Enhancing COVID-19 prediction using transfer learning from Chest X-ray images
2021 8th NAFOSTED Conference on Information and Computer Science (NICS)(2021)
摘要
The pandemic of COVID-19 is expansion and effect for human lives all over the world. Although many countries have been vaccinated, the number of new COVID-19 patients infected is still increasing. Recently, the detection of COVID-19 early can help find effective treatment plans using machine learning technologies algorithms. We propose the transfer learning models to detect pneumonia disease by this virus from chest X-Ray images. The public dataset is used in this work, and the new chest X-Ray images of COVID-19 patients are collected by An Giang Regional General Hospital. These images enrich the current public dataset and improve the performance prediction. Six transfer learning architectures are investigated using locally collected and public dataset. The experiment results show that the DenseNet121 transfer learning model outperforms others with the accuracy, precision, recall, F1-scores, and AUC of 98.51%, 98.54%, 98.51%, 98.05% and 99.15%, respectively on the augmented dataset and most algorithms process new data are improved performance.
更多查看译文
关键词
COVID-19,transfer learning,imbalanced dataset,X-Ray images
AI 理解论文
溯源树
样例
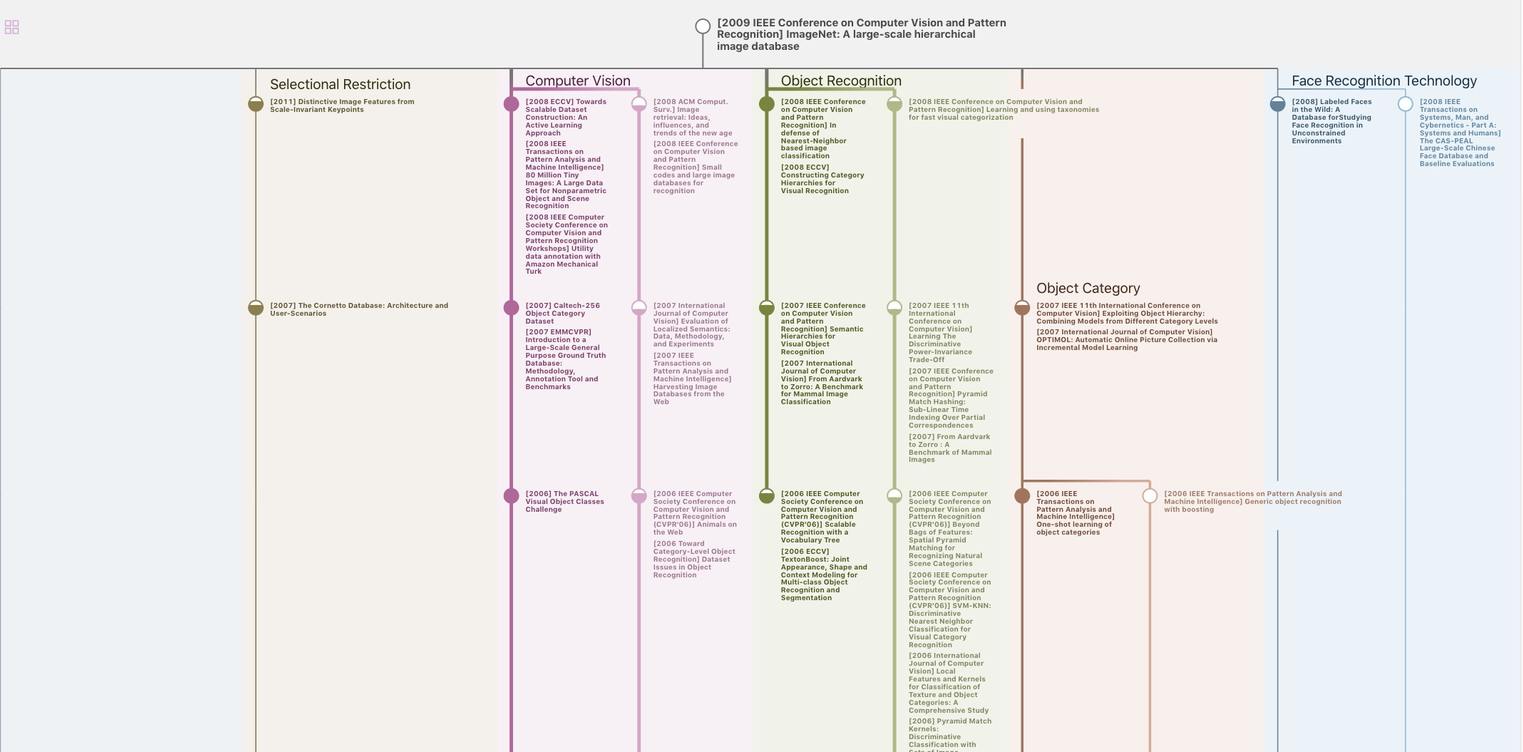
生成溯源树,研究论文发展脉络
Chat Paper
正在生成论文摘要