An Improved Neural Network Based on SENet for Sleep Stage Classification
IEEE Journal of Biomedical and Health Informatics(2022)
摘要
Sleep staging is an important step in analyzing sleep quality. Traditional manual analysis by psychologists is time-consuming. In this paper, we propose an automatic sleep staging model with an improved attention module and hidden Markov model (HMM). The model is driven by single-channel electroencephalogram (EEG) data. It automatically extracts features through two convolution kernels with different scales. Subsequently, an improved attention module based on Squeeze-and-Excitation Networks (SENet) will perform feature fusion. The neural network will give a preliminary sleep stage based on the learned features. Finally, an HMM will apply sleep transition rules to refine the classification. The proposed method is tested on the sleep-EDFx dataset and achieves excellent performance. The accuracy on the Fpz-Cz channel is 84.6%, and the kappa coefficient is 0.79. For the Pz-Oz channel, the accuracy is 82.3% and kappa is 0.76. The experimental results show that the attention mechanism plays a positive role in feature fusion. And our improved attention module improves the classification performance. In addition, applying sleep transition rules through HMM helps to improve performance, especially N1, which is difficult to identify.
更多查看译文
关键词
Electroencephalogram,sleep staging,convolutional neural network,attention mechanism,hidden Markov model
AI 理解论文
溯源树
样例
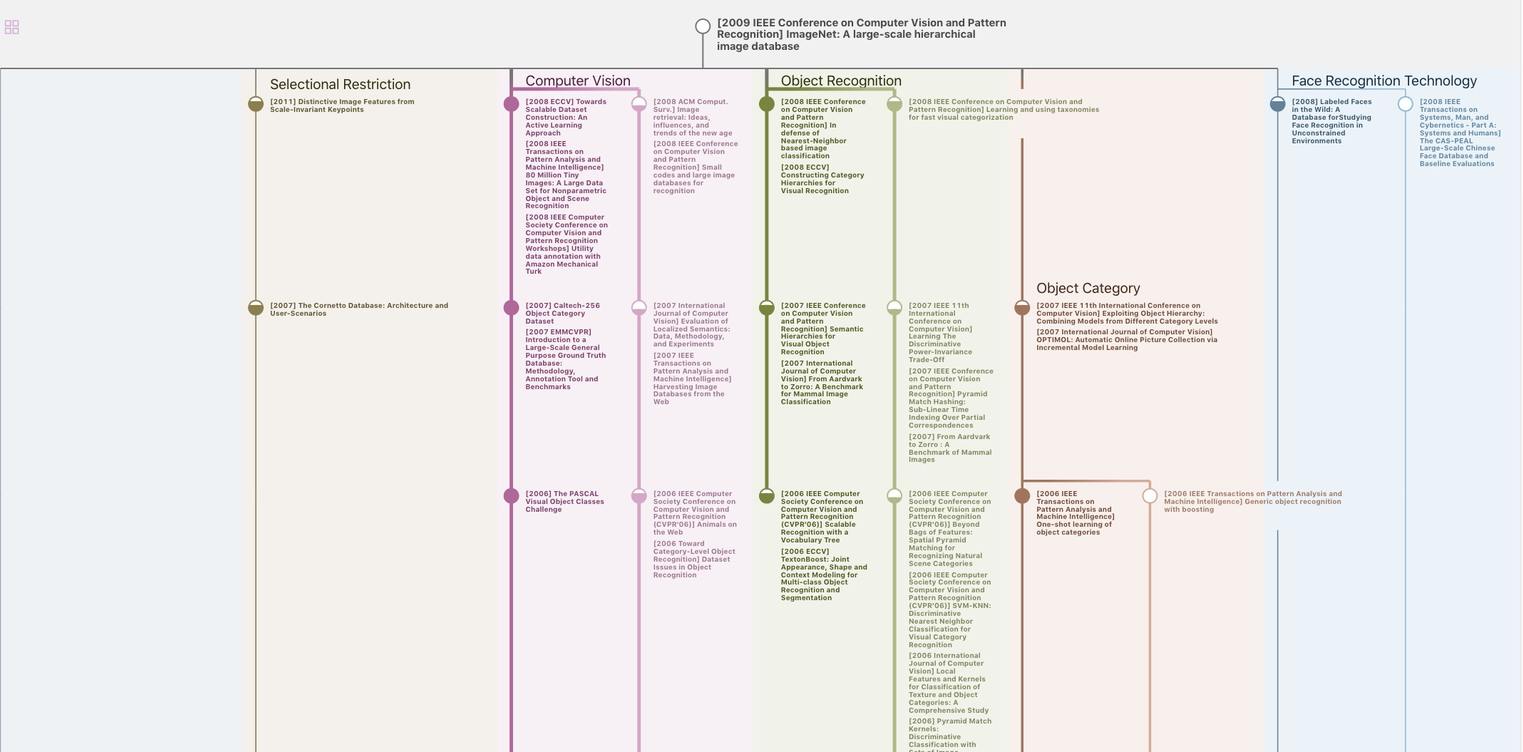
生成溯源树,研究论文发展脉络
Chat Paper
正在生成论文摘要