$$\ell _{1/2,1}$$ ℓ 1 / 2 , 1 group sparse regularization for compressive sensing
Signal, Image and Video Processing(2016)
摘要
Recently, the design of group sparse regularization has drawn much attention in group sparse signal recovery problem. Two of the most popular group sparsity-inducing regularization models are $$\ell _{1,2}$$
and $$\ell _{1,\infty }$$
regularization. Nevertheless, they do not promote the intra-group sparsity. For example, Huang and Zhang (Ann Stat 38:1978–2004, 2010) claimed that the $$\ell _{1,2}$$
regularization is superior to the $$\ell _1$$
regularization only for strongly group sparse signals. This means the sparsity of intra-group is useless for $$\ell _{1,2}$$
regularization. Our experiments show that recovering signals with intra-group sparse needs more measurements than those without, by the $$\ell _{1,\infty }$$
regularization. In this paper, we propose a novel group sparsity-inducing regularization defined as a mixture of the $$\ell _{1/2}$$
norm and the $$\ell _{1}$$
norm, referred to as $$\ell _{1/2,1}$$
regularization, which can overcome these shortcomings of $$\ell _{1,2}$$
and $$\ell _{1,\infty }$$
regularization. We define a new null space property for $$\ell _{1/2,1}$$
regularization and apply it to establish a recoverability theory for both intra-group and inter-group sparse signals. In addition, we introduce an iteratively reweighted algorithm to solve this model and analyze its convergence. Comprehensive experiments on simulated data show that the proposed $$\ell _{1/2,1}$$
regularization is superior to $$\ell _{1,2}$$
and $$\ell _{1,\infty }$$
regularization.
更多查看译文
AI 理解论文
溯源树
样例
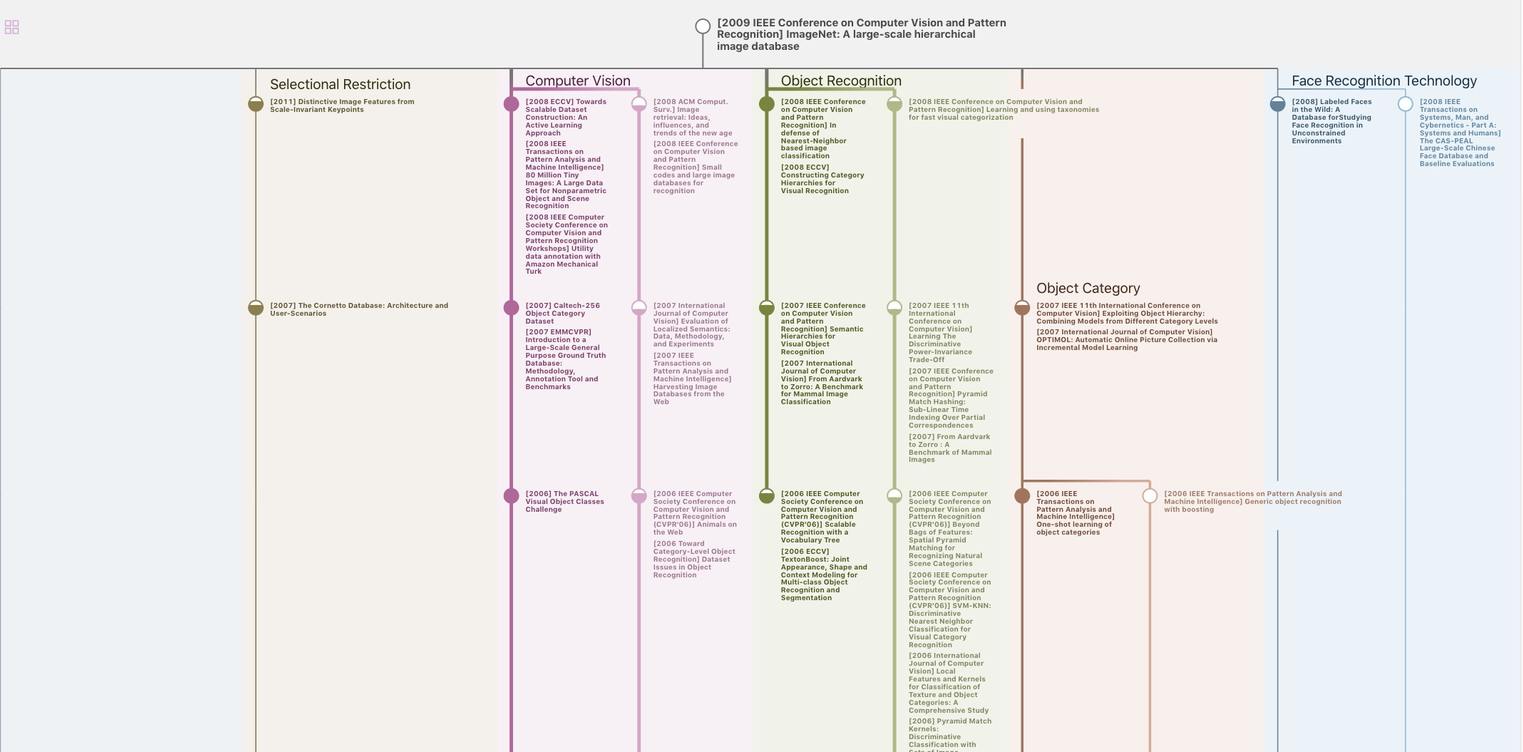
生成溯源树,研究论文发展脉络
Chat Paper
正在生成论文摘要