Simulation training for laparoscopic surgery with 3rd and 4th year medical students
Qatar Foundation Annual Research Forum Volume 2010 Issue 1(2010)
摘要
Abstract Medical education is characterized by apprenticeship reflected in ‘see one, do one, teach one’. This research investigated effects of practice and individual guidance by a mentor for laparoscopic surgery using a simulation engine. Based on earlier research, we expected that it would take extensive training for self-directed, experience-based learning to compensate for individual, on-time instruction that is typical for medical education. Three groups of 3rd and 4th medical students trained to perform laparoscopic colecystectmy in 5 training sessions of 30 or 60 min. The mentored group received one-to-one individual guidance by a mentor during the complete training. A time-matched control group received exclusively feedback from the simulation engine. An extensive practice group was allotted double the time to compensate for missing guidance. Before and after training, their performance was analyzed for the first case in the system. Mentored students performed better during the pretest than students from both control groups. After training those students performed as well without guidance as during the pretest with guidance. Participants from both control groups improved performance from pre-test to post-test. In addition, students with extensive training performed almost as well as mentored students during the post-test. This implies that feedback provided by the simulation system is good enough for unsupervised students to reach a performance level comparable to mentored students, but it requires double the time for training. Next, we aim to improve the system's feedback to dramatically reducing training time while reaching the same level of performance. For example, this means to provide in-time warning before committing an error instead of presenting an error message after committing it. This will prevent students from automatizing suboptimal or dangerous routines.
更多查看译文
AI 理解论文
溯源树
样例
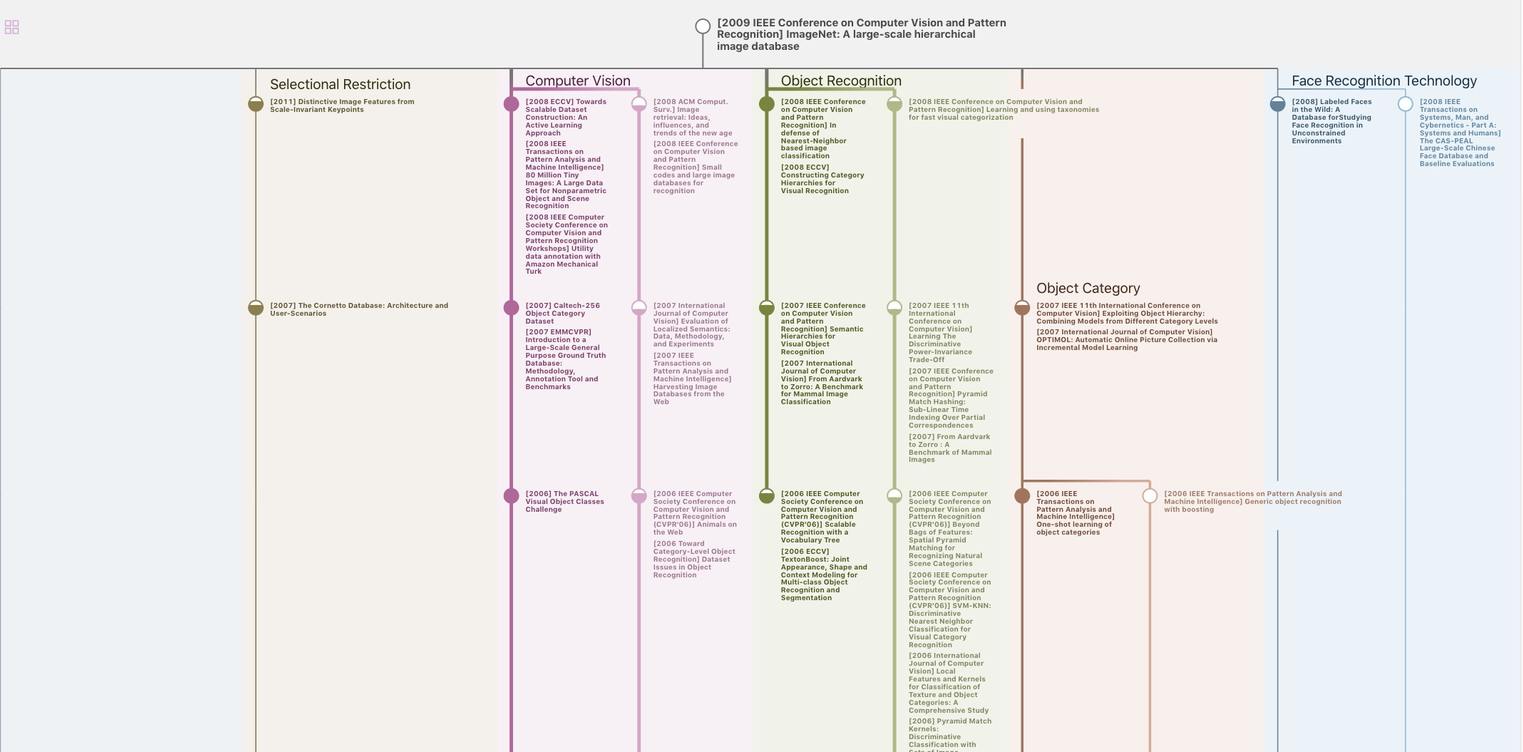
生成溯源树,研究论文发展脉络
Chat Paper
正在生成论文摘要