One-Shot Learning with Memory-Augmented Neural Networks Using a 64-kbit, 118 GOPS/W RRAM-Based Non-Volatile Associative Memory
2021 Symposium on VLSI Technology(2021)
摘要
Learning from a few examples (one/few-shot learning) on the fly is a key challenge for on-device machine intelligence. We present the first chip-level demonstration of one-shot learning using a 2T-2R resistive RAM (RRAM) non-volatile associative memory (AM) as the backend of memory-augmented neural networks (MANNs). The 64-kbit fully integrated RRAM-CMOS AM core (0.2 mm
2
at 40 nm node) enables long-term feature embedding and retrieval, demonstrated in a challenging 32-way one-shot learning task using Omniglot dataset. Using only one example per class for 32 unseen classes during on-chip learning, our AM chip achieves ~72% measured inference accuracy on Omniglot as the first chip accuracy report compared to software accuracy (~82%), while reaching 118 GOPS/W for in-memory L1 distance computation and prediction.
更多查看译文
AI 理解论文
溯源树
样例
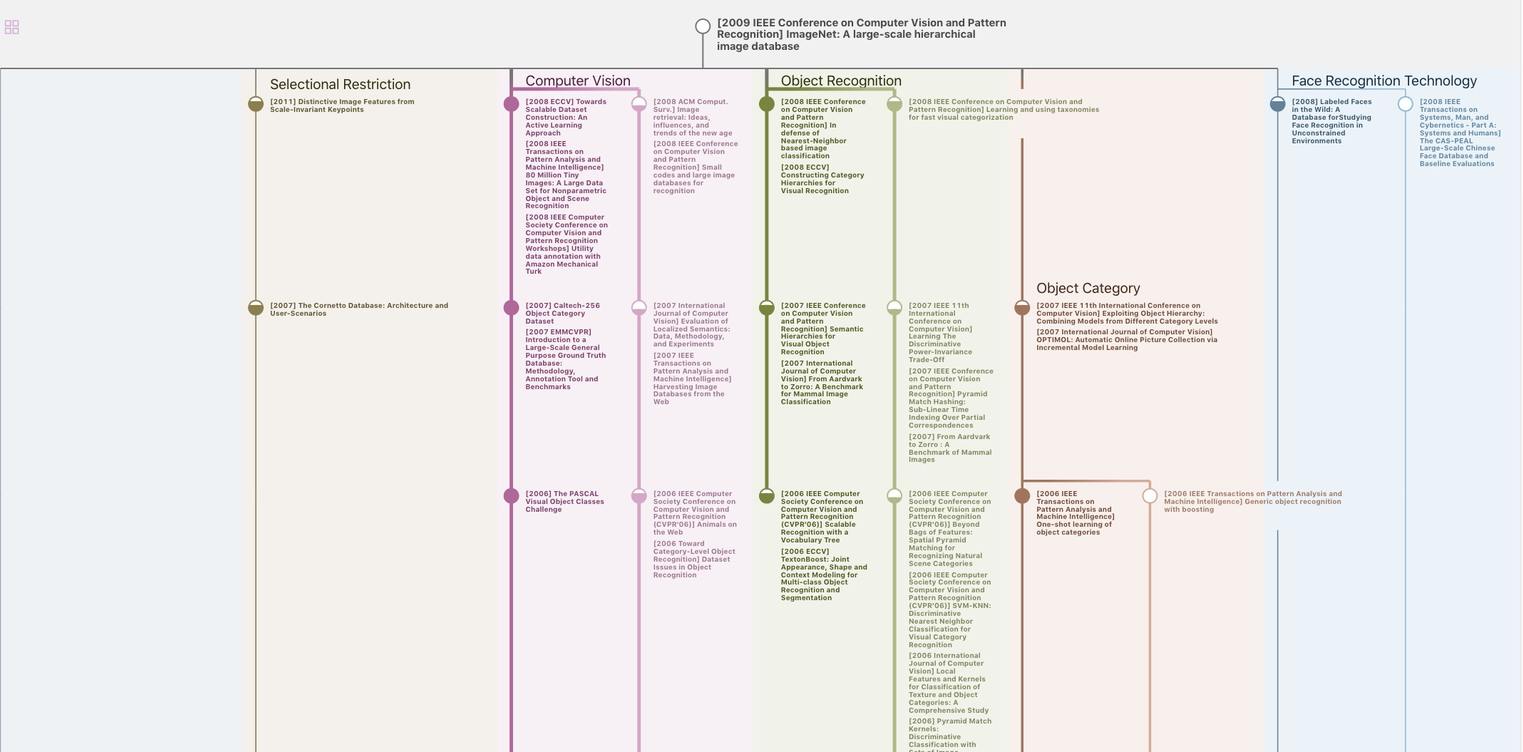
生成溯源树,研究论文发展脉络
Chat Paper
正在生成论文摘要