Power System Fault Classification: An Imbalanced Learning Approach
2021 1st International Conference on Power Electronics and Energy (ICPEE)(2021)
摘要
Critical infrastructures like power systems are inherent to faults for modern grid operation. Operators at the control center must identify them from the frequency as well as voltage measurements available in real time for its proper detection followed by its classification. With the real time high resolution measurements available due to deployment of micro PMUs, faults must be detected in real time with high accuracy. The prime objective of this work lies on a comparative analysis of several machine learning methodologies for proper classification of faults like L-G, LL-G, LLL-G. L-G faults and LLL-G faults generally appear to be the most and least occurred fault scenarios creating an imbalanced datatset, finally promoting a biased classifier. To mitigate this issue this paper applies SMOTE based synthetic data oversampling, finally developing an unbiased machine learning classifier. All the datas for the respective fault scenarios were accumulated from the Drexel University's Reconfigurable Distribution Automation and Control (RDAC) software/hardware laboratory.
更多查看译文
关键词
Fault Classification,Imbalanced Learning,Machine learning,SMOTE
AI 理解论文
溯源树
样例
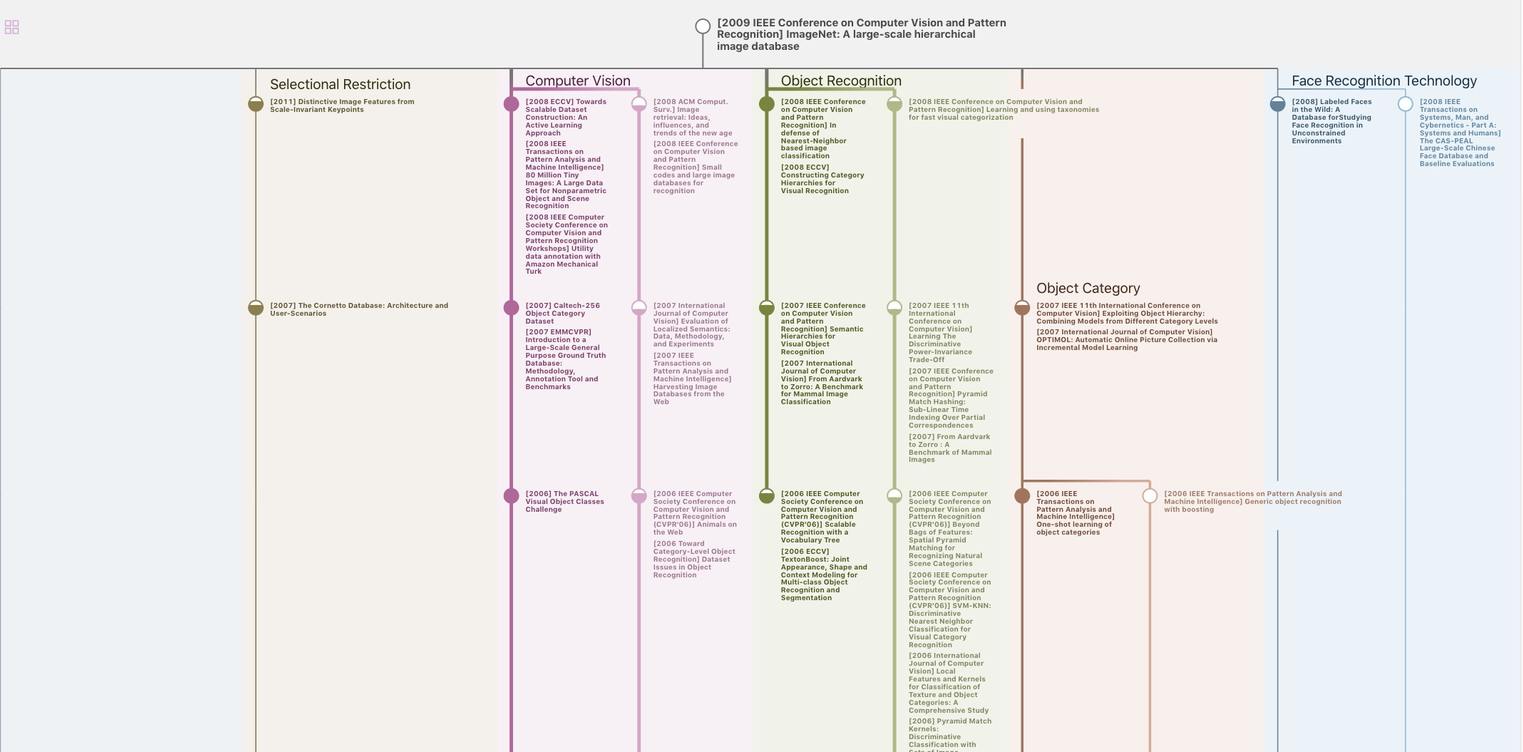
生成溯源树,研究论文发展脉络
Chat Paper
正在生成论文摘要