Automated Diagnosis of Breast Cancer Using Wavelet Based Entropy Features
2018 Second International Conference on Electronics, Communication and Aerospace Technology (ICECA)(2018)
摘要
Breast cancer is one of the most common and serious threat around worldwide for women. It is necessary to prognosis of Histopathology image in order to avoid death rate also early detection is required for the best treatment. Histopathology refers to the examination of biopsy sample by a pathologist under microscope. The pathologist locates, analyze and classify the image type and grade the image. The classification depends on structure of tissue, cell distribution, and shape of the cell which decides the histopathology image as benign or malignant. Manual classification of image is most time consuming and prone to error. To overcome this difficulty automated diagnosis using machine learning is needed for accurate and early diagnosis. In this paper k-means clustering is used for segmentation of cell nuclei and DWT (Discrete Wavelet Transform) is applied to the segmented image. Coiflets is one of the discrete wavelets used in this paper it has scaling functions with vanishing moments. Energy, shannon entropy and log energy entropy features are extracted from the LL, LH, HL and HH sub bands. In the present work we have used BreakHis (The Breast Cancer Histopathological Images) dataset. The proposed method result in a accuracy of 93.3% in linear SVM and 92.7% in Quadratic SVM and 91.3% in Fine Gaussian SVM.
更多查看译文
关键词
Breast cancer,Benign,Malignant,k-means clustering,Coiflets,Entropy,SVM (Support Vector Machine)
AI 理解论文
溯源树
样例
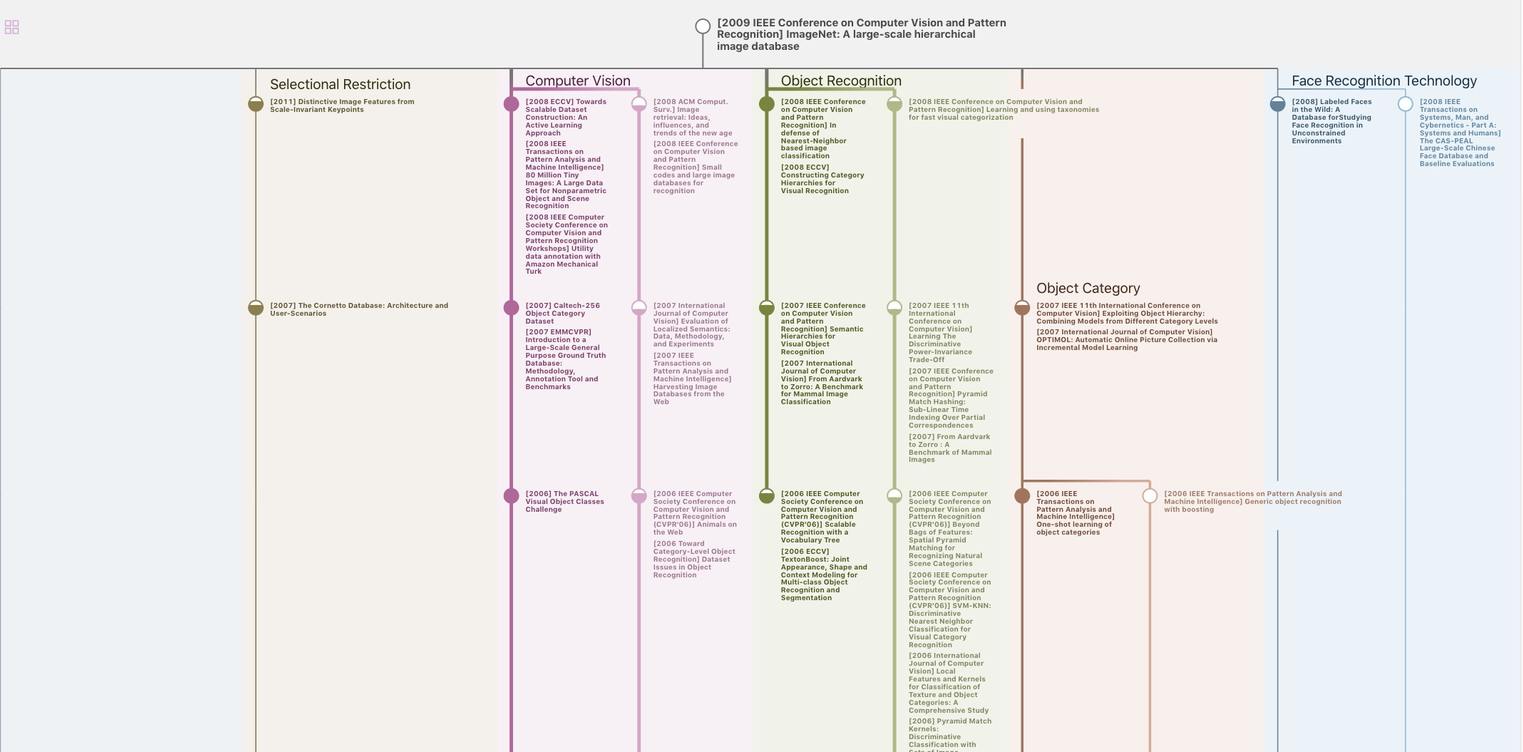
生成溯源树,研究论文发展脉络
Chat Paper
正在生成论文摘要