Model-Agnostic Temporal Regularizer for Object Localization Using Motion Fields
IEEE TRANSACTIONS ON IMAGE PROCESSING(2022)
摘要
Video analysis often requires locating and tracking target objects. In some applications, the localization system has access to the full video, which allows fine-grain motion information to be estimated. This paper proposes capturing this information through motion fields and using it to improve the localization results. The learned motion fields act as a model-agnostic temporal regularizer that can be used with any localization system based on keypoints. Unlike optical flow-based strategies, our motion fields are estimated from the model domain, based on the trajectories described by the object keypoints. Therefore, they are not affected by poor imaging conditions. The benefits of the proposed strategy are shown on three applications: 1) segmentation of cardiac magnetic resonance; 2) facial model alignment; and 3) vehicle tracking. In each case, combining popular localization methods with the proposed regularizer leads to improvement in overall accuracies and reduces gross errors.
更多查看译文
关键词
Location awareness, Tracking, Trajectory, Predictive models, Computational modeling, Estimation, Motion segmentation, Object localization, motion fields, temporal regularization
AI 理解论文
溯源树
样例
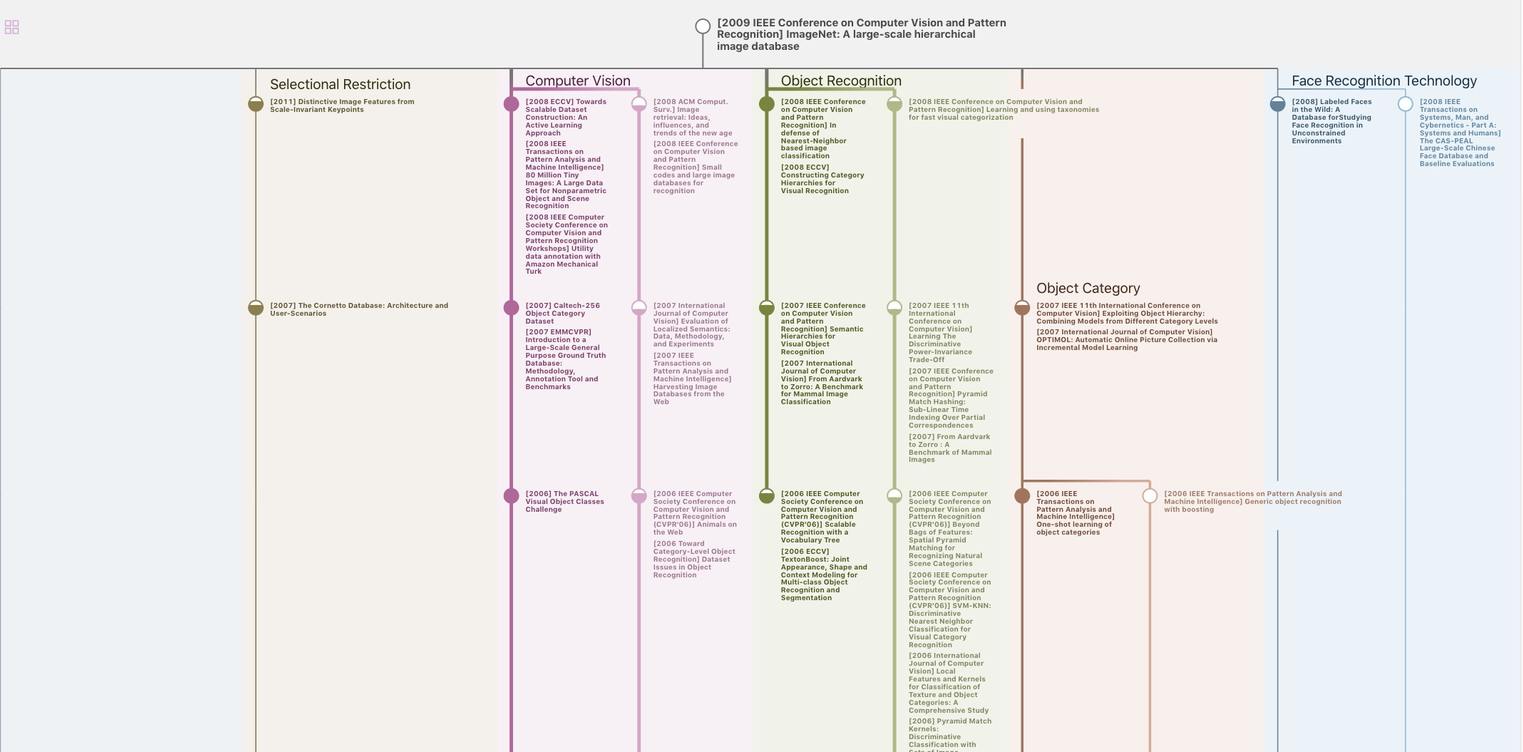
生成溯源树,研究论文发展脉络
Chat Paper
正在生成论文摘要