Identification of tumor homing peptides by utilizing hybrid feature representation.
Journal of biomolecular structure & dynamics(2022)
摘要
Cancer is one of the serious diseases, recent studies reported that tumor homing peptides (THPs) play a key role in treatment of cancer. Due to the experimental methods are time-consuming and expensive, it is urgent to develop automatic computational approaches to identify THPs. Hence, in this study, we proposed a novel machine learning methods to distinguish THPs from non-THPs, in which the peptide sequences firstly encoded by pseudo residue pairwise energy content matrix (PseRECM) and pseudo physicochemical property (PsePC). Moreover, the least absolute shrinkage and selection operator (LAASO) was employed to select optimal features from the extracted features. All of these selected features were fed into support vector machine (SVM) for identifying THPs. We achieved 89.02%, 88.49%, and 94.58% classification accuracy on the Main, Small, and Main90 dataset, respectively. Experimental results showed that our proposed method outperforms the existing predictors on the same benchmark datasets. It indicates that the proposed method may be a useful tool in identifying THPs. The datasets and codes used in current study are available at https://figshare.com/articles/online_resource/iTHPs/16778770.Communicated by Ramaswamy H. Sarma.
更多查看译文
关键词
LASSO,PsePC,PseRECM,Tumor homing peptides,support vector machine
AI 理解论文
溯源树
样例
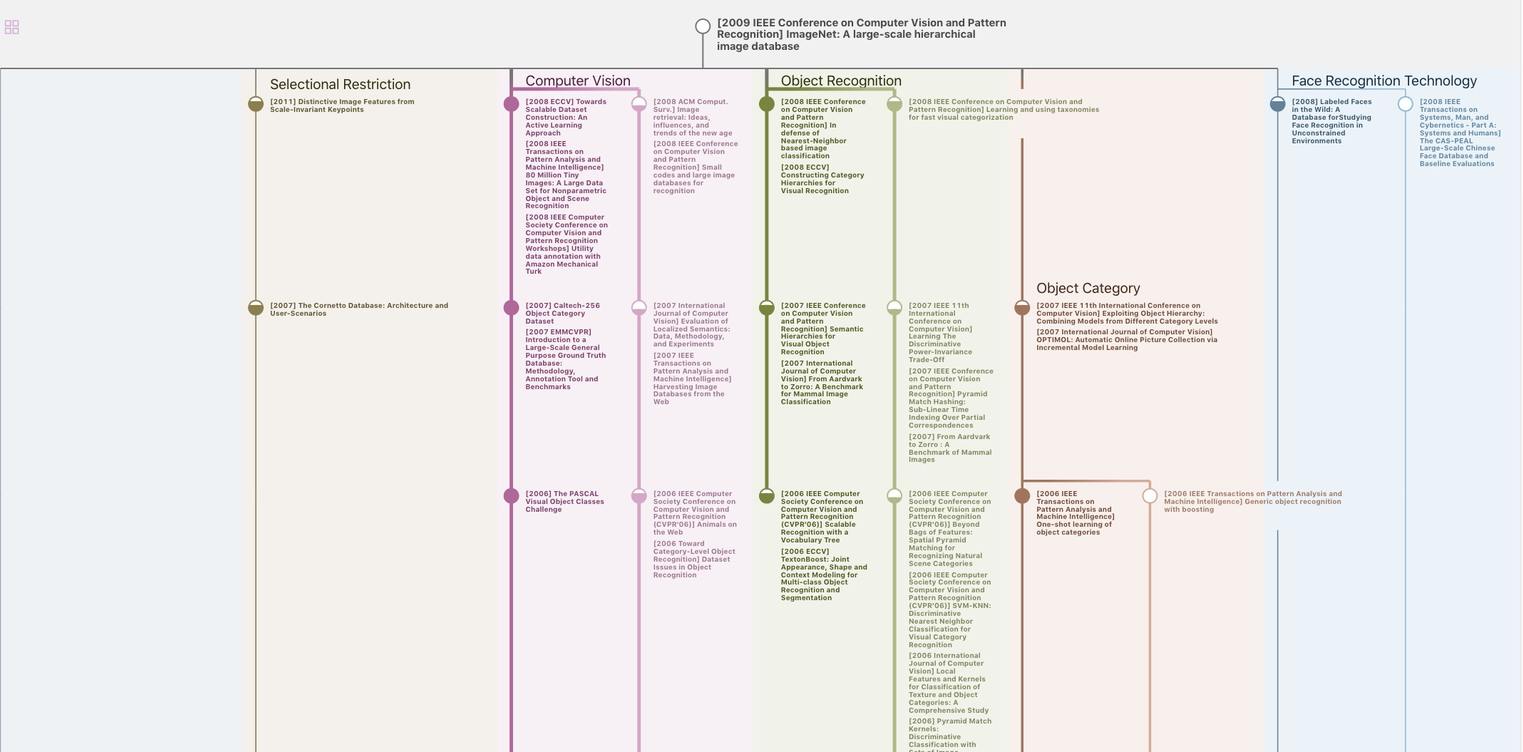
生成溯源树,研究论文发展脉络
Chat Paper
正在生成论文摘要