Feature Weighting for Improved Classifier Robustness
conference on email and anti spam(2009)
摘要
There are often discrepancies between the learning sample and the evaluation environment, be it natural or adversarial. It is therefore desirable that classifiers are robust, i.e., not very sensitive to changes in data distribution. In this paper, we introduce a new methodology to measure the lower bound of classifier robustness under adversarial attack and show that simple averaged classifiers can improve classifier robustness significantly. In addition, we propose a new feature reweighting technique that ameliorates the performance and robustness of standard classifiers at at most twice the computational cost. We verify our claims in content based email spam classification experiments on some public and private datasets.
更多查看译文
AI 理解论文
溯源树
样例
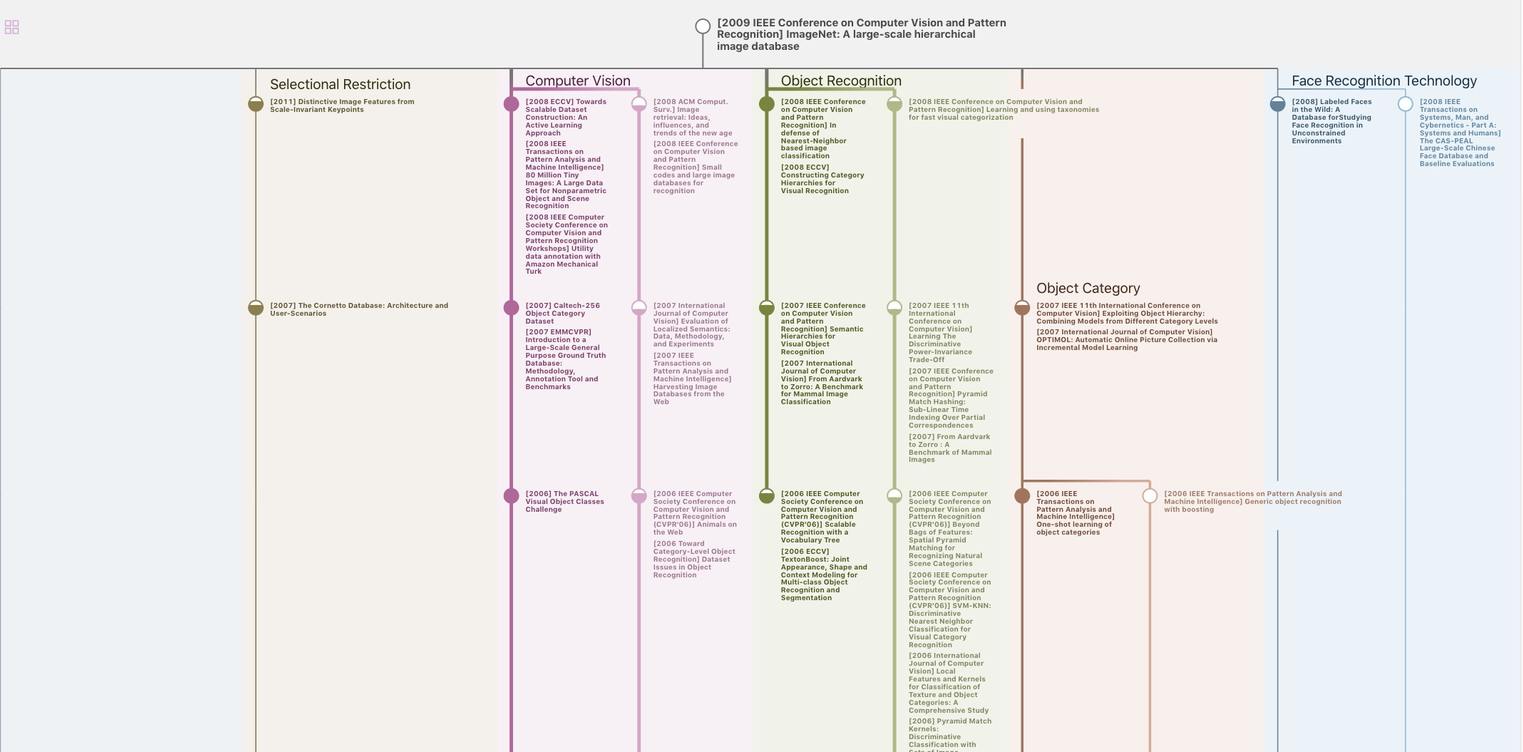
生成溯源树,研究论文发展脉络
Chat Paper
正在生成论文摘要