Learning Algorithms for Price Control in an Internet-Based Dutch Auction
Social Science Research Network(2001)
摘要
In this paper, we consider a multi-unit Dutch auction over the Internet where auctioneer gradually decrements per unit price of the item during the course of the auction. We investigate an optimal price control problem of the auctioneer, that is, the problem of finding a decrementing price sequence that maximizes his total expected revenue in the presence of uncertainty with regard to arrival pattern of bidders and their individual price-demand curves.We start with an analysis of an analogous multi-unit pay-your-bid auction in a discrete setting and provide a characterization of mixed strategy equilibrium. Since it is difficult to arrive at a pure strategy equilibrium, we deviate from game theoretic consideration and model the above decision problem in a Dutch auction as a single-agent Reinforcement Learning in an uncertain non-stationary auction environment wherein the auctioneer (or his agent) uses its experience interacting with the environment to improve his (its) pricing strategies.Over the Internet, auctioneer always has an option of concealing information pertaining to dynamics of the ongoing Dutch auction from bidders. In this situation, it can be assumed that each bidder values the items independently of other bidders. For this case we develop a finite horizon Markov Decision Process (MDP) model with undiscounted returns and propose a Qlearning algorithm for generating a decrementing price sequence for optimal revenue.In a more general setting where bidders can observe the ongoing auction, the state-space representation will lead to the domain appearing non-Markov to the reinforcement learning agent. In a particular case where history provides the needed missing state information, we investigate the applicability of direct reinforcement learning and contrast the temporal difference based RL with actual return based RL. We show that direct application of temporal difference based reinforcement learning algorithms will in general fail to learn even deterministic optimal policies in a Dutch auction environment. Furthermore, our analysis suggests that actual return based reinforcement learning algorithms be used instead.
更多查看译文
AI 理解论文
溯源树
样例
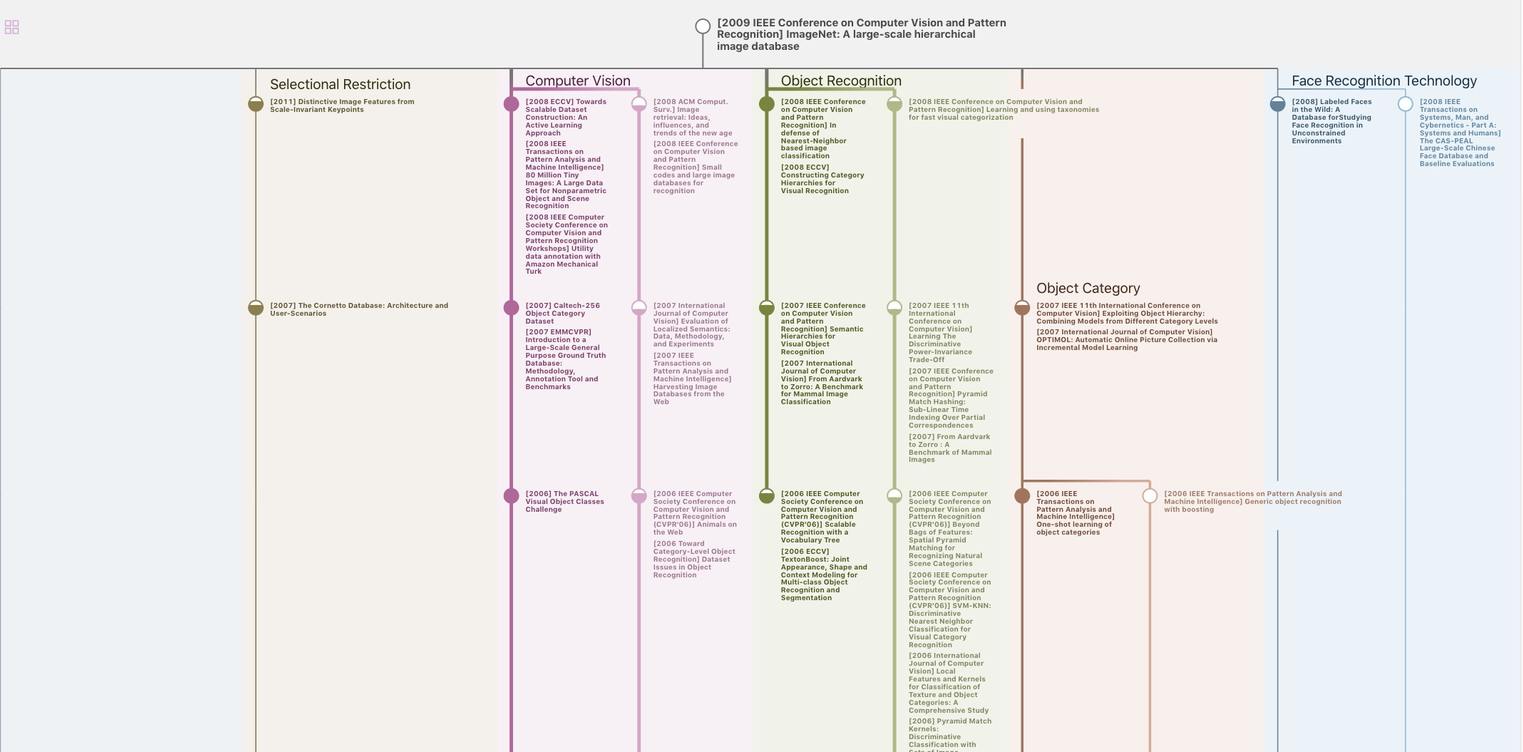
生成溯源树,研究论文发展脉络
Chat Paper
正在生成论文摘要