Total Variability Space for LDA-based multi-viewtext categorization
IEEE Transactions on Audio, Speech, and Language Processing(2015)
摘要
Mapping text document into LDA-based topic-space is a classical way to extract high level representation of text documents. Unfortunatly , LDA is higly sensitive to hyper-parameters related to class number or word and topic distribution , and there is not any systematic way to prior estimate optimal configurations. Morover , various hyperparameter configurations offer complementary views on the document. In this paper , we propose a method based on a two-step process that , first , expands representation space by using a set of topic spaces and , second , compacts representation space by removing poorly relevant dimensions. These two steps are based respectivelly on multi-view LDA-based representation spaces and factor-analysis models. This model provides a view-independant representation of documents while extracting complementary information from a massive multi-view representation. Experiments are conducted on the DECODA conversation corpus and Reuters-21578 textual dataset. Results show the effectiveness of the proposed multi-view compact representation paradigm. The proposed categorization system reaches an accuracy of 86. 9% and 86. 5% respectively with manual and automatic transcriptions of conversations , and a macro-F1 of 80% during a classification task of the well-known studied Reuters-21578 corpus , with a significant gain compared to the baseline (best single topic space configuration) , as well as methods and document representations previously studied .
更多查看译文
AI 理解论文
溯源树
样例
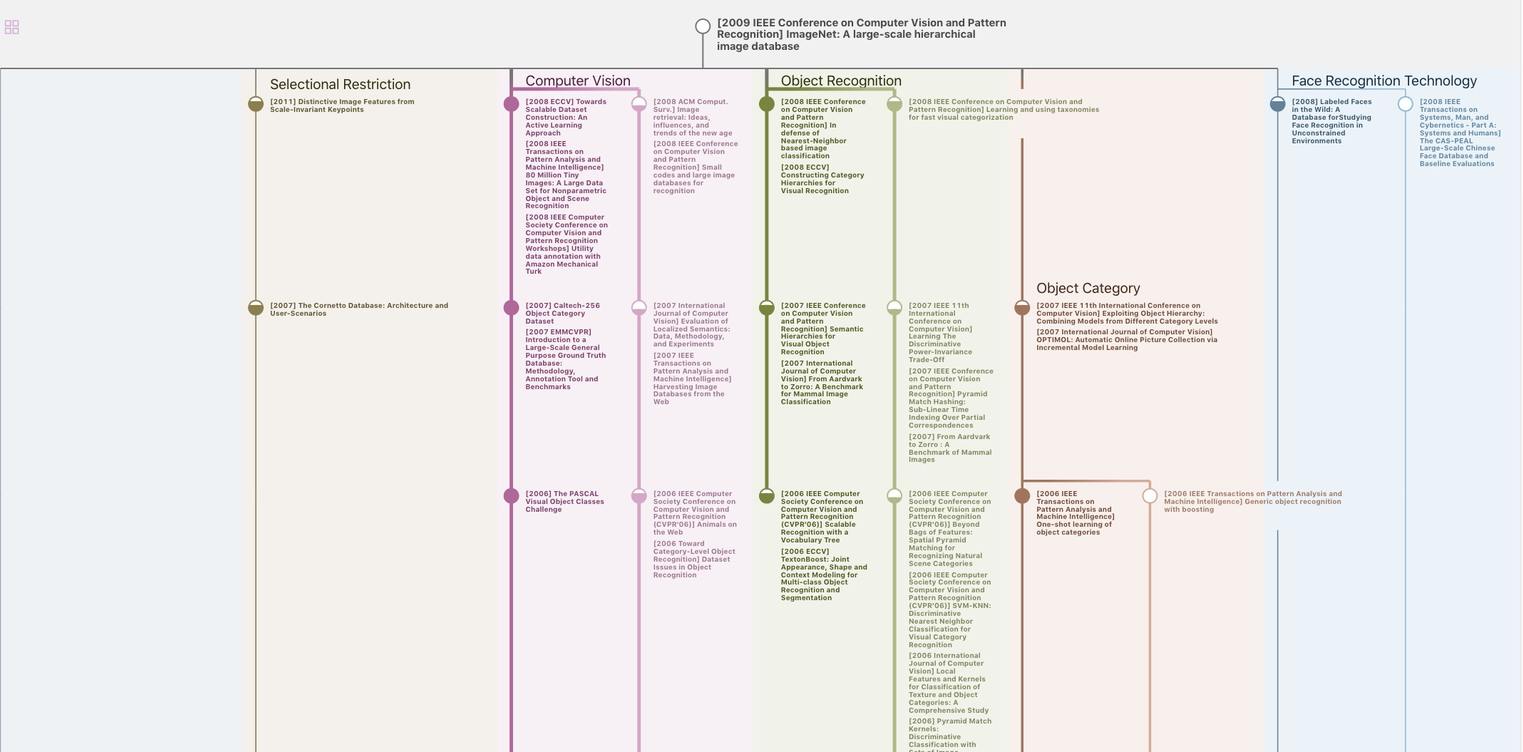
生成溯源树,研究论文发展脉络
Chat Paper
正在生成论文摘要