Modeling Individual Differences through Frequent Pattern Mining on Role-Playing Game Actions
national conference on artificial intelligence(2021)
摘要
There has been much work on player modeling using game behavioral data collected. Many of the previous research projects that targeted this goal used aggregate game statistics as features to develop behavior models using both statistical and machine learning techniques. While existing methods have already led to interesting findings, we suspect that aggregated features discard valuable information such as temporal or sequential patterns, which may be important in deciphering information about decisionmaking, problem solving, or individual differences. Such sequential information is critical to analyze player behaviors especially in role-playing games (RPG) where players can face ample choices, experience different contexts, behave freely with individual propensities but possibly end up with similar aggregated statistics (e.g., levels, time spent). In this paper we intend to develop and apply a modeling technique that takes into consideration sequential patters to decipher individual differences in playing a Role Playing Game (RPG) game. Using an RPG with multiple affordances, we designed an experiment collecting granular in-game behaviors of 64 players. Using closed sequential pattern mining and logistic regression, we developed a model that uses gameplay action sequences to predict the real world characteristics, including gender, game play expertise and five personality traits (as defined by psychology). The results show that game expertise is a dominant factor that impacts in-game behaviors. The contribution of this paper is the algorithms we developed combined with a validation procedure to determine the reliability and validity of the results and the results themselves.
更多查看译文
AI 理解论文
溯源树
样例
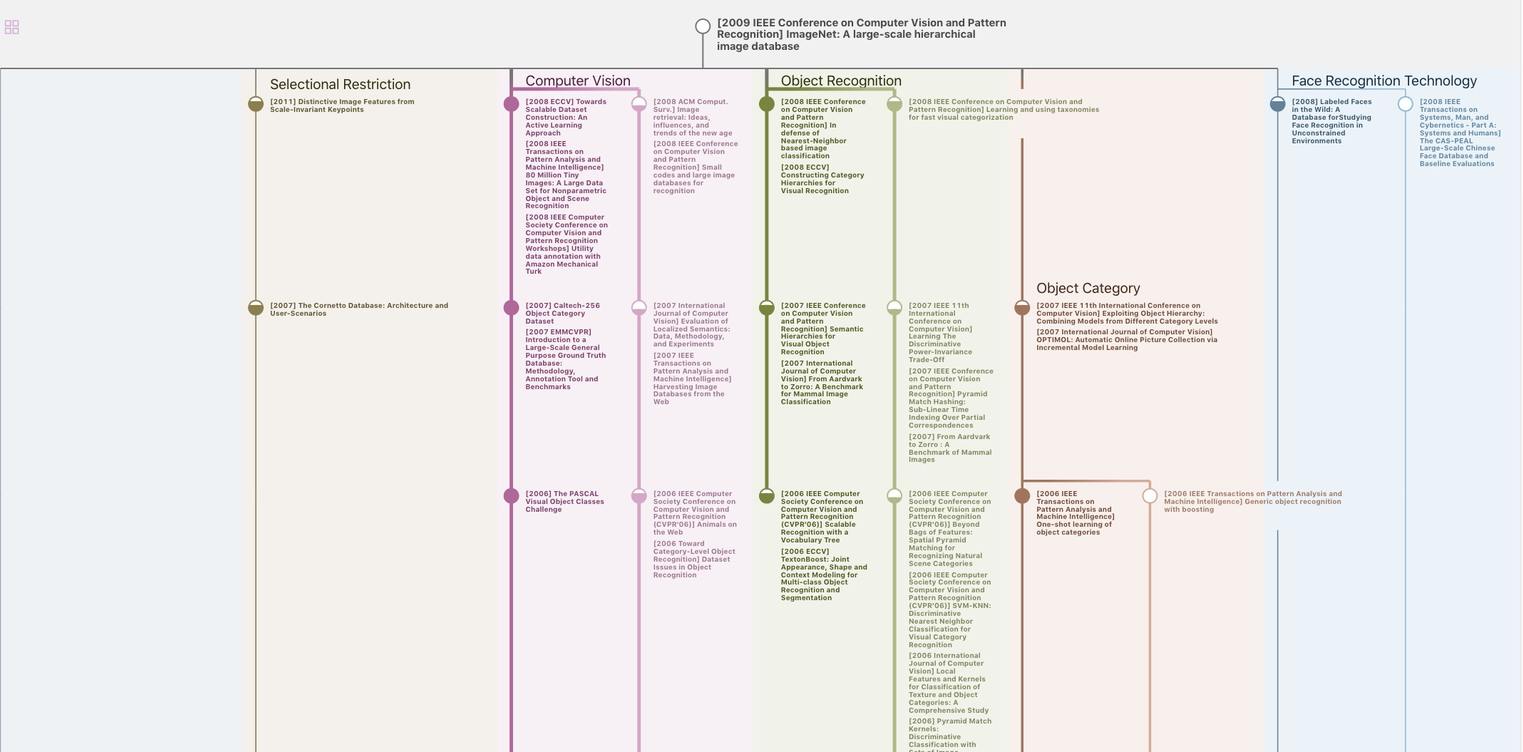
生成溯源树,研究论文发展脉络
Chat Paper
正在生成论文摘要