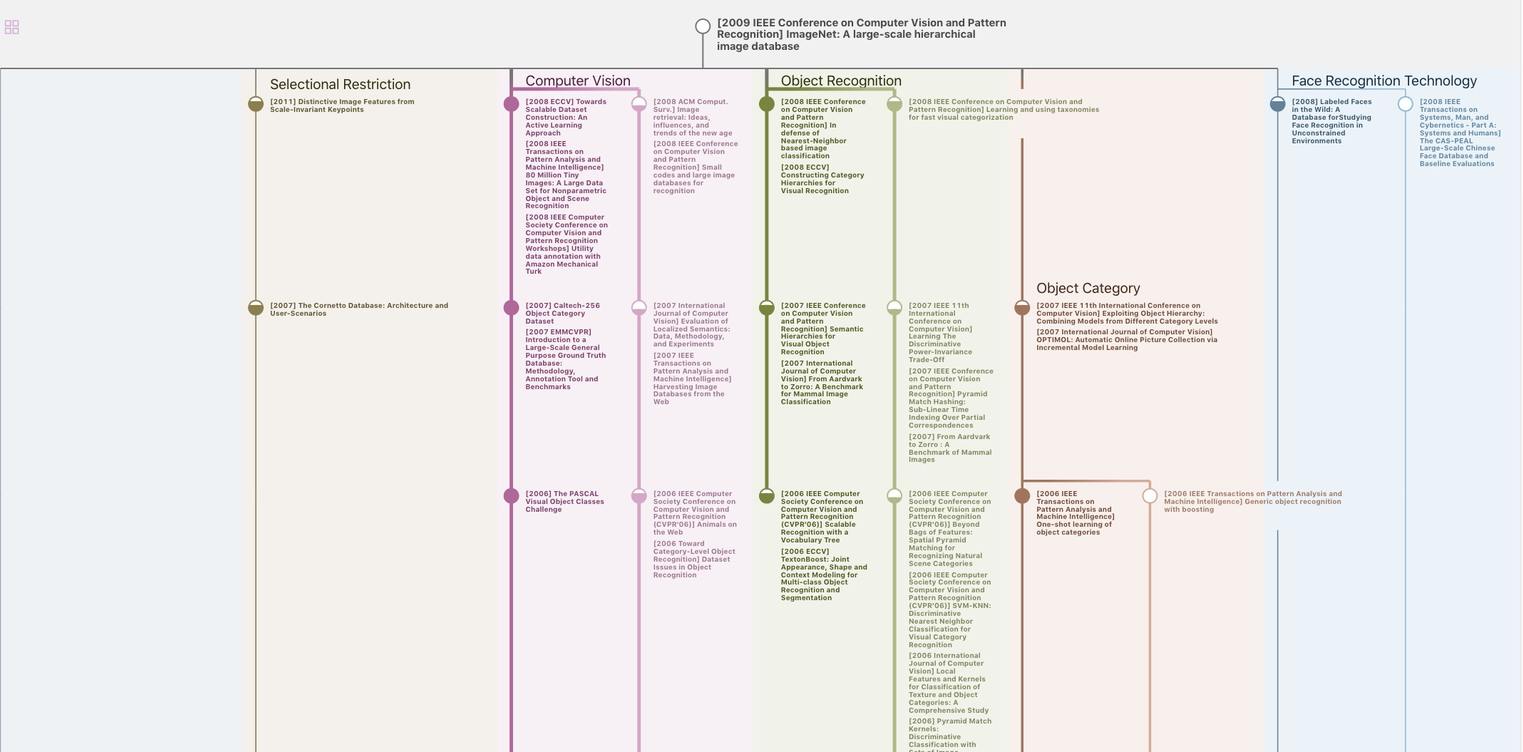
生成溯源树,研究论文发展脉络
Chat Paper
正在生成论文摘要